Data-driven initialization of deep learning solvers for Hamilton-Jacobi-Bellman PDEs
IFAC-PapersOnLine(2022)
摘要
A deep learning approach for the approximation of the Hamilton-Jacobi-Bellman partial differential equation (HJB PDE) associated to the Nonlinear Quadratic Regulator (NLQR) problem. A state-dependent Riccati equation control law is first used to generate a gradient-augmented synthetic dataset for supervised learning. The resulting model becomes a warm start for the minimization of a loss function based on the residual of the HJB PDE. The combination of supervised learning and residual minimization avoids spurious solutions and mitigate the data inefficiency of a supervised learning-only approach. Numerical tests validate the different advantages of the proposed methodology.
更多查看译文
关键词
Hamilton-Jacobi-Bellman PDE,NLQR,supervised learning,residual minimization
AI 理解论文
溯源树
样例
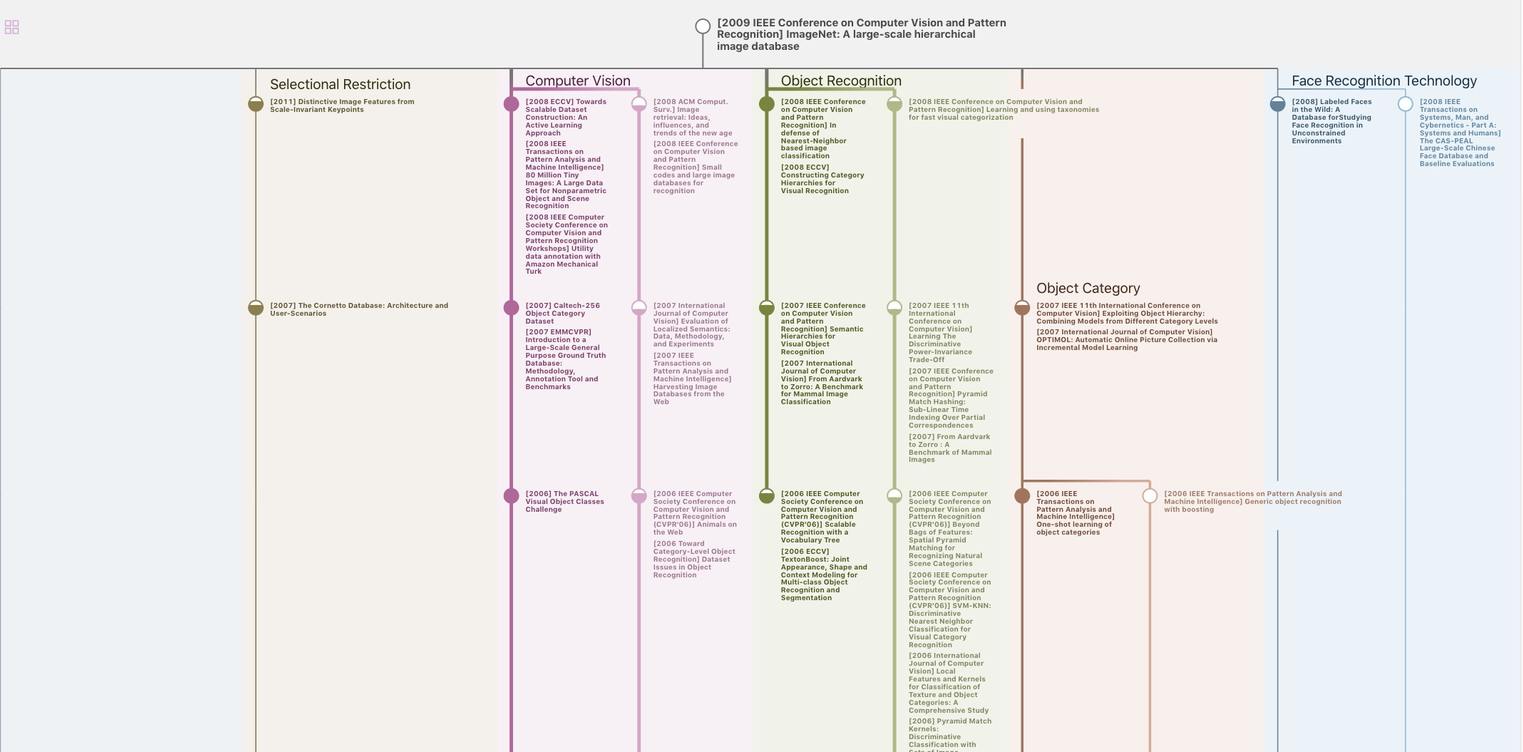
生成溯源树,研究论文发展脉络
Chat Paper
正在生成论文摘要