Learning to Enrich Query Representation with Pseudo-Relevance Feedback for Cross-lingual Retrieval
SIGIR '22: Proceedings of the 45th International ACM SIGIR Conference on Research and Development in Information Retrieval(2022)
摘要
Cross-lingual information retrieval (CLIR) aims to provide access to information across languages. Recent pre-trained multilingual language models brought large improvements to the natural language tasks, including cross-lingual adhoc retrieval. However, pseudo-relevance feedback (PRF), a family of techniques for improving ranking using the contents of top initially retrieved items, has not been explored with neural CLIR retrieval models. Two of the challenges are incorporating feedback from long documents, and cross-language knowledge transfer. To address these challenges, we propose a novel neural CLIR architecture, NCLPRF, capable of incorporating PRF feedback from multiple potentially long documents, which enables improvements to query representation in the shared semantic space between query and document languages. The additional information that the feedback documents provide in a target language, can enrich the query representation, bringing it closer to relevant documents in the embedding space. The proposed model performance across three CLIR test collections in Chinese, Russian, and Persian languages, exhibits significant improvements over traditional and SOTA neural CLIR baselines across all three collections.
更多查看译文
关键词
cross-lingual information retrieval, pseudo-relevance feedback, dense retrieval, language modeling, contextualization, reciprocal rank weighting
AI 理解论文
溯源树
样例
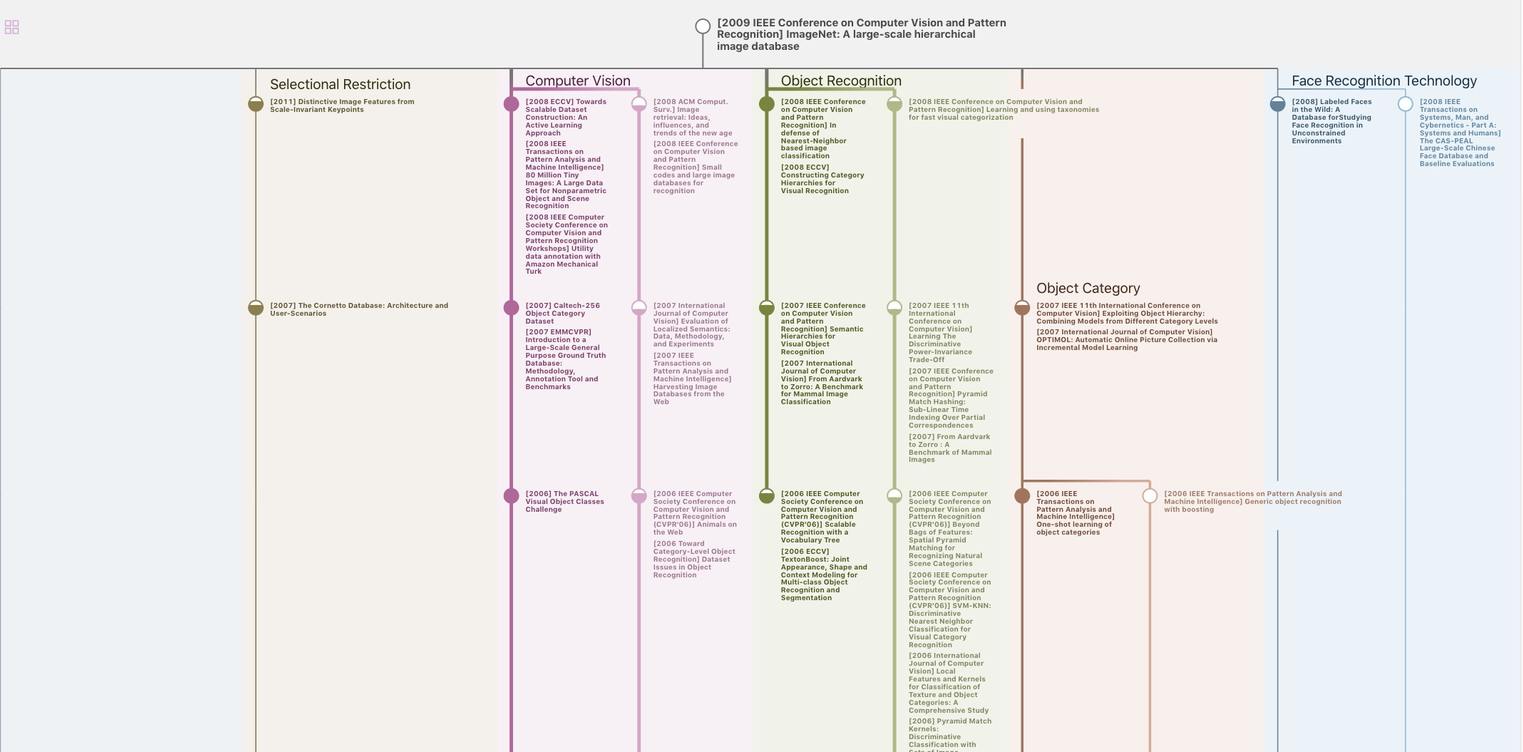
生成溯源树,研究论文发展脉络
Chat Paper
正在生成论文摘要