Fixed-Domain Posterior Contraction Rates for Spatial Gaussian Process Model with Nugget
arxiv(2023)
摘要
Spatial Gaussian process regression models typically contain finite dimensional covariance parameters that need to be estimated from the data. We study the Bayesian estimation of covariance parameters including the nugget parameter in a general class of stationary covariance functions under fixed-domain asymptotics, which is theoretically challenging due to the increasingly strong dependence among spatial observations. We propose a novel adaptation of the Schwartz's consistency theorem for showing posterior contraction rates of the covariance parameters including the nugget. We derive a new polynomial evidence lower bound, and propose consistent higher-order quadratic variation estimators that satisfy concentration inequalities with exponentially small tails. Our Bayesian fixed-domain asymptotics theory leads to explicit posterior contraction rates for the microergodic and nugget parameters in the isotropic Matern covariance function under a general stratified sampling design. We verify our theory and the Bayesian predictive performance in simulation studies and an application to sea surface temperature data. for this article are available online.
更多查看译文
关键词
Bayesian inference,Evidence lower bound,Higher-order quadratic variation,Matern covariance function
AI 理解论文
溯源树
样例
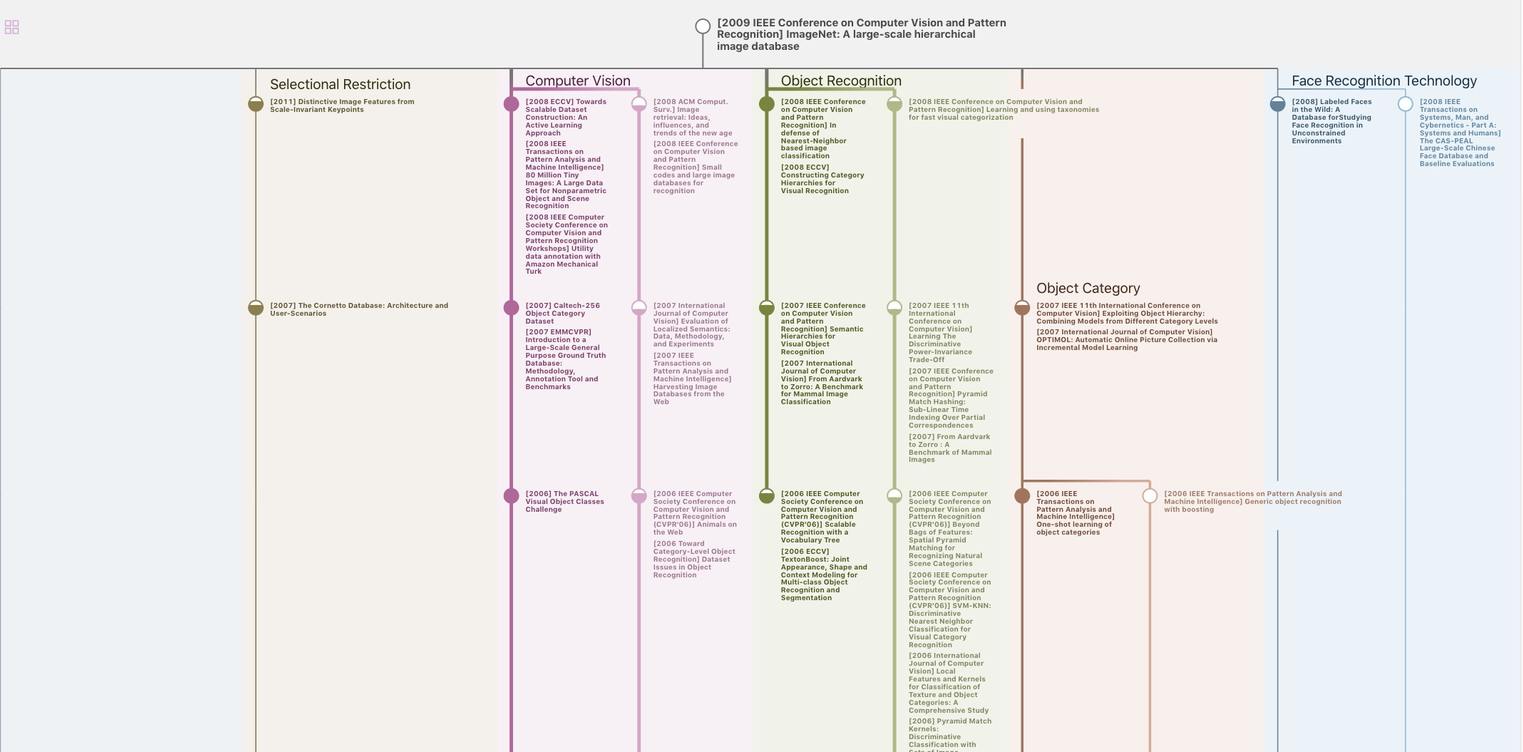
生成溯源树,研究论文发展脉络
Chat Paper
正在生成论文摘要