Generative Domain Adaptation for Face Anti-Spoofing.
European Conference on Computer Vision(2022)
Abstract
Face anti-spoofing (FAS) approaches based on unsupervised domain adaption (UDA) have drawn growing attention due to promising performances for target scenarios. Most existing UDA FAS methods typically fit the trained models to the target domain via aligning the distribution of semantic high-level features. However, insufficient supervision of unlabeled target domains and neglect of low-level feature alignment degrade the performances of existing methods. To address these issues, we propose a novel perspective of UDA FAS that directly fits the target data to the models, i.e., stylizes the target data to the source-domain style via image translation, and further feeds the stylized data into the well-trained source model for classification. The proposed Generative Domain Adaptation (GDA) framework combines two carefully designed consistency constraints: 1) Inter-domain neural statistic consistency guides the generator in narrowing the inter-domain gap. 2) Dual-level semantic consistency ensures the semantic quality of stylized images. Besides, we propose intra-domain spectrum mixup to further expand target data distributions to ensure generalization and reduce the intra-domain gap. Extensive experiments and visualizations demonstrate the effectiveness of our method against the state-of-the-art methods.
MoreTranslated text
Key words
Face anti-spoofing,Unsupervised domain adaptation
AI Read Science
Must-Reading Tree
Example
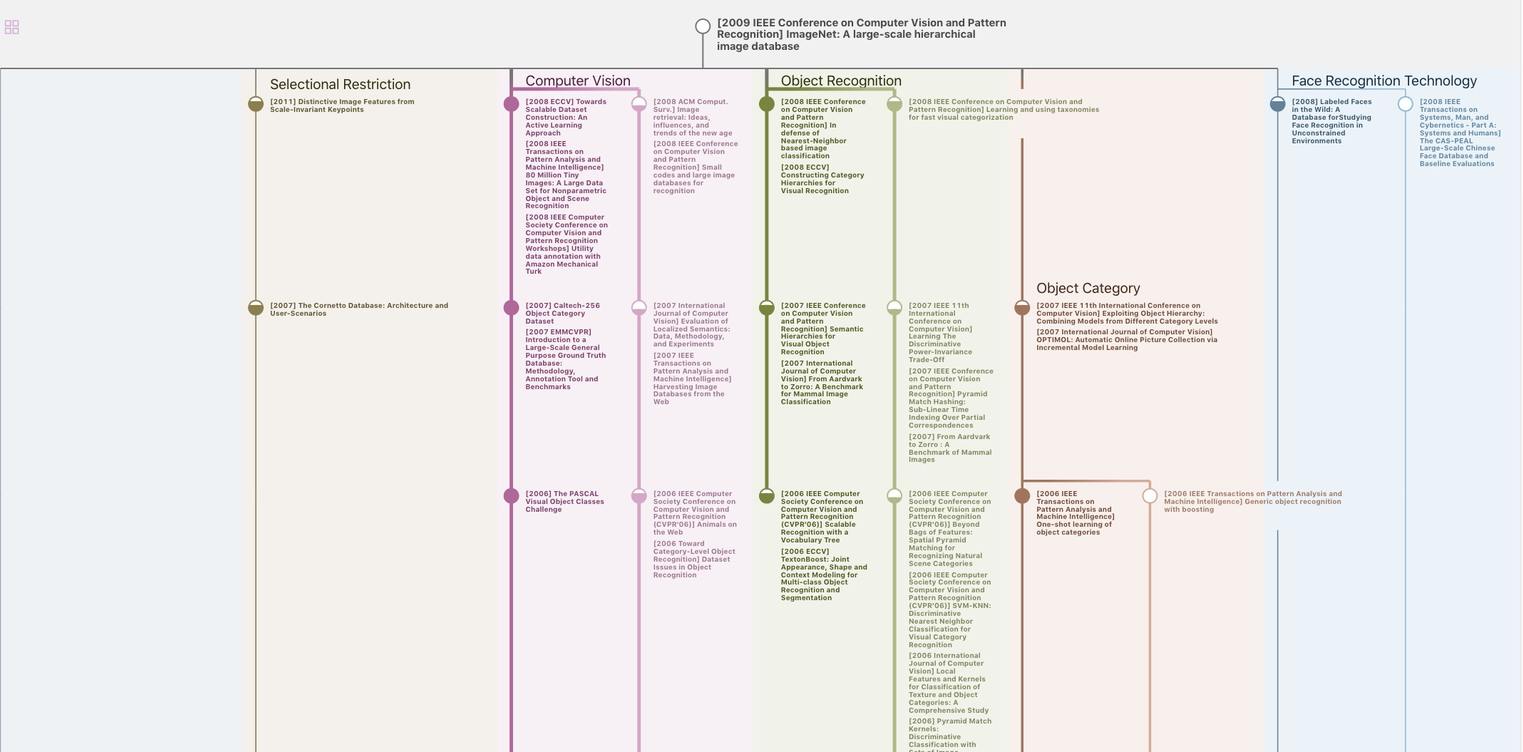
Generate MRT to find the research sequence of this paper
Chat Paper
Summary is being generated by the instructions you defined