Context-aware controller inference for stabilizing dynamical systems from scarce data
Proceedings of the Royal Society A Mathematical, Physical and Engineering Sciences(2023)
摘要
This work introduces a data-driven control approach for stabilizing high-dimensional dynamical systems from scarce data. The proposed context-aware controller inference approach is based on the observation that controllers need to act locally only on the unstable dynamics to stabilize systems. This means it is sufficient to learn the unstable dynamics alone, which are typically confined to much lower dimensional spaces than the high-dimensional state spaces of all system dynamics and thus few data samples are sufficient to identify them. Numerical experiments demonstrate that context-aware controller inference learns stabilizing controllers from orders of magnitude fewer data samples than traditional data-driven control techniques and variants of reinforcement learning. The experiments further show that the low data requirements of context-aware controller inference are especially beneficial in data-scarce engineering problems with complex physics, for which learning complete system dynamics is often intractable in terms of data and training costs.
更多查看译文
关键词
nonlinear systems,stabilizing feedback,data-driven control,context-aware learning
AI 理解论文
溯源树
样例
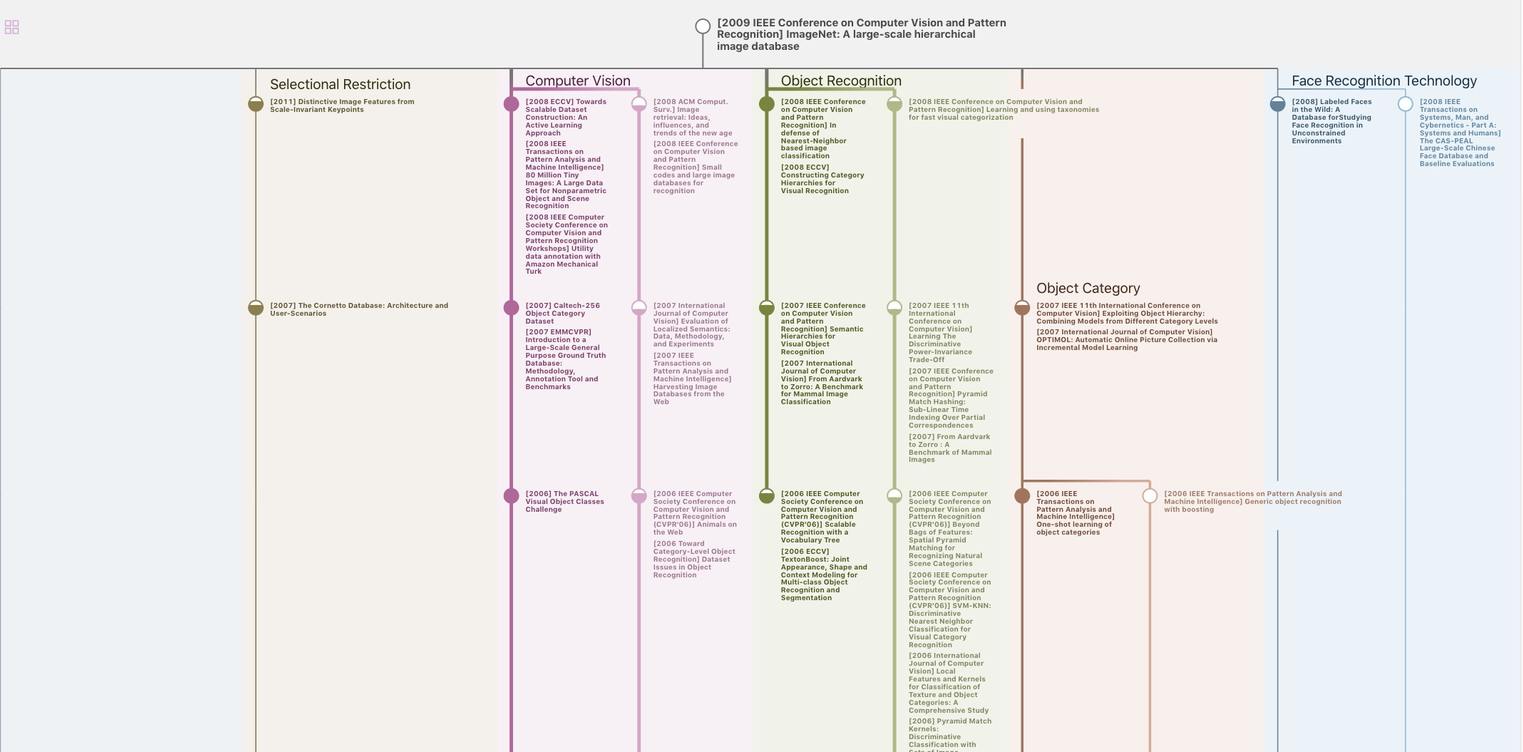
生成溯源树,研究论文发展脉络
Chat Paper
正在生成论文摘要