SCHA-VAE: Hierarchical Context Aggregation for Few-Shot Generation.
International Conference on Machine Learning(2022)
摘要
A few-shot generative model should be able to generate data from a novel distribution by only observing a limited set of examples. In few-shot learning the model is trained on data from many sets from distributions sharing some underlying properties such as sets of characters from different alphabets or objects from different categories. We extend current latent variable models for sets to a fully hierarchical approach with an attention-based point to set-level aggregation and call our method SCHA-VAE for Set-Context-Hierarchical-Aggregation Variational Autoencoder. We explore likelihood-based model comparison, iterative data sampling, and adaptation-free out-of-distribution generalization. Our results show that the hierarchical formulation better captures the intrinsic variability within the sets in the small data regime. This work generalizes deep latent variable approaches to few-shot learning, taking a step toward large-scale few-shot generation with a formulation that readily works with current state-of-the-art deep generative models.
更多查看译文
关键词
hierarchical context aggregation,generation,scha-vae,few-shot
AI 理解论文
溯源树
样例
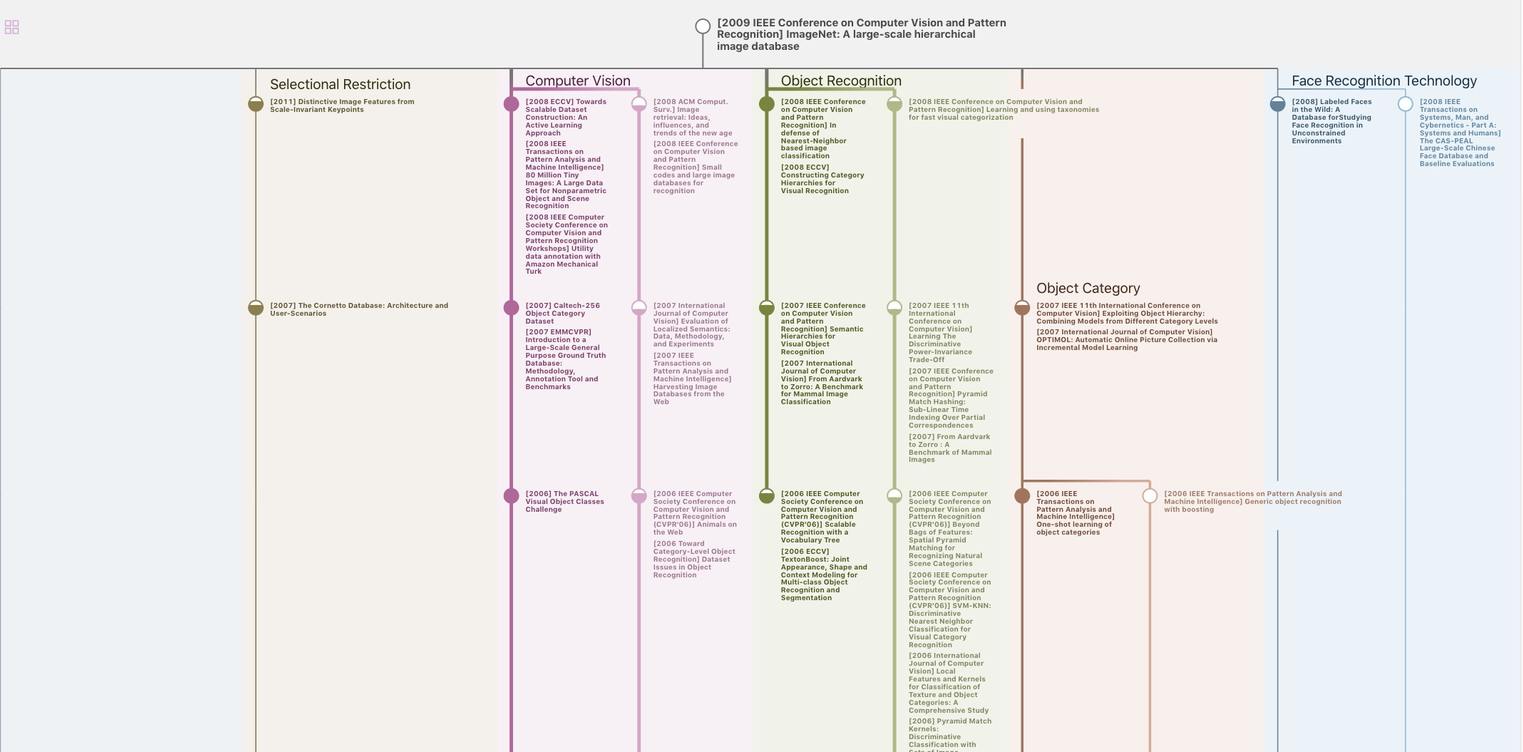
生成溯源树,研究论文发展脉络
Chat Paper
正在生成论文摘要