Ground state search by local and sequential updates of neural network quantum states
arxiv(2022)
摘要
Neural network quantum states are a promising tool to analyze complex quantum systems given their representative power. It can however be difficult to optimize efficiently and effectively the parameters of this type of ansatz. Here we propose a local optimization procedure which, when integrated with stochastic reconfiguration, outperforms previously used global optimization approaches. Specifically, we analyze both the ground state energy and the correlations for the non-integrable tilted Ising model with restricted Boltzmann machines. We find that sequential local updates can lead to faster convergence to states which have energy and correlations closer to those of the ground state, depending on the size of the portion of the neural network which is locally updated. To show the generality of the approach we apply it to both 1D and 2D non-integrable spin systems.
更多查看译文
关键词
neural network quantum states,ground state search,ground state,neural network
AI 理解论文
溯源树
样例
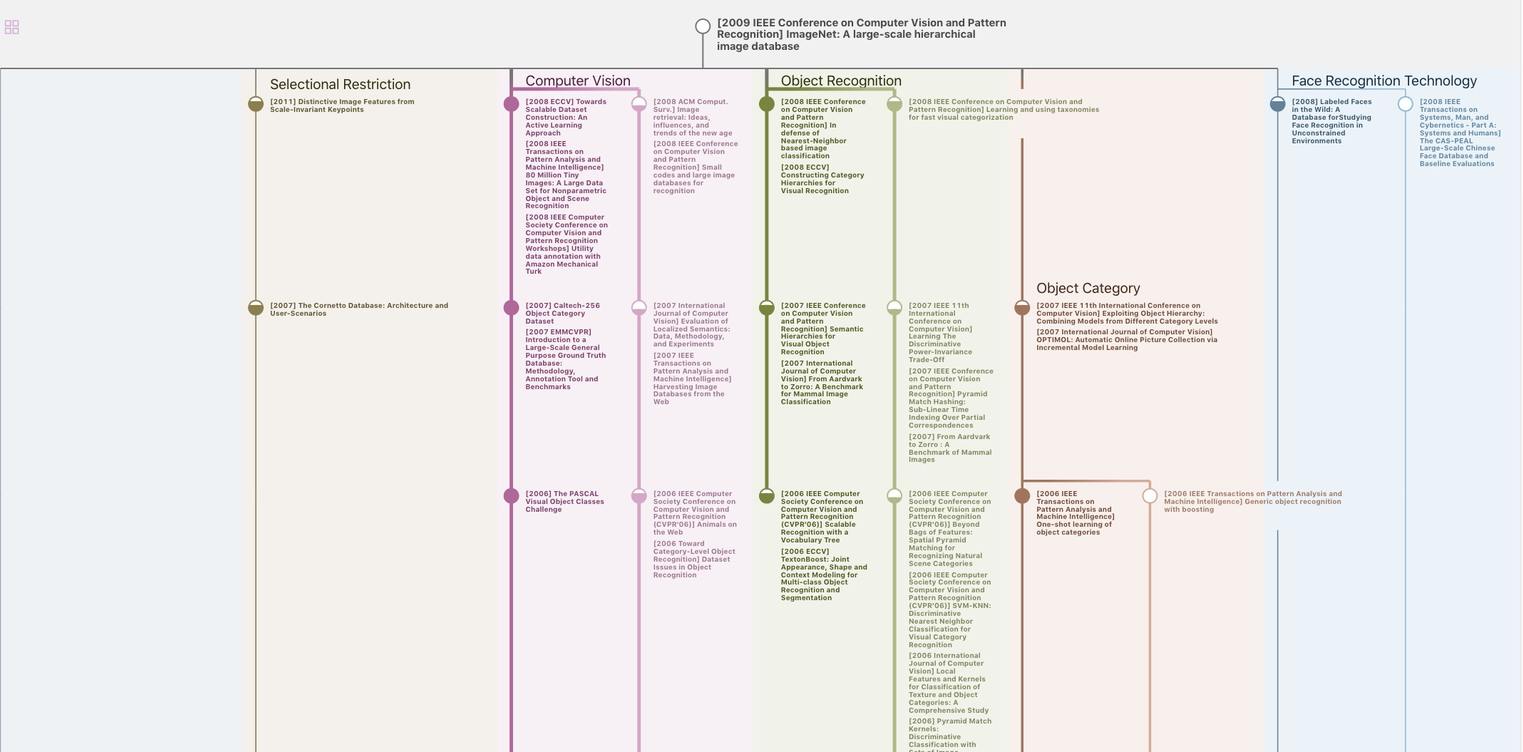
生成溯源树,研究论文发展脉络
Chat Paper
正在生成论文摘要