Rapid Lung Ultrasound COVID-19 Severity Scoring with Resource-Efficient Deep Feature Extraction
SIMPLIFYING MEDICAL ULTRASOUND, ASMUS 2022(2022)
摘要
Artificial intelligence-based analysis of lung ultrasound imaging has been demonstrated as an effective technique for rapid diagnostic decision support throughout the COVID-19 pandemic. However, such techniques can require days- or weeks-long training processes and hyper-parameter tuning to develop intelligent deep learning image analysis models. This work focuses on leveraging 'off-the-shelf pre-trained models as deep feature extractors for scoring disease severity with minimal training time. We propose using pre-trained initializations of existing methods ahead of simple and compact neural networks to reduce reliance on computational capacity. This reduction of computational capacity is of critical importance in time-limited or resource-constrained circumstances, such as the early stages of a pandemic. On a dataset of 49 patients, comprising over 20,000 images, we demonstrate that the use of existing methods as feature extractors results in the effective classification of COVID-19-related pneumonia severity while requiring only minutes of training time. Our methods can achieve an accuracy of over 0.93 on a 4-level severity score scale and provides comparable per-patient region and global scores compared to expert annotated ground truths. These results demonstrate the capability for rapid deployment and use of such minimally-adapted methods for progress monitoring, patient stratification and management in clinical practice for COVID-19 patients, and potentially in other respiratory diseases.
更多查看译文
关键词
Severity scoring, Deep learning, Lung ultrasound, COVID-19
AI 理解论文
溯源树
样例
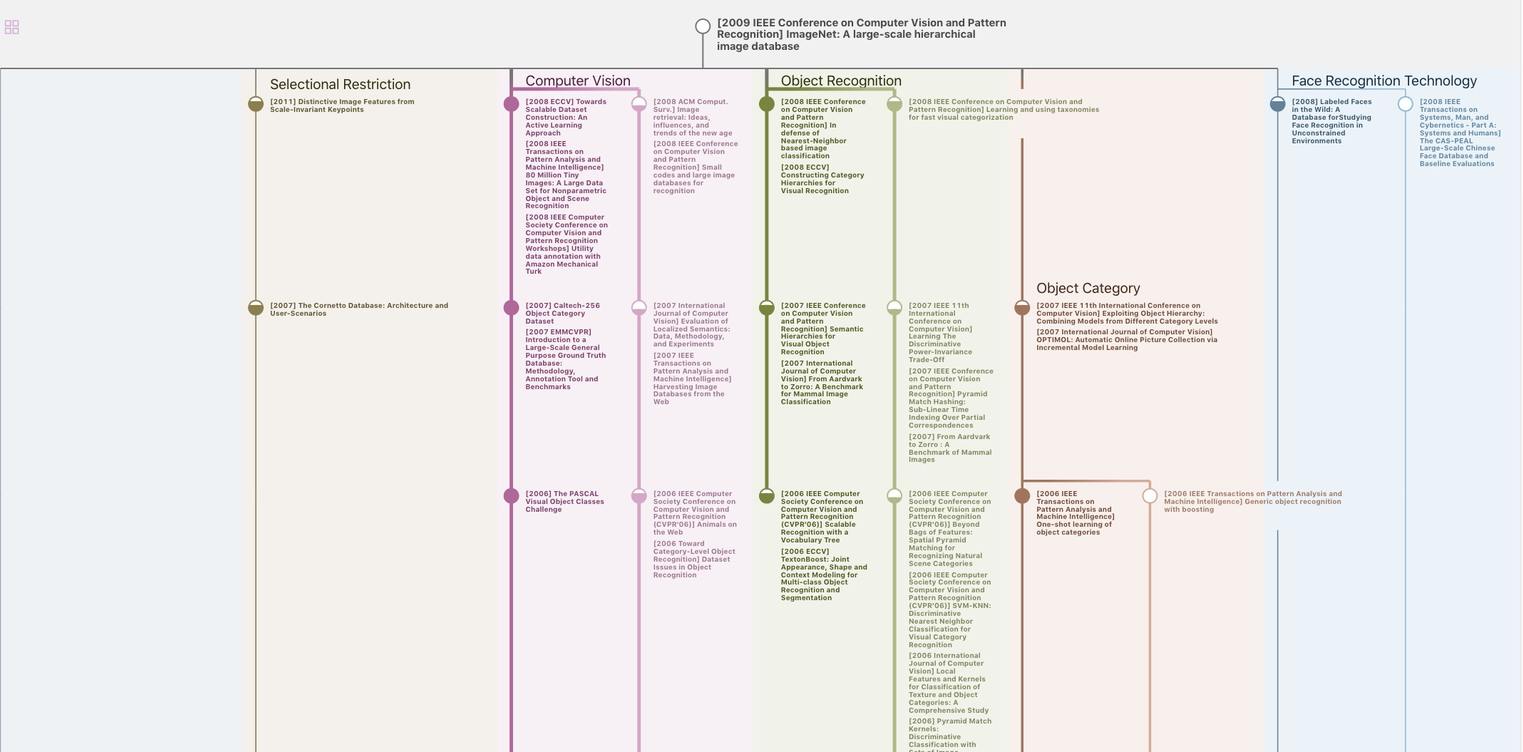
生成溯源树,研究论文发展脉络
Chat Paper
正在生成论文摘要