KETCH: Knowledge Graph Enhanced Thread Recommendation in Healthcare Forums
SIGIR '22: Proceedings of the 45th International ACM SIGIR Conference on Research and Development in Information Retrieval(2022)
摘要
Health thread recommendation methods aim to suggest the most relevant existing threads for a user. Most of the existing methods tend to rely on modeling the post contents to retrieve relevant answers. However, some posts written by users with different clinical conditions can be lexically similar, as unrelated diseases (e.g., Angina and Osteoporosis) may have the same symptoms (e.g., back pain), yet irrelevant threads to a user. Therefore, it is critical to not only consider the connections between users and threads, but also the descriptions of users' symptoms and clinical conditions. In this paper, towards this problem of thread recommendation in online healthcare forums, we propose a knowledge graph enhanced Threads Recommendation (KETCH) model, which leverages graph neural networks to model the interactions among users and threads, and learn their representations. In our model, the users, threads and posts are three types of nodes in a graph, linked through their associations. KETCH uses the message passing strategy by aggregating information along with the network. In addition, we introduce a knowledge-enhanced attention mechanism to capture the latent conditions and symptoms. We also apply the method to the task of predicting the side effects of drugs, to show that KETCH has the potential to complement the medical knowledge graph. Comparing with the best results of seven competing methods, in terms of MRR, KETCH outperforms all methods by at least 0.125 on the MedHelp dataset, 0.048 on the Patient dataset and 0.092 on HealthBoards dataset, respectively. We release the source code of KETCH at: https://github.com/cuilimeng/KETCH.
更多查看译文
关键词
Recommendation system, online health forum, graph neural networks, medical knowledge graph
AI 理解论文
溯源树
样例
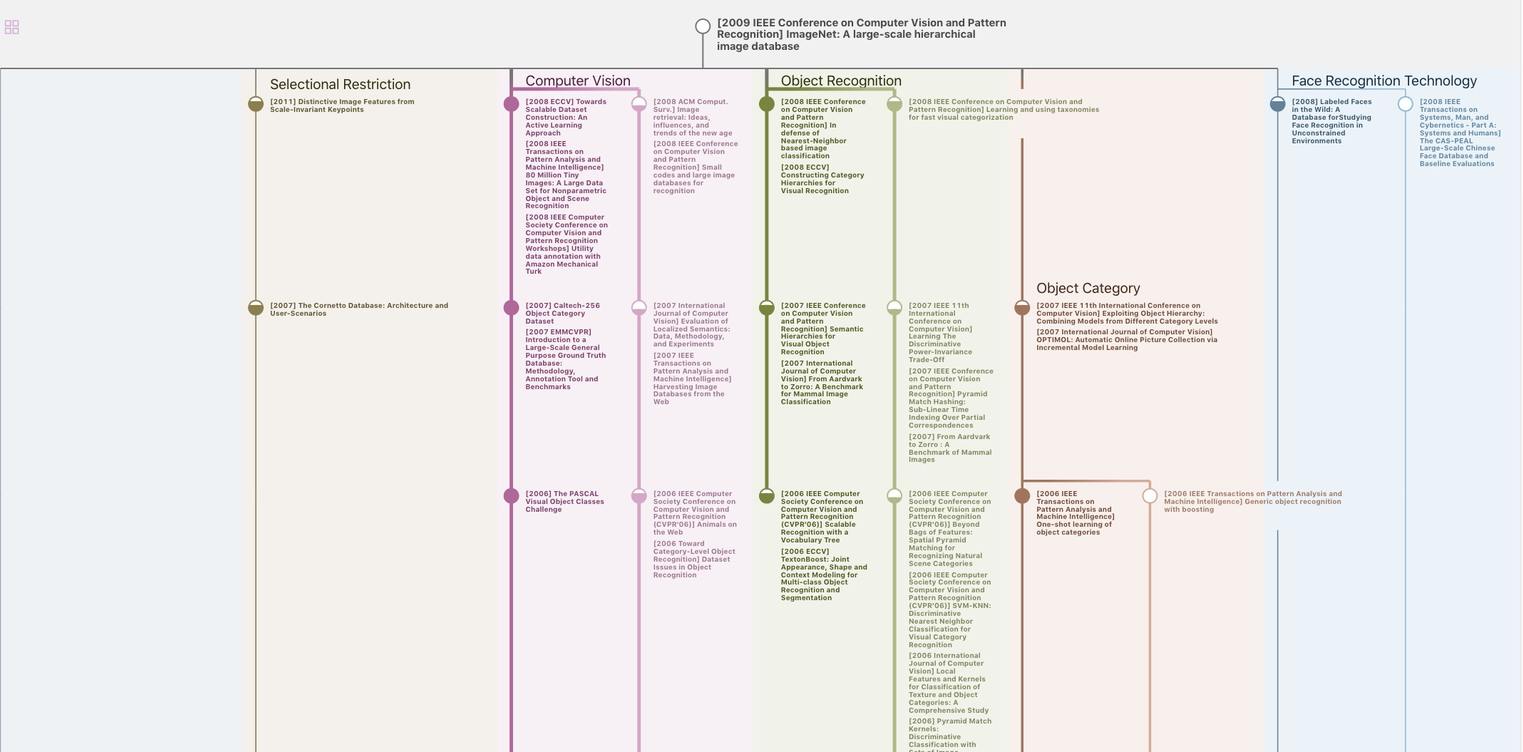
生成溯源树,研究论文发展脉络
Chat Paper
正在生成论文摘要