High Dimensional Stochastic Linear Contextual Bandit with Missing Covariates
2022 IEEE 32nd International Workshop on Machine Learning for Signal Processing (MLSP)(2022)
摘要
Recent works in bandit problems adopted lasso convergence theory in the sequential decision-making setting. Even with fully observed contexts, there are technical challenges that hinder the application of existing lasso convergence theory: 1) proving the restricted eigenvalue condition under conditionally sub-Gaussian noise and 2) accounting for the dependence between the context variables and the chosen actions. This paper studies the effect of missing covariates on regret for stochastic linear bandit algorithms. Our work provides a high-probability upper bound on the regret incurred by the proposed algorithm in terms of covariate sampling probabilities, showing that the regret degrades due to missingness by at most
$\zeta_{min}^{2}$
, where
$\zeta_{min}$
is the minimum probability of observing covariates in the context vector. We illustrate our algorithm for the practical application of experimental design for collecting gene expression data by a sequential selection of class discriminating DNA probes.
更多查看译文
关键词
Contextual bandit,missing values,regret analysis
AI 理解论文
溯源树
样例
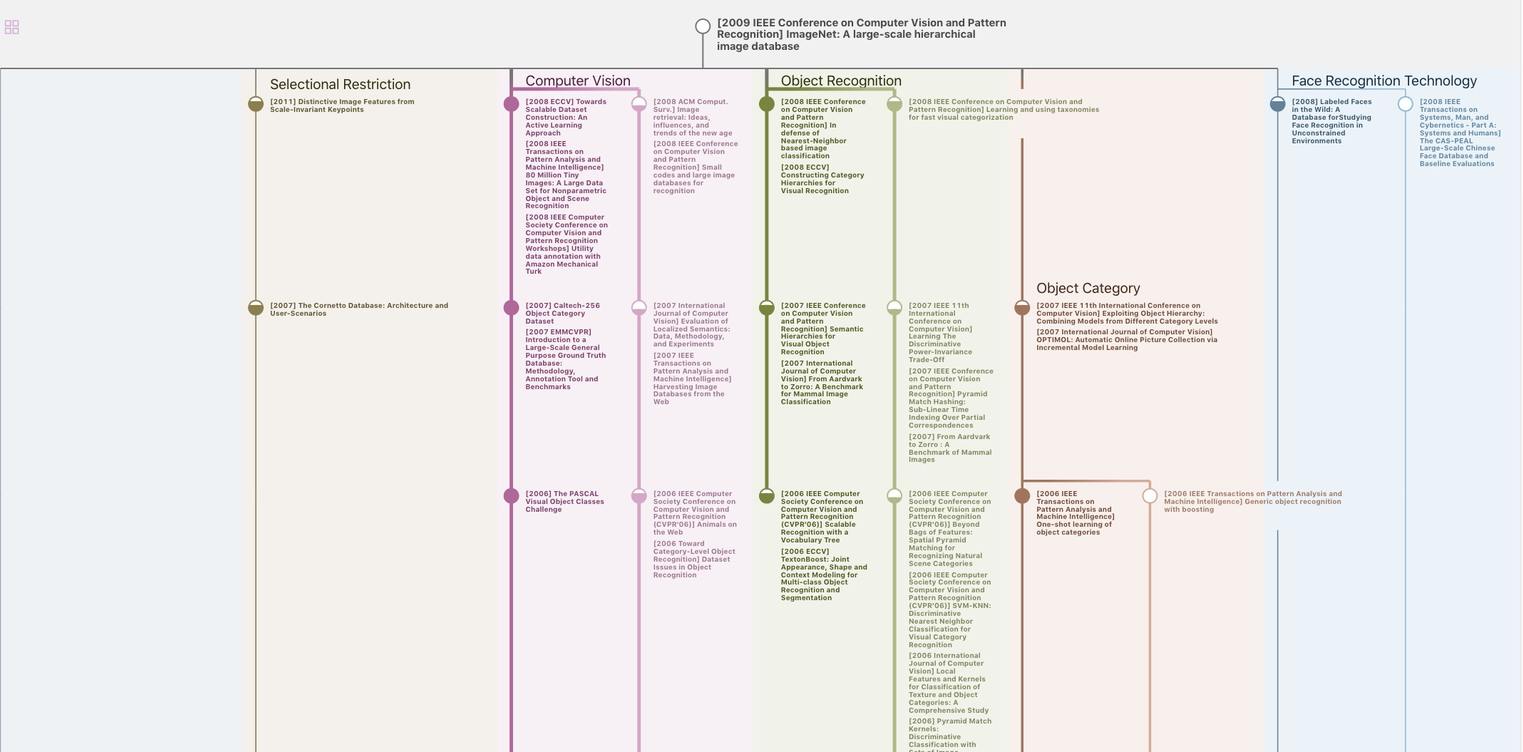
生成溯源树,研究论文发展脉络
Chat Paper
正在生成论文摘要