Large-Scale Patch-Wise Pathological Image Feature Dataset with a Hardware-agnostic Feature Extraction Tool
MEDICAL IMAGE UNDERSTANDING AND ANALYSIS, MIUA 2022(2022)
摘要
Recent advances in whole slide imaging (WSI) have transformed computer-aided pathological studies from small-scale (e.g., <500 patients) to large-scale (e.g., >10,000 patients). Moreover, a single whole slide image might yield Gigapixel resolution; thus, even basic preprocessing steps, such as foreground segmentation, tiling, and patch-wise feature extraction (e.g., via ImageNet pretrained models), can be computationally expensive. For example, it would take 2,400 h to simply obtain patch-level low-dimensional features (e.g., 1D feature with 2048 dimension) from all foreground patches (e.g., 512x512 images) in 10,000 WSI images. In this paper, we present a large-scale patch-wise pathological image feature dataset, covering 14,000 WSIs from TCGA and PAIP cohorts. The contribution of this study is five-fold: (1) We release a foreground patch-level feature dataset, saving 92.1% of storage space and 140 days of computational time; (2) The global spatial location of the patch-level features is provided to aggregate WSI-level results; (3) The feature dataset from two pretrained models (ImageNet and BiT) and two resolutions (1024 and 2048) are evaluated and released for flexible downstream analyses; (4) We containerize the foreground segmentation, tiling, and feature extraction steps as an operating system and hardware agnostic Docker toolkit, called PathContainer, to allow for convenient feature extraction; (5) The entire PathFeature dataset and the PathContainer software have been made publicly available. When performing a standard weakly supervised segmentation method on 940 WSIs, 85.3% of computational time was saved using the PathFeature dataset. The code and data have been made publicly available at https://github.com/hrlblab/PathContainer.
更多查看译文
关键词
Computational pathology,Feature extraction,Weakly supervised learning
AI 理解论文
溯源树
样例
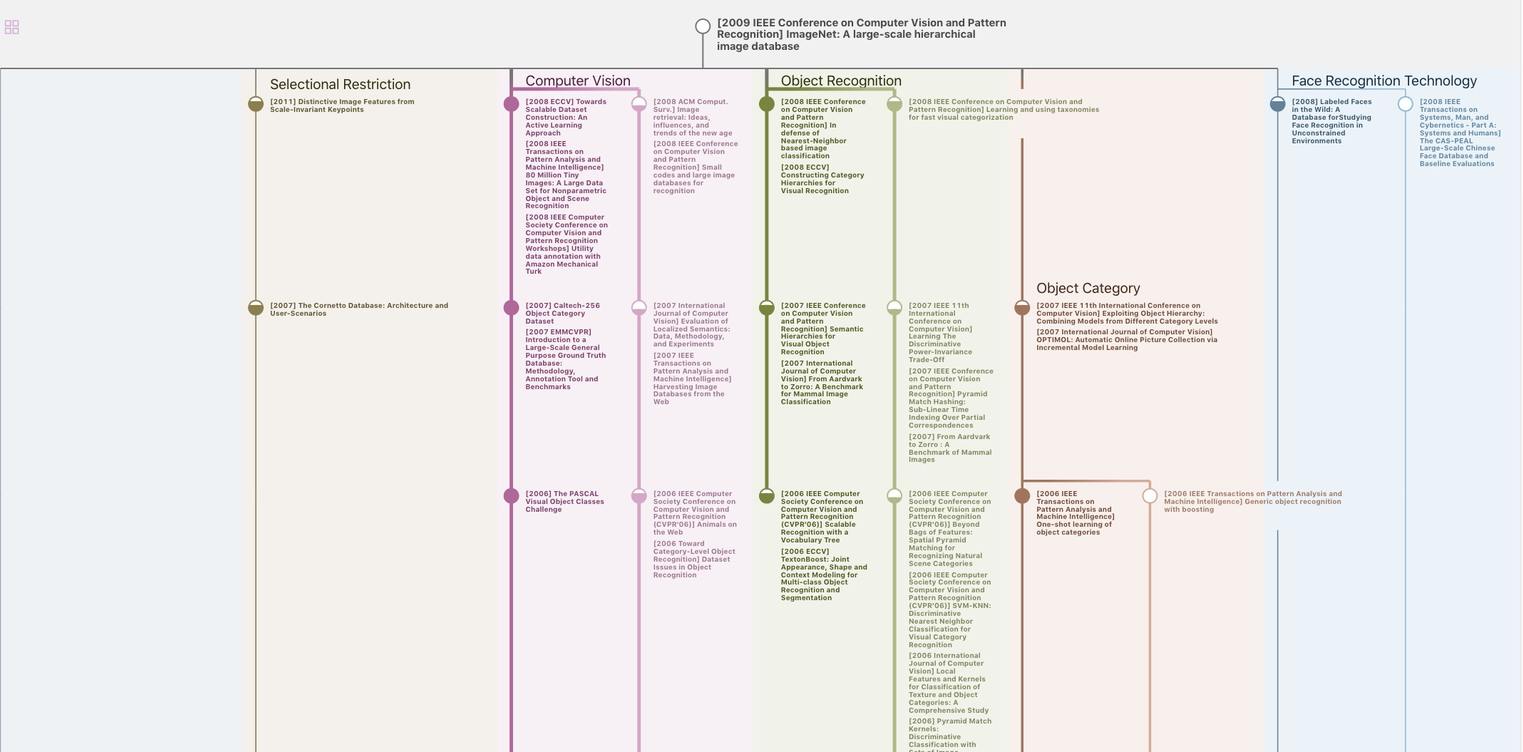
生成溯源树,研究论文发展脉络
Chat Paper
正在生成论文摘要