Benchmarking Transformers-based models on French Spoken Language Understanding tasks
Conference of the International Speech Communication Association (INTERSPEECH)(2022)
摘要
In the last five years, the rise of the self-attentional Transformer-based architectures led to state-of-the-art performances over many natural language tasks. Although these approaches are increasingly popular, they require large amounts of data and computational resources. There is still a substantial need for benchmarking methodologies ever upwards on under-resourced languages in data-scarce application conditions. Most pre-trained language models were massively studied using the English language and only a few of them were evaluated on French. In this paper, we propose a unified benchmark, focused on evaluating models quality and their ecological impact on two well-known French spoken language understanding tasks. Especially we benchmark thirteen well-established Transformer-based models on the two available spoken language understanding tasks for French: MEDIA and ATIS-FR. Within this framework, we show that compact models can reach comparable results to bigger ones while their ecological impact is considerably lower. However, this assumption is nuanced and depends on the considered compression method.
更多查看译文
关键词
benchmarking,language,models,transformers-based
AI 理解论文
溯源树
样例
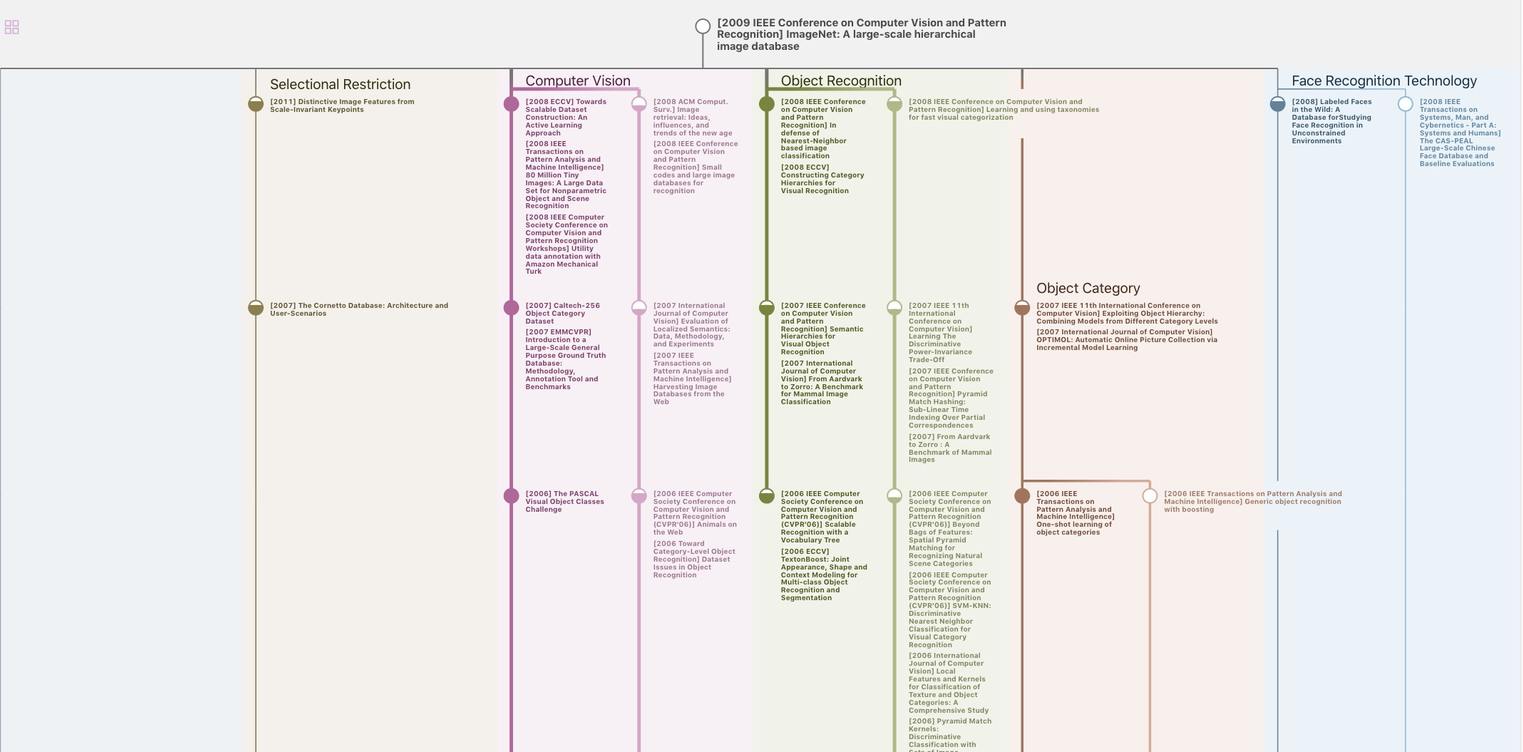
生成溯源树,研究论文发展脉络
Chat Paper
正在生成论文摘要