Identifying infected patients using semi-supervised and transfer learning
JOURNAL OF THE AMERICAN MEDICAL INFORMATICS ASSOCIATION(2022)
摘要
Objectives Early identification of infection improves outcomes, but developing models for early identification requires determining infection status with manual chart review, limiting sample size. Therefore, we aimed to compare semi-supervised and transfer learning algorithms with algorithms based solely on manual chart review for identifying infection in hospitalized patients. Materials and Methods This multicenter retrospective study of admissions to 6 hospitals included "gold-standard" labels of infection from manual chart review and "silver-standard" labels from nonchart-reviewed patients using the Sepsis-3 infection criteria based on antibiotic and culture orders. "Gold-standard" labeled admissions were randomly allocated to training (70%) and testing (30%) datasets. Using patient characteristics, vital signs, and laboratory data from the first 24 hours of admission, we derived deep learning and non-deep learning models using transfer learning and semi-supervised methods. Performance was compared in the gold-standard test set using discrimination and calibration metrics. Results The study comprised 432 965 admissions, of which 2724 underwent chart review. In the test set, deep learning and non-deep learning approaches had similar discrimination (area under the receiver operating characteristic curve of 0.82). Semi-supervised and transfer learning approaches did not improve discrimination over models fit using only silver- or gold-standard data. Transfer learning had the best calibration (unreliability index P value: .997, Brier score: 0.173), followed by self-learning gradient boosted machine (P value: .67, Brier score: 0.170). Discussion Deep learning and non-deep learning models performed similarly for identifying infection, as did models developed using Sepsis-3 and manual chart review labels. Conclusion In a multicenter study of almost 3000 chart-reviewed patients, semi-supervised and transfer learning models showed similar performance for model discrimination as baseline XGBoost, while transfer learning improved calibration.
更多查看译文
关键词
sepsis, machine learning, deep learning, time-series data analysis, AI in medicine
AI 理解论文
溯源树
样例
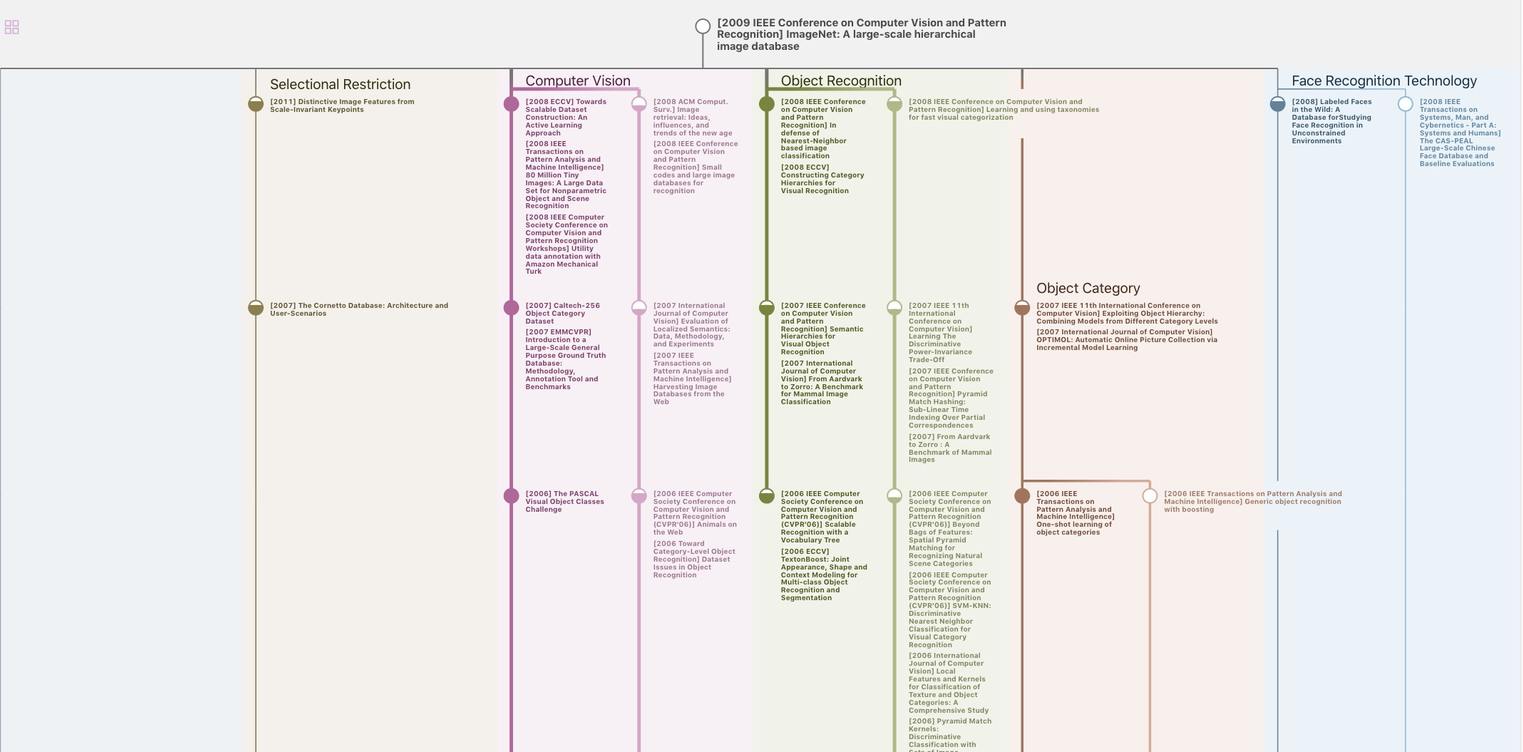
生成溯源树,研究论文发展脉络
Chat Paper
正在生成论文摘要