Learning to Infer User Implicit Preference in Conversational Recommendation
SIGIR '22: Proceedings of the 45th International ACM SIGIR Conference on Research and Development in Information Retrieval(2022)
摘要
Conversational recommender systems (CRS) enable traditional recommender systems to interact with users by asking questions about attributes and recommending items. The attribute-level and item-level feedback of users can be utilized to estimate users' preferences. However, existing works do not fully exploit the advantage of explicit item feedback --- they only use the item feedback in rather implicit ways such as updating the latent user and item representation. Since CRS has multiple chances to interact with users, leveraging the context in the conversation may help infer users' implicit feedback (e.g., some specific attributes) when recommendations get rejected. To address the limitations of existing methods, we propose a new CRS framework called Conversational Recommender with Implicit Feedback (CRIF). CRIF formulates the conversational recommendation scheme as a four-phase process consisting of offline representation learning, tracking, decision, and inference. In the inference module, by fully utilizing the relation between users' attribute-level and item-level feedback, our method can explicitly deduce users' implicit preferences. Therefore, CRIF is able to achieve more accurate user preference estimation. Besides, in the decision module, to better utilize the attribute-level and item-level feedback, we adopt inverse reinforcement learning to learn a flexible decision strategy that selects the suitable action at each conversation turn. Through extensive experiments on four benchmark CRS datasets, we validate the effectiveness of our approach, which significantly outperforms the state-of-the-art CRS methods.
更多查看译文
关键词
Conversational Recommendation, User Preference Inference, Inverse Reinforcement Learning
AI 理解论文
溯源树
样例
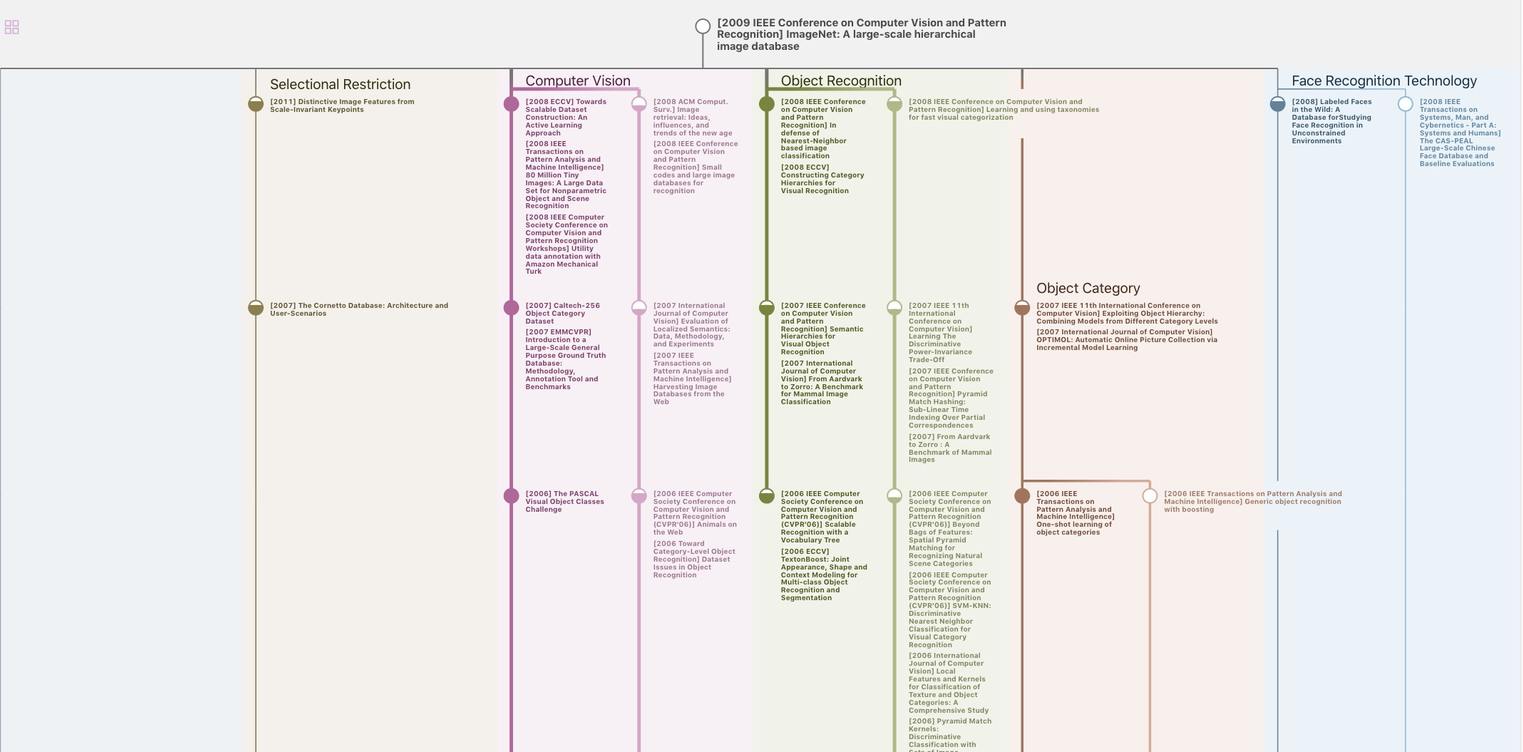
生成溯源树,研究论文发展脉络
Chat Paper
正在生成论文摘要