Learning Counterfactually Invariant Predictors
ICLR 2023(2022)
摘要
We propose a method to learn predictors that are invariant under counterfactual changes of certain covariates. This method is useful when the prediction target is causally influenced by covariates that should not affect the predictor output. For instance, an object recognition model may be influenced by position, orientation, or scale of the object itself. We address the problem of training predictors that are explicitly counterfactually invariant to changes of such covariates. We propose a model-agnostic regularization term based on conditional kernel mean embeddings, to enforce counterfactual invariance during training. We prove the soundness of our method, which can handle mixed categorical and continuous multi-variate attributes. Empirical results on synthetic and real-world data demonstrate the efficacy of our method in a variety of settings.
更多查看译文
关键词
causality,kernel mean embeddings,counterfactual fairness,counterfactual invariance
AI 理解论文
溯源树
样例
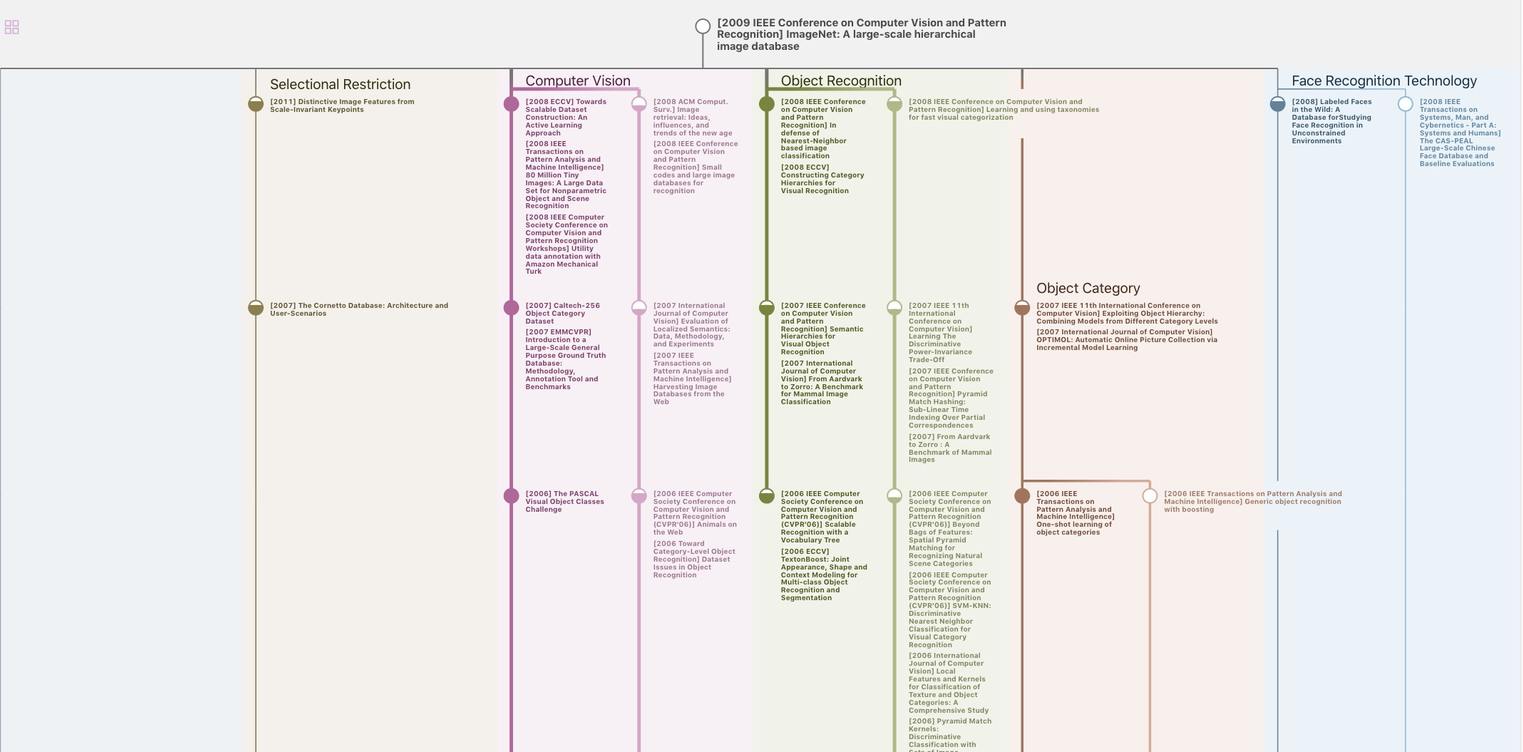
生成溯源树,研究论文发展脉络
Chat Paper
正在生成论文摘要