Who Wants to Shop With You: Joint Product-Participant Recommendation for Group-Buying Service
IEEE TRANSACTIONS ON NEURAL NETWORKS AND LEARNING SYSTEMS(2024)
摘要
Recent years have witnessed the great success of group buying (GB) in social e-commerce, opening up a new way of online shopping. In this business model, a user can launch a GB as an initiator to share her interested product with social friends. The GB is clinched once enough friends join in as participants to copurchase the shared product. As such, a successful GB depends on not only whether the initiator can find her interested product but also whether the friends are willing to join in as participants. Most existing recommenders are incompetent in such complex scenario, as they merely seek to help users find their preferred products and cannot help identify potential participants to join in a GB. To this end, we propose a novel joint product-participant recommendation (J2PRec) framework, which recommends both candidate products and participants for maximizing the success rate of a GB. Specifically, J2PRec first designs a relational graph embedding module, which effectively encodes the various relations in GB for learning enhanced user and product embeddings. It then jointly learns the product and participant recommendation tasks under a probabilistic framework to maximize the GB likelihood, i.e., boost the success rate of a GB. Extensive experiments on three real-world datasets demonstrate the superiority of J2PRec for GB recommendation.
更多查看译文
关键词
Task analysis,Electronic commerce,Social networking (online),Computer science,Toy manufacturing industry,Sun,Recommender systems,Graph neural networks,group buying (GB),recommender systems,social e-commerce
AI 理解论文
溯源树
样例
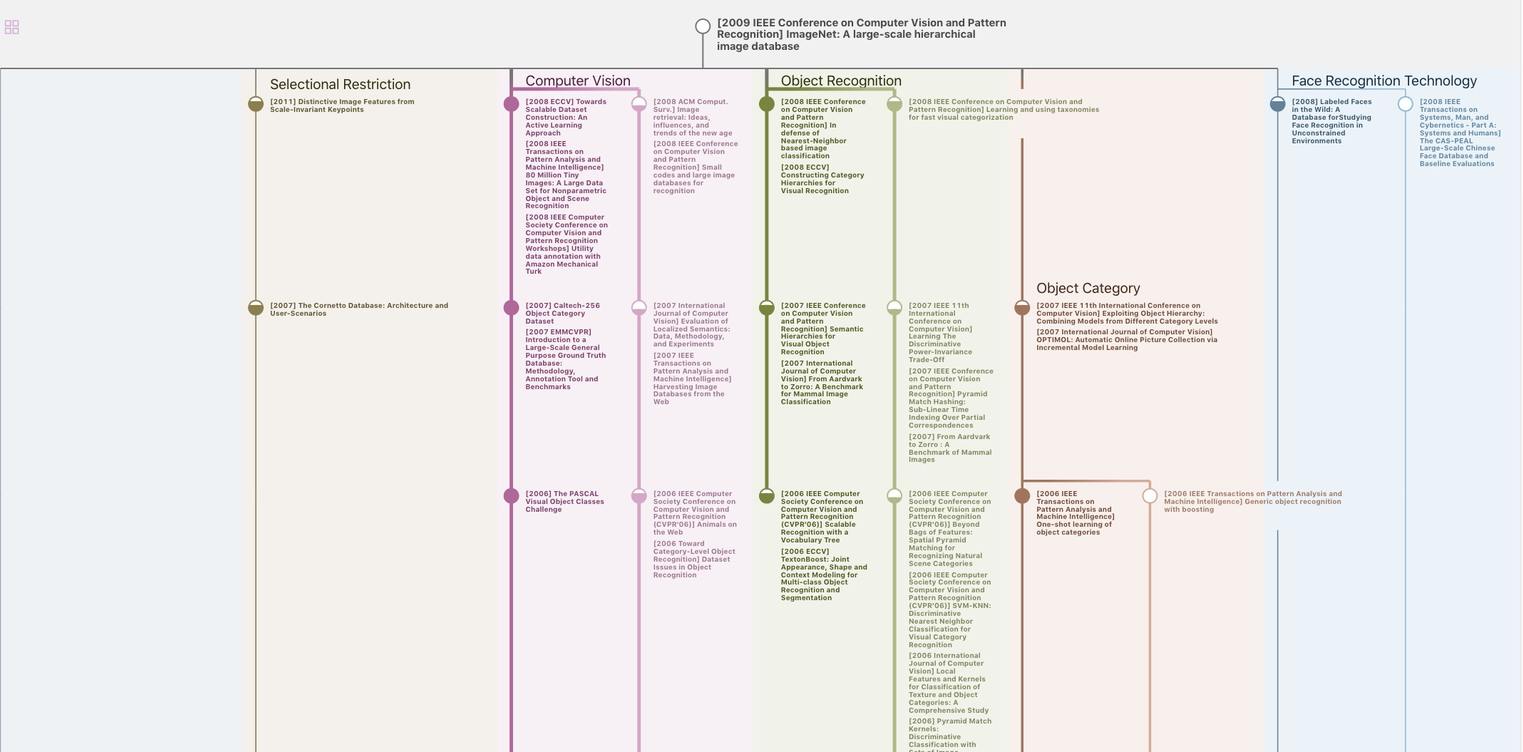
生成溯源树,研究论文发展脉络
Chat Paper
正在生成论文摘要