When Online Meets Offline: Exploring Periodicity for Travel Destination Prediction
SIGIR '22: Proceedings of the 45th International ACM SIGIR Conference on Research and Development in Information Retrieval(2022)
摘要
Online travel platforms (OTPs), e.g., booking.com and Ctrip.com, deliver travel experiences to online users by providing travel-related products. One key problem facing OTPs is to predict users' future travel destination, which has many important applications, e.g., proactively recommending users flight tickets or hotels in the destination city. Although much progress has been made for the next POI recommendation, they are largely sub-optimal for travel destination prediction on OTPs, due to the unique characteristics exhibited from users' travel behaviors such as offline spatial-temporal periodicity and online multi-interest exploration. In this paper, we propose an online-offline periodicity-aware information gain network, OOPIN, for travel destination prediction on OTPs. The key components of the model are (1) an offline mobility pattern extractor, which extracts spatial-temporal periodicity along with the sequential dependencies from the visited city sequence; and (2) an online multi-interests exploration module that discovers destinations that the user might be interested in but not yet visited from their online interaction data.Comprehensive experiments on real-world OTP demonstrate the superior performance of the proposed model for travel destination prediction compared with state-of-the-art methods.
更多查看译文
关键词
Travel destination prediction, Neural network
AI 理解论文
溯源树
样例
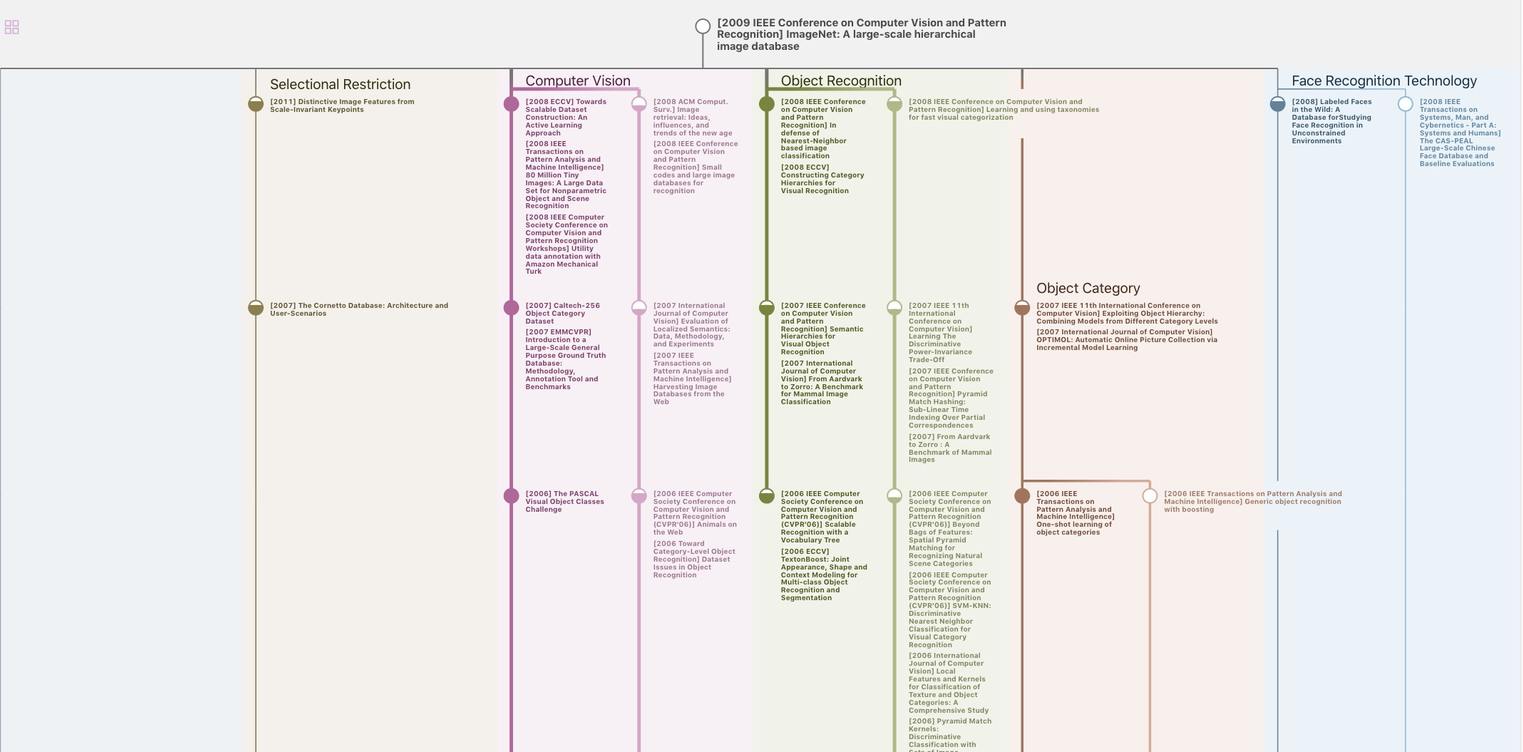
生成溯源树,研究论文发展脉络
Chat Paper
正在生成论文摘要