Generative Text Convolutional Neural Network for Hierarchical Document Representation Learning
IEEE Transactions on Pattern Analysis and Machine Intelligence(2023)
摘要
For document analysis, existing methods often resort to the document representation that either discards the word order information or projects each word into a low-dimensional dense embedding vector. However, confined by the data's sparsity and high-dimensionality, limited effort has been made to explore the semantic structures underlying the document representation that formulates each document as a sequence of one-hot vectors, especially in the probabilistic modeling literature. To construct a probabilistic generative model for this type of document representation, we first develop convolutional Poisson factor analysis (CPFA) that not only utilizes the sparse property of data but also enables model parallelism. Through interleaving probabilistic Dirichlet-gamma pooling layers with learnable parameters, we extend the shallow CPFA into a generative text convolutional neural network (GTCNN), which captures richer semantic information with multiple probabilistic convolutional layers and can be coupled with existing deep topic models to alleviate their loss of word order. For efficient and scalable model inference, we not only develop both a parallel upward-downward Gibbs sampler and SG-MCMC based algorithm for training GTCNN, but also construct a hierarchical Weibull convolutional inference network for fast out-of-sample prediction. Experimental results on document representation learning tasks demonstrate the effectiveness of the proposed methods.
更多查看译文
关键词
Probabilistic logic,Computational modeling,Semantics,Analytical models,Task analysis,Vocabulary,Data models,Document analysis,deep topic models,probabilistic convolutional generative models,variational autoencoder
AI 理解论文
溯源树
样例
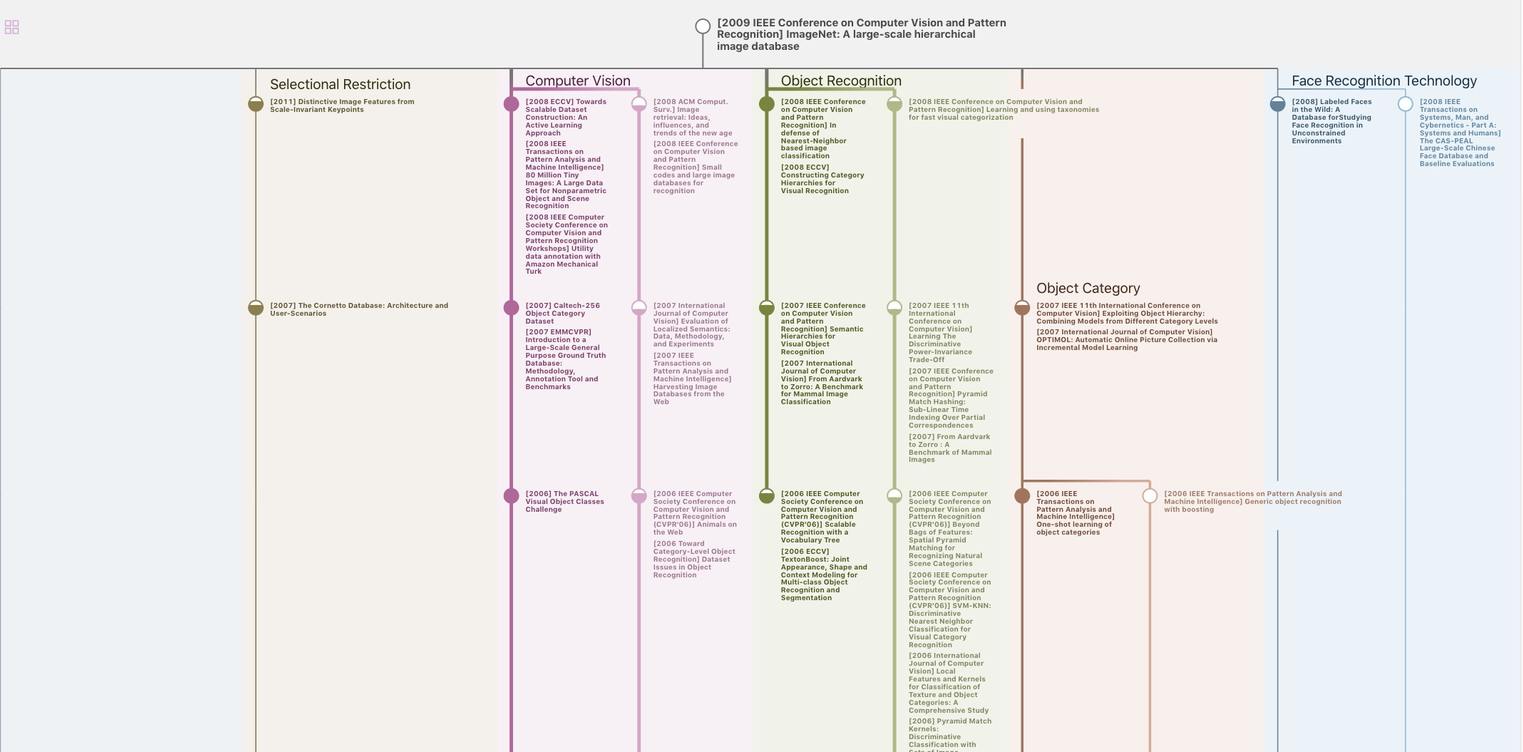
生成溯源树,研究论文发展脉络
Chat Paper
正在生成论文摘要