DeSCoVeR: Debiased Semantic Context Prior for Venue Recommendation
SIGIR '22: Proceedings of the 45th International ACM SIGIR Conference on Research and Development in Information Retrieval(2022)
摘要
We present a novel semantic context prior-based venue recommendation system that uses only the title and the abstract of a paper. Based on the intuition that the text in the title and abstract have both semantic and syntactic components, we demonstrate that a joint training of a semantic feature extractor and syntactic feature extractor collaboratively leverages meaningful information that helps to provide venues for papers. The proposed methodology that we call DeSCoVeR at first elicits these semantic and syntactic features using a Neural Topic Model and text classifier respectively. The model then executes a transfer learning optimization procedure to perform a contextual transfer between the feature distributions of the Neural Topic Model and the text classifier during the training phase. DeSCoVeR also mitigates the document-level label bias using a Causal back-door path criterion and a sentence-level keyword bias removal technique. Experiments on the DBLP dataset show that DeSCoVeR outperforms the state-of-the-art methods.
更多查看译文
关键词
Document Classification, Topic Modeling, Joint Learning, Mutual Transfer, Causal Debiasing
AI 理解论文
溯源树
样例
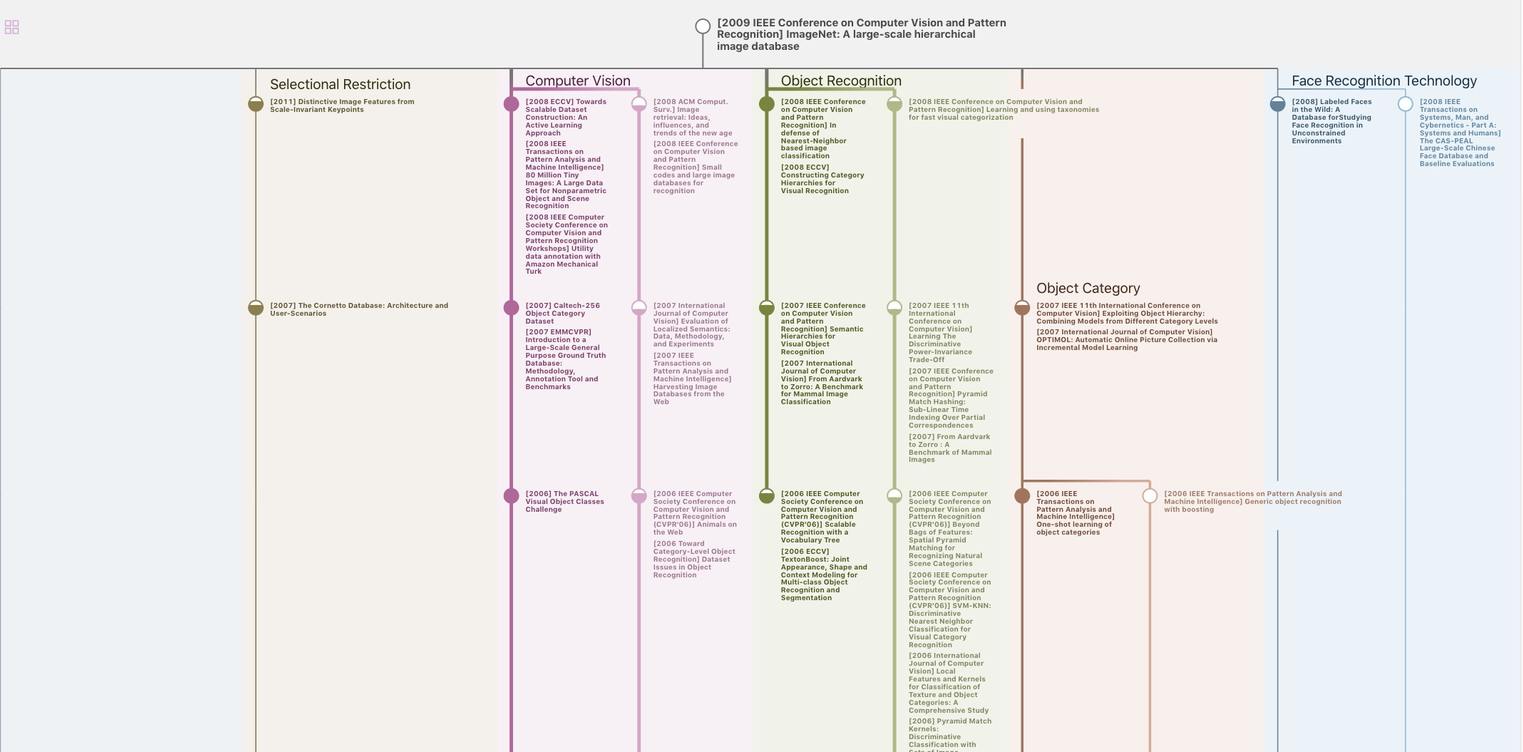
生成溯源树,研究论文发展脉络
Chat Paper
正在生成论文摘要