Nonlinearly scaled prior image-controlled frequency split for high-frequency metal artifact reduction in computed tomography
MEDICAL PHYSICS(2022)
摘要
Purpose This paper introduces a new approach for the dedicated reduction of high-frequency metal artifacts, which applies a nonlinear scaling (NLS) transfer function on the high-frequency projection domain to reduce artifacts, while preserving edge information and anatomic detail by incorporating prior image information. Methods An NLS function is applied to suppress high-frequency streak artifacts, but to restrict the correction to metal projections only, scaling is performed in the sinogram domain. Anatomic information should be preserved and is excluded from scaling by incorporating a prior image from tissue classification. The corrected high-frequency sinogram is reconstructed and combined with the low-frequency component of a normalized metal artifact reduction (NMAR) image. Scans of different anthropomorphic phantoms were acquired (unilateral hip, bilateral hip, dental implants, and embolization coil). Multiple regions of interest (ROIs) were drawn around the metal implants and hounsfield unit (HU) deviations were analyzed. Clinical data sets including single image slices of dental fillings, a bilateral hip implant, spinal fixation screws, and an aneurysm coil were reconstructed and assessed. Results The prior image-controlled NLS can remove streak artifacts while preserving anatomic detail within the bone and soft tissue. The qualitative analysis of clinical cases showed a tremendous enhancement within dental fillings and neuro coils, and a significant enhancement within spinal screws or hip implants. The phantom scan measurements support this observation. In all phantom setups, the NLS-corrected result showed lowest HU derivation and the best visualization of the data. Conclusions The prior image-controlled NLS provides a method to reduce high-frequency streaks in metal-corrupted computed tomography (CT) data.
更多查看译文
关键词
computed tomography, metal artifact reduction, metal implants
AI 理解论文
溯源树
样例
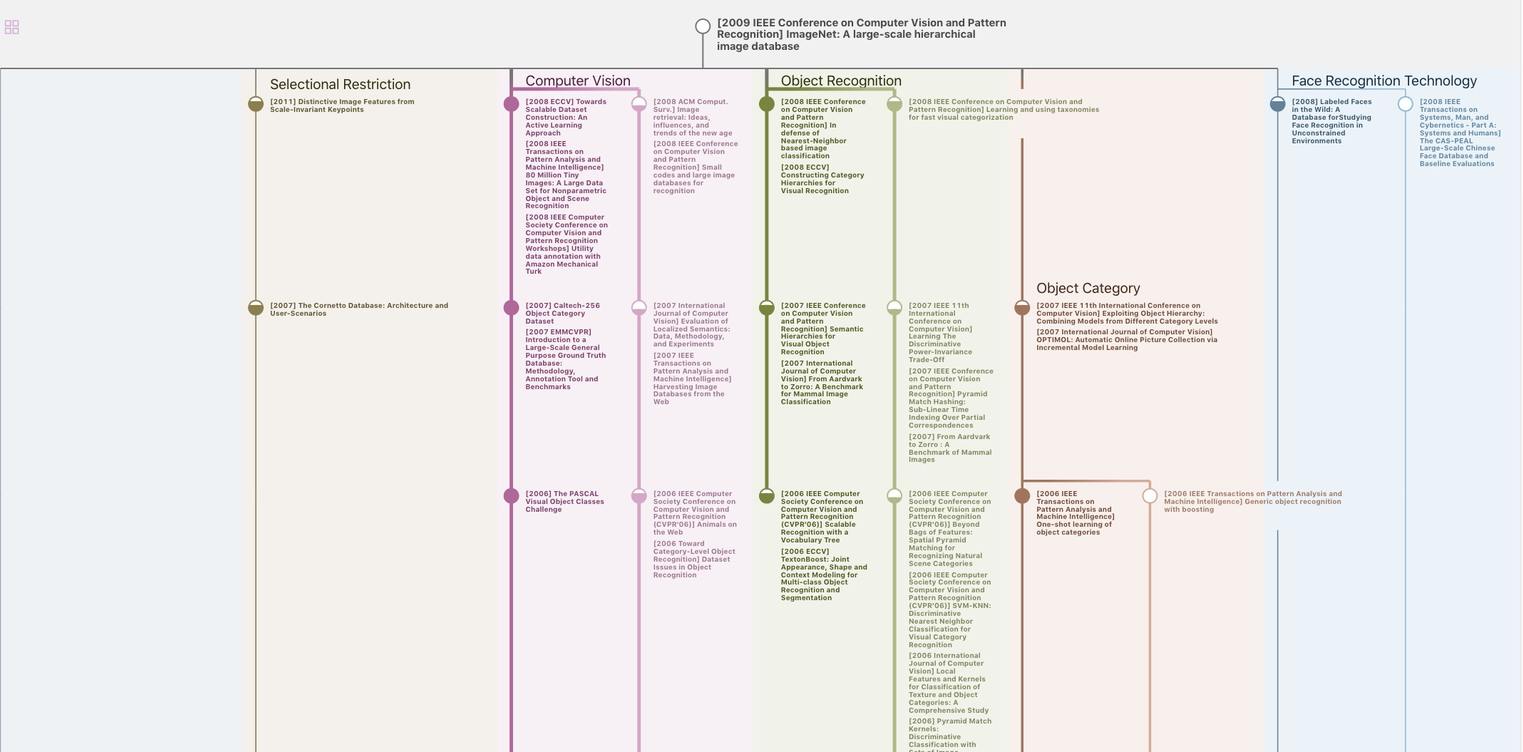
生成溯源树,研究论文发展脉络
Chat Paper
正在生成论文摘要