DEVIANT: Depth EquiVarIAnt NeTwork for Monocular 3D Object Detection.
European Conference on Computer Vision(2022)
摘要
Modern neural networks use building blocks such as convolutions that are equivariant to arbitrary \(2\)D translations. However, these vanilla blocks are not equivariant to arbitrary \(3\)D translations in the projective manifold. Even then, all monocular \(3\)D detectors use vanilla blocks to obtain the \(3\)D coordinates, a task for which the vanilla blocks are not designed for. This paper takes the first step towards convolutions equivariant to arbitrary \(3\)D translations in the projective manifold. Since the depth is the hardest to estimate for monocular detection, this paper proposes Depth EquiVarIAnt NeTwork (DEVIANT) built with existing scale equivariant steerable blocks. As a result, DEVIANT is equivariant to the depth translations in the projective manifold whereas vanilla networks are not. The additional depth equivariance forces the DEVIANT to learn consistent depth estimates, and therefore, DEVIANT achieves state-of-the-art monocular \(3\)D detection results on KITTI and Waymo datasets in the image-only category and performs competitively to methods using extra information. Moreover, DEVIANT works better than vanilla networks in cross-dataset evaluation.
更多查看译文
关键词
Equivariance,Projective manifold,Monocular \(3\)D detection
AI 理解论文
溯源树
样例
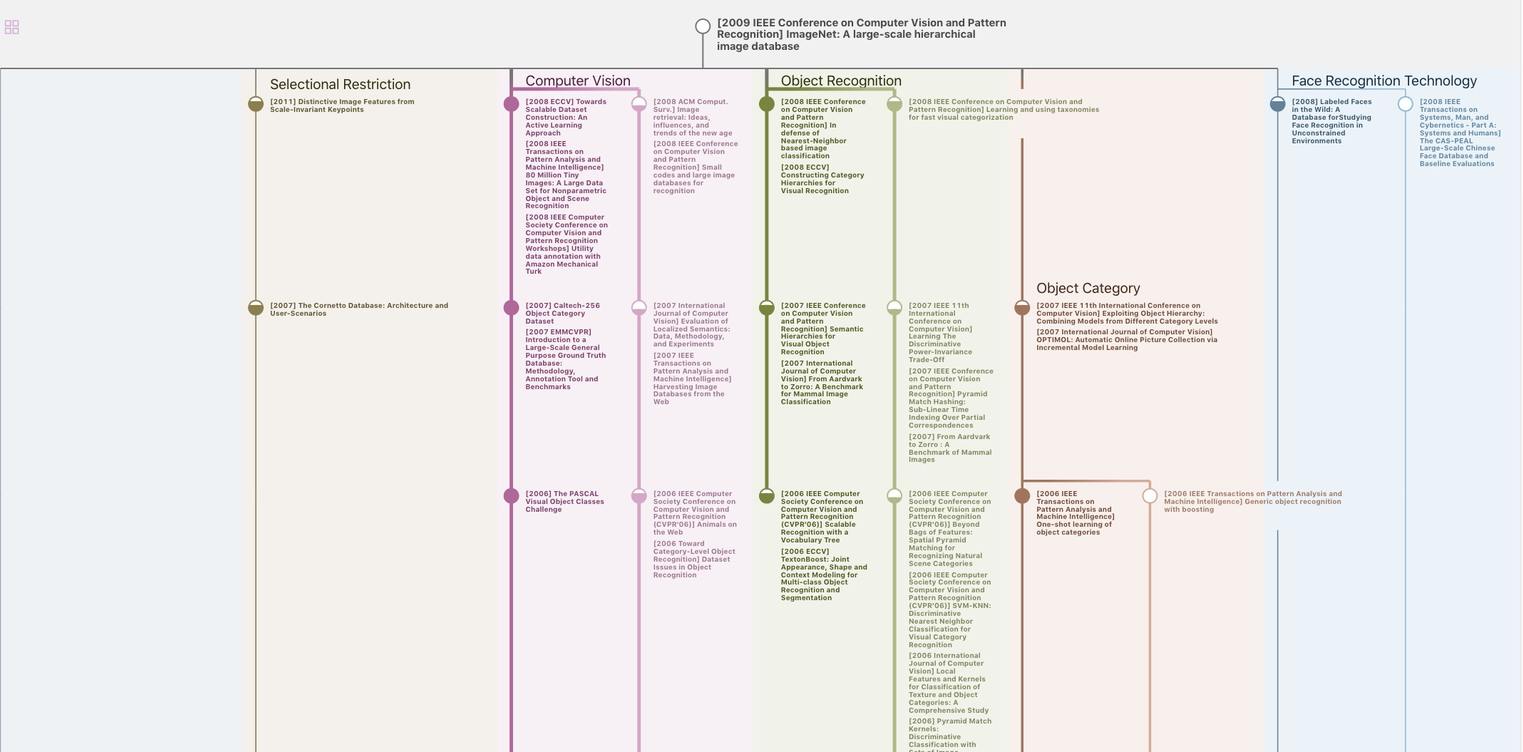
生成溯源树,研究论文发展脉络
Chat Paper
正在生成论文摘要