Continual learning via region-aware memory
Applied Intelligence(2022)
摘要
Continual learning for classification is a common learning scenario in practice yet remains an open challenge for deep neural networks (DNNs). The contemporary DNNs suffer from catastrophic forgetting—they are prone to forgetting the previously acquired knowledge when learning new tasks. Storing a small portion of samples of old tasks in an episodic memory and then replaying them when learning new tasks is an effective way to mitigate catastrophic forgetting. Due to the storage constraint, an episodic memory with limited but diverse samples is more preferable for continual learning. To select samples from various regions in the feature space, we propose a region-aware memory (RAM) construction method. Specifically, we exploit adversarial attack to approximately measure the distance of an example to its class decision boundary. Then, we uniformly choose the samples with different distances to the decision boundary, i.e. the samples from various regions, to store in the episodic memory. We evaluate our RAM on CIFAR10, CIFAR100 and ImageNet datasets in the ‘blurry’ setup Prabhu et al. ( 1 ) and Bang et al. ( 2 ). Experimental results show that our RAM can outperform state-of-the-art methods. In particular, the performance on ImageNet is boosted by 4.82%.
更多查看译文
关键词
Continual learning,Region-aware memory,Adversarial attack,Diverse samples
AI 理解论文
溯源树
样例
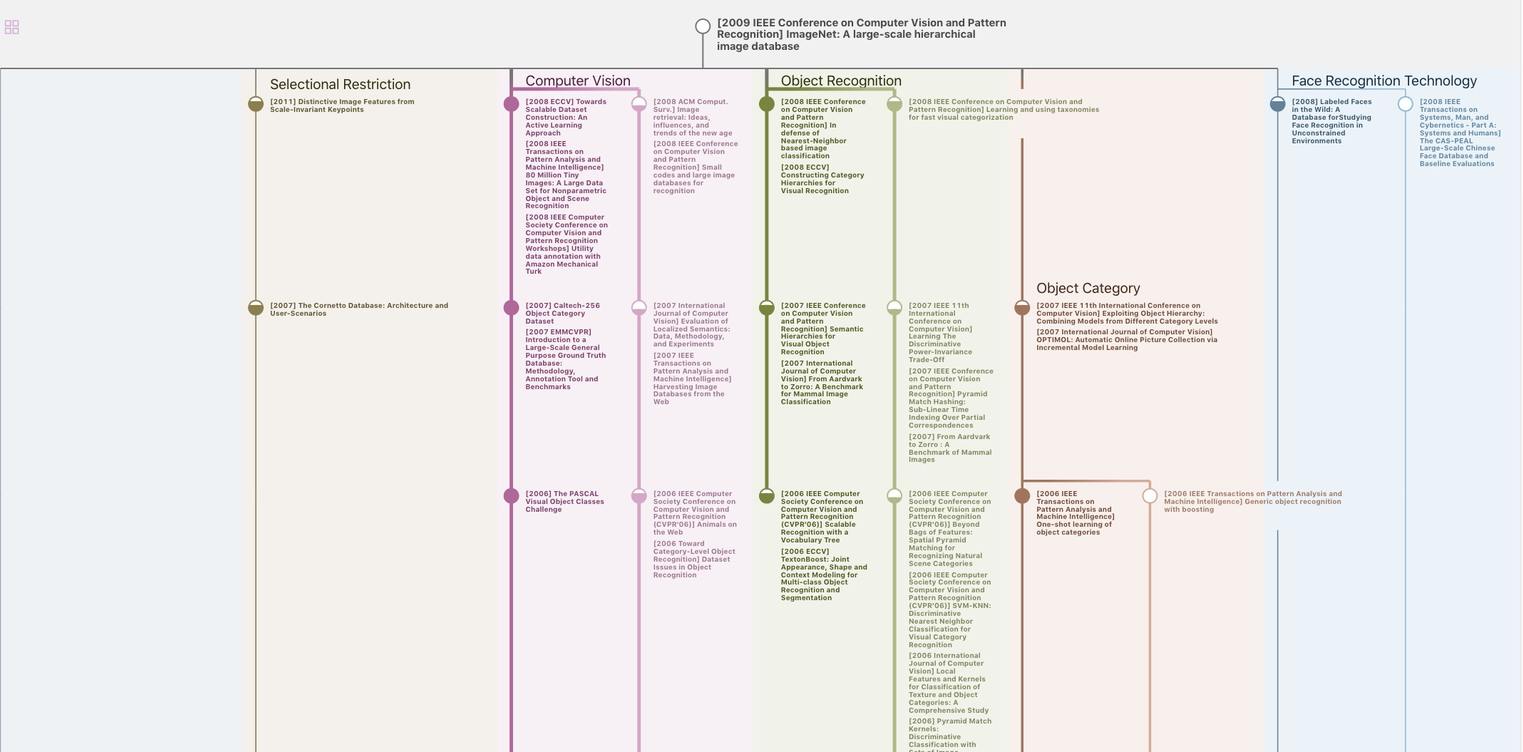
生成溯源树,研究论文发展脉络
Chat Paper
正在生成论文摘要