Webformer: Pre-training with Web Pages for Information Retrieval
SIGIR '22: Proceedings of the 45th International ACM SIGIR Conference on Research and Development in Information Retrieval(2022)
摘要
Pre-trained language models (PLMs) have achieved great success in the area of Information Retrieval. Studies show that applying these models to ad-hoc document ranking can achieve better retrieval effectiveness. However, on the Web, most information is organized in the form of HTML web pages. In addition to the pure text content, the structure of the content organized by HTML tags is also an important part of the information delivered on a web page. Currently, such structured information is totally ignored by pre-trained models which are trained solely based on text content. In this paper, we propose to leverage large-scale web pages and their DOM (Document Object Model) tree structures to pre-train models for information retrieval. We argue that using the hierarchical structure contained in web pages, we can get richer contextual information for training better language models. To exploit this kind of information, we devise four pre-training objectives based on the structure of web pages, then pre-train a Transformer model towards these tasks jointly with traditional masked language model objective. Experimental results on two authoritative ad-hoc retrieval datasets prove that our model can significantly improve ranking performance compared to existing pre-trained models.
更多查看译文
关键词
Ad-hoc Retrieval, Pre-training, Web Page, DOM Tree
AI 理解论文
溯源树
样例
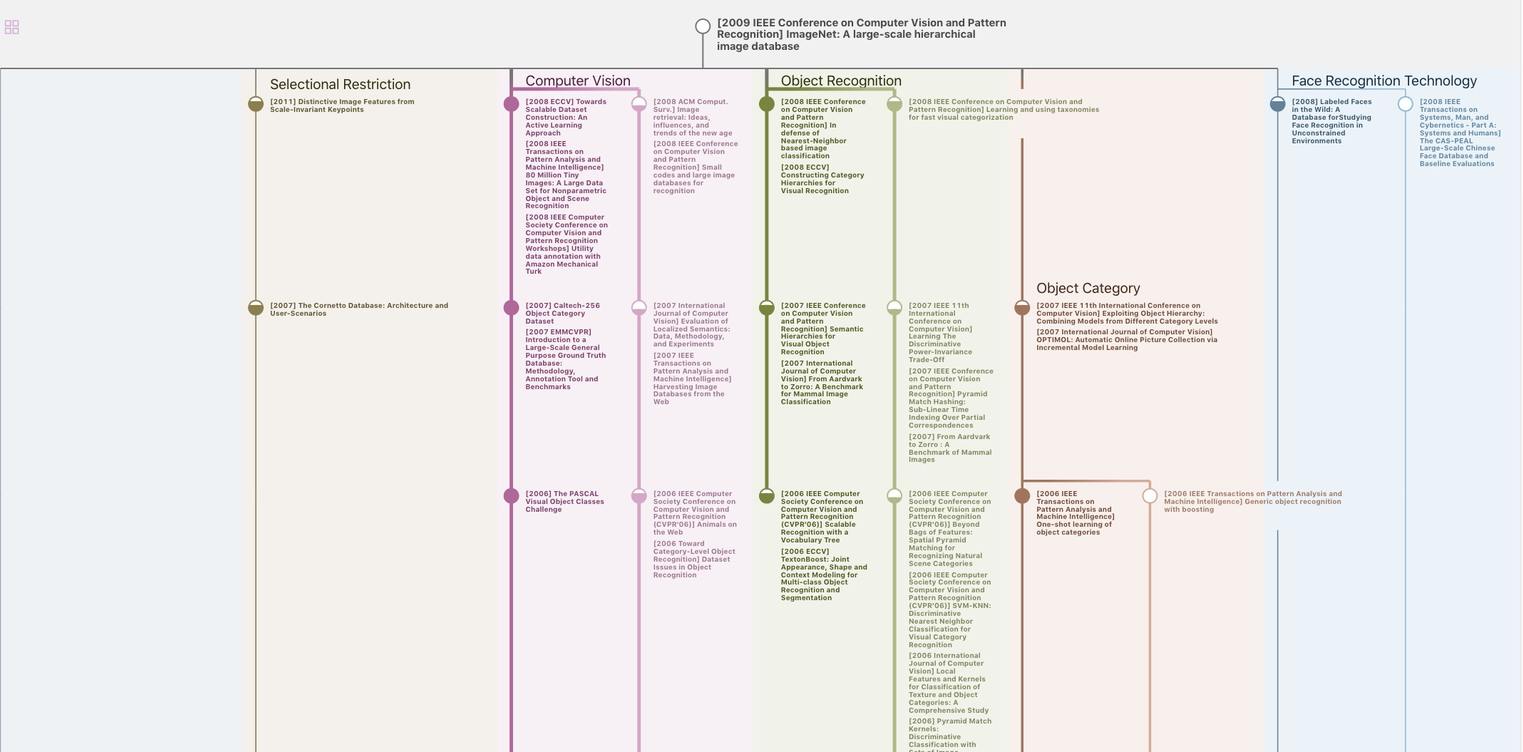
生成溯源树,研究论文发展脉络
Chat Paper
正在生成论文摘要