InPars: Unsupervised Dataset Generation for Information Retrieval
SIGIR '22: Proceedings of the 45th International ACM SIGIR Conference on Research and Development in Information Retrieval(2022)
摘要
The Information Retrieval (IR) community has recently witnessed a revolution due to large pretrained transformer models. Another key ingredient for this revolution was the MS MARCO dataset, whose scale and diversity has enabled zero-shot transfer learning to various tasks. However, not all IR tasks and domains can benefit from one single dataset equally. Extensive research in various NLP tasks has shown that using domain-specific training data, as opposed to a general-purpose one, improves the performance of neural models. In this work, we harness the few-shot capabilities of large pretrained language models as synthetic data generators for IR tasks. We show that models finetuned solely on our synthetic datasets outperform strong baselines such as BM25 as well as recently proposed self-supervised dense retrieval methods. Code, models, and data are available at https://github.com/zetaalphavector/inpars.
更多查看译文
关键词
Few-shot Models, Large Language Models, Generative Models, Question Generation, Synthetic Datasets, Multi-stage Ranking
AI 理解论文
溯源树
样例
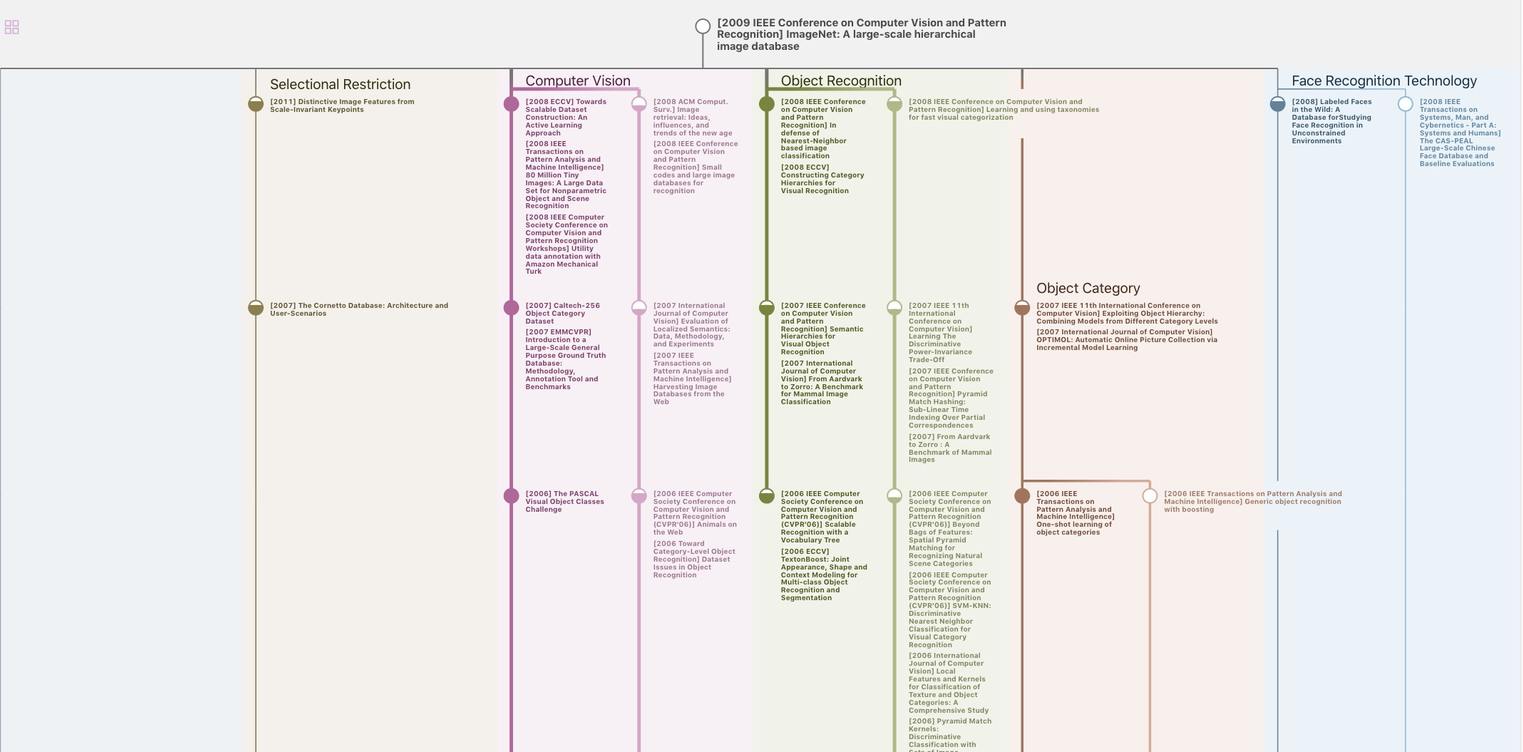
生成溯源树,研究论文发展脉络
Chat Paper
正在生成论文摘要