Online Failure Prediction for Complex Systems: Methodology and Case Studies
IEEE Transactions on Dependable and Secure Computing(2023)
摘要
Online Failure Prediction (OFP) allows proactively taking countermeasures before a failure occurs, such as saving data or restarting a system. However, despite its potential contribution to improving dependability, OFP still presents key limitations. Besides the problem of choosing the optimal set of features, assessing predictive models is complex and common procedures for supporting comparison are not available. There is, in fact, little work on developing and assessing failure predictors for complex systems. In this aricle, we present two extensive case studies on distinct Operating Systems (OSs), Linux and Windows, showing that it is possible to create models that can predict different types of incoming failures, highlighting various important considerations such as the operational requirements of the target system. To drive the case studies, we define a well-structured framework for a fair and sound assessment and comparison of alternative predictive solutions. It includes scenarios for choosing the most adequate metrics for the assessment, comparing alternative models, and selecting the best predictor, while considering the need to tolerate perturbations in the data. In practice, we show that, by following a well-defined process, it is possible to develop accurate failure predictors and establish a ranking of the models under evaluation in different scenarios and OSs.
更多查看译文
关键词
Online failure prediction,reliability,availability,machine learning
AI 理解论文
溯源树
样例
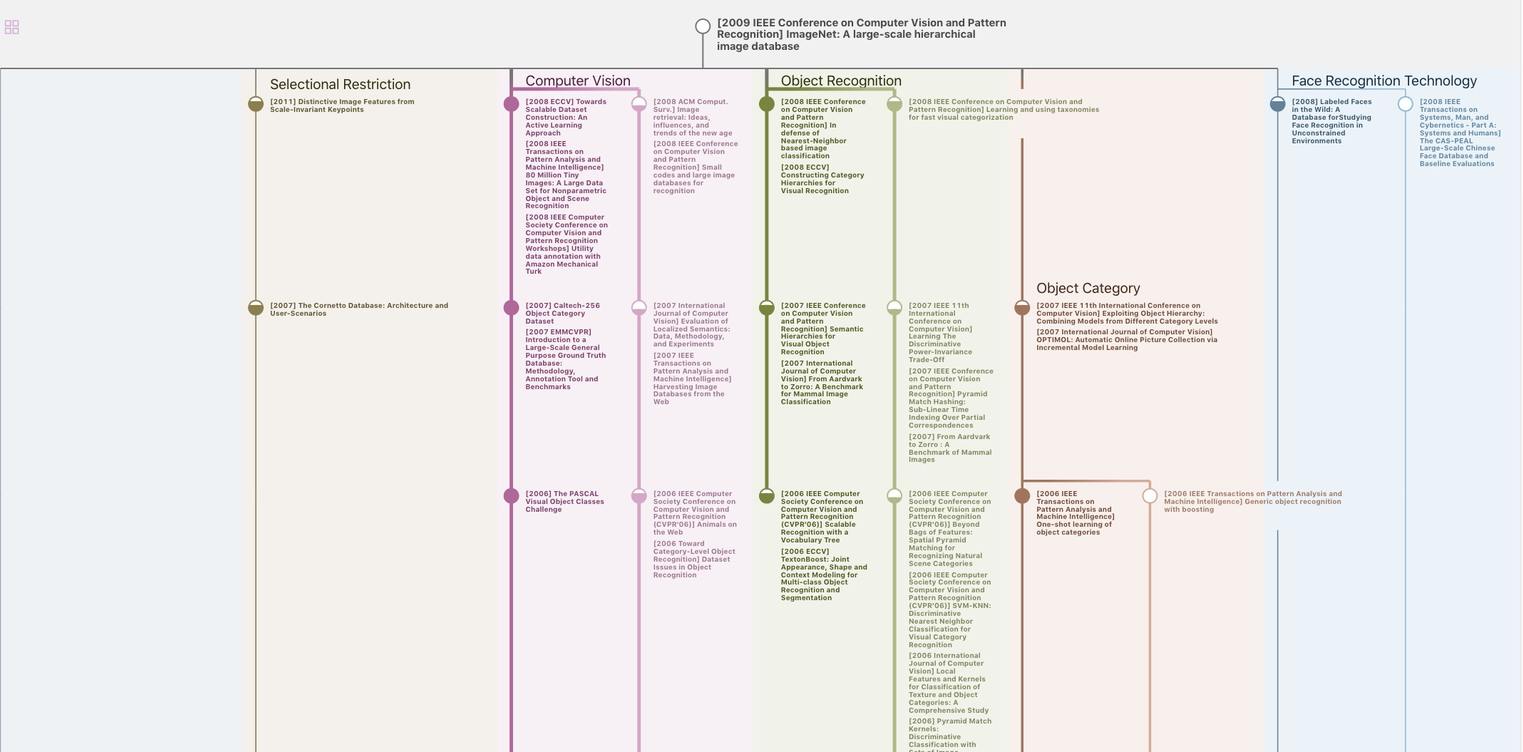
生成溯源树,研究论文发展脉络
Chat Paper
正在生成论文摘要