Leveraging Natural Language Processing for Quality Assurance of a Situational Judgement Test
Artificial Intelligence in Education. Posters and Late Breaking Results, Workshops and Tutorials, Industry and Innovation Tracks, Practitioners’ and Doctoral Consortium(2022)
Abstract
Situational judgement tests (SJTs) measure various non-cognitive skills based on examinees’ actions for hypothetical real-life scenarios. To ensure the validity of scores obtained from SJTs, a quality assurance (QA) framework is essential. In this study, we leverage natural language processing (NLP) to build an efficient and effective QA framework for evaluating scores from an SJT focusing on different aspects of professionalism. Using 635,106 written responses from an operational SJT (Casper), we perform sentiment analysis to analyze if the tone of written responses affects scores assigned by human raters. Furthermore, we implement unsupervised text classification to evaluate the extent to which written responses reflect the theoretical aspects of professionalism underlying the test. Our findings suggest that NLP tools can help us build an efficient and effective QA process to evaluate human scoring and collect validity evidence supporting the inferences drawn from Casper scores.
MoreTranslated text
Key words
Quality assurance,Validity,Situational judgement,Natural language processing
AI Read Science
Must-Reading Tree
Example
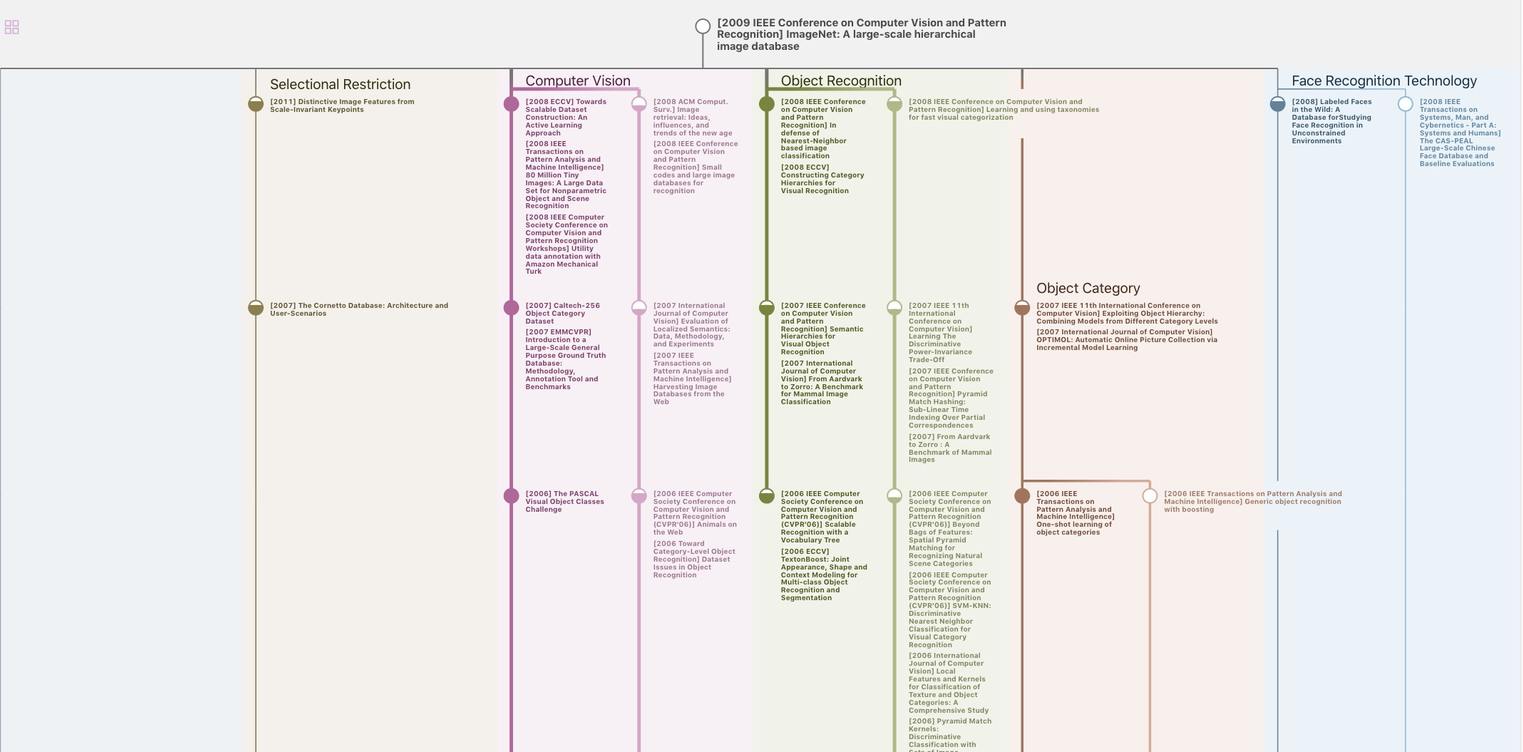
Generate MRT to find the research sequence of this paper
Chat Paper
Summary is being generated by the instructions you defined