EVE: Environmental Adaptive Neural Network Models for Low-Power Energy Harvesting System.
ICCAD(2022)
摘要
IoT devices are increasingly being implemented with neural network models to enable smart applications. Energy harvesting (EH) technology that harvests energy from ambient environment is a promising alternative to batteries for powering those devices due to the low maintenance cost and wide availability of the energy sources. However, the power provided by the energy harvester is low and has an intrinsic drawback of instability since it varies with the ambient environment. This paper proposes EVE, an automated machine learning (autoML) co-exploration framework to search for desired multi-models with shared weights for energy harvesting IoT devices. Those shared models incur significantly reduced memory footprint with different levels of model sparsity, latency, and accuracy to adapt to the environmental changes. An efficient on-device implementation architecture is further developed to efficiently execute each model on device. A run-time model extraction algorithm is proposed that retrieves individual model with negligible overhead when a specific model mode is triggered.Experimental results show that the neural networks models generated by EVE is on average 2.5X times faster than the baseline models without pruning and shared weights.
更多查看译文
关键词
Energy Harvesting,DNN,Pruning,IoT,Memory Footprint
AI 理解论文
溯源树
样例
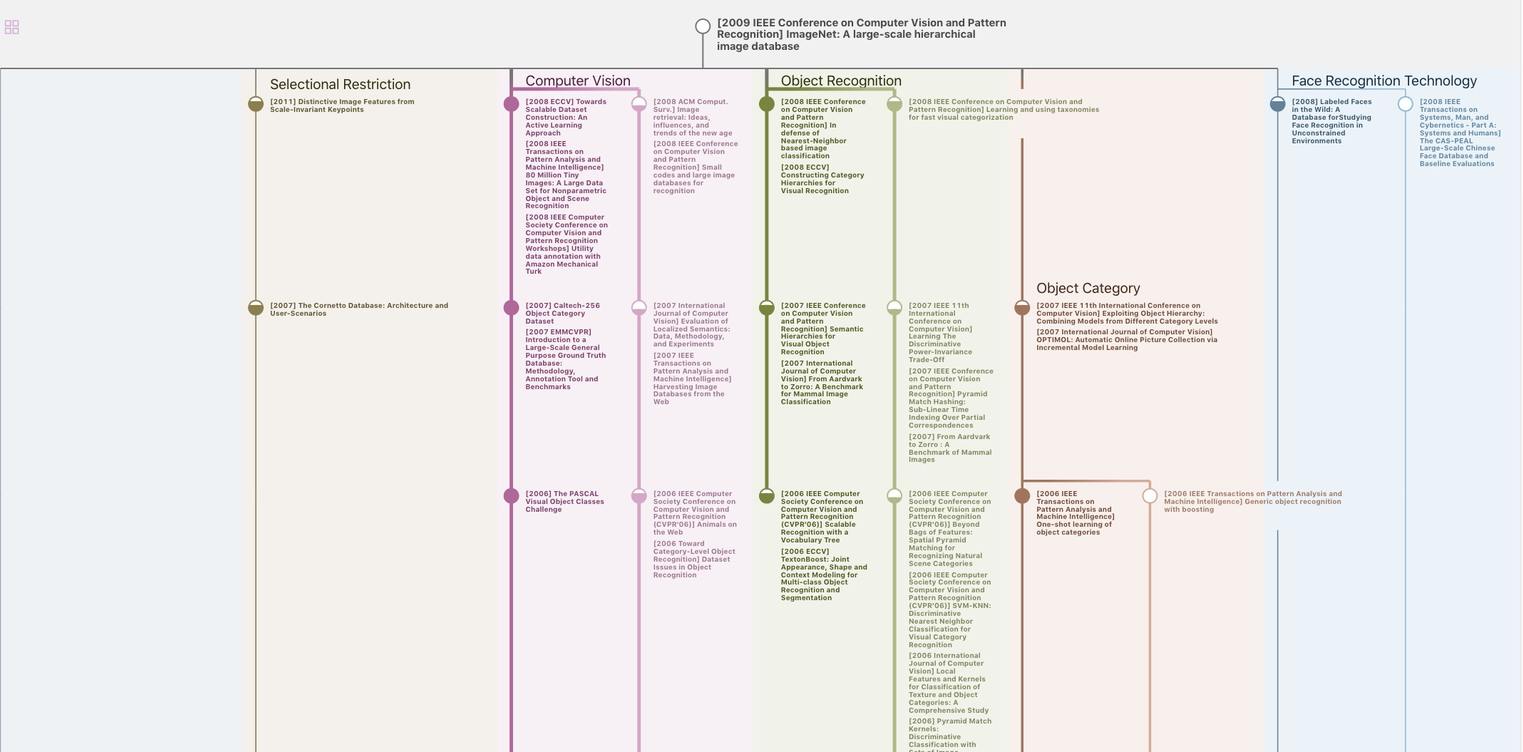
生成溯源树,研究论文发展脉络
Chat Paper
正在生成论文摘要