CA-SpaceNet: Counterfactual Analysis for 6D Pose Estimation in Space.
IEEE/RJS International Conference on Intelligent RObots and Systems (IROS)(2022)
Abstract
Reliable and stable 6D pose estimation of uncooperative space objects plays an essential role in on-orbit servicing and debris removal missions. Considering that the pose estimator is sensitive to background interference, this paper proposes a counterfactual analysis framework named CASpaceNet to complete robust 6D pose estimation of the spaceborne targets under complicated background. Specifically, conventional methods are adopted to extract the features of the whole image in the factual case. In the counterfactual case, a non-existent image without the target but only the background is imagined. Side effect caused by background interference is reduced by counterfactual analysis, which leads to unbiased prediction in final results. In addition, we also carry out lowbit-width quantization for CA-SpaceNet and deploy part of the framework to a Processing-In-Memory (PIM) accelerator on FPGA. Qualitative and quantitative results demonstrate the effectiveness and efficiency of our proposed method. To our best knowledge, this paper applies causal inference and network quantization to the 6D pose estimation of space-borne targets for the first time. The code is available at https://github.com/Shunli-Wang/CA-SpaceNet.
MoreTranslated text
Key words
background interference,complicated background,counterfactual analysis framework,counterfactual case,debris removal missions,nonexistent image,on-orbit servicing,pose estimator,reliable D pose estimation,robust 6D pose estimation,space objects,space-borne targets,stable 6D pose estimation
AI Read Science
Must-Reading Tree
Example
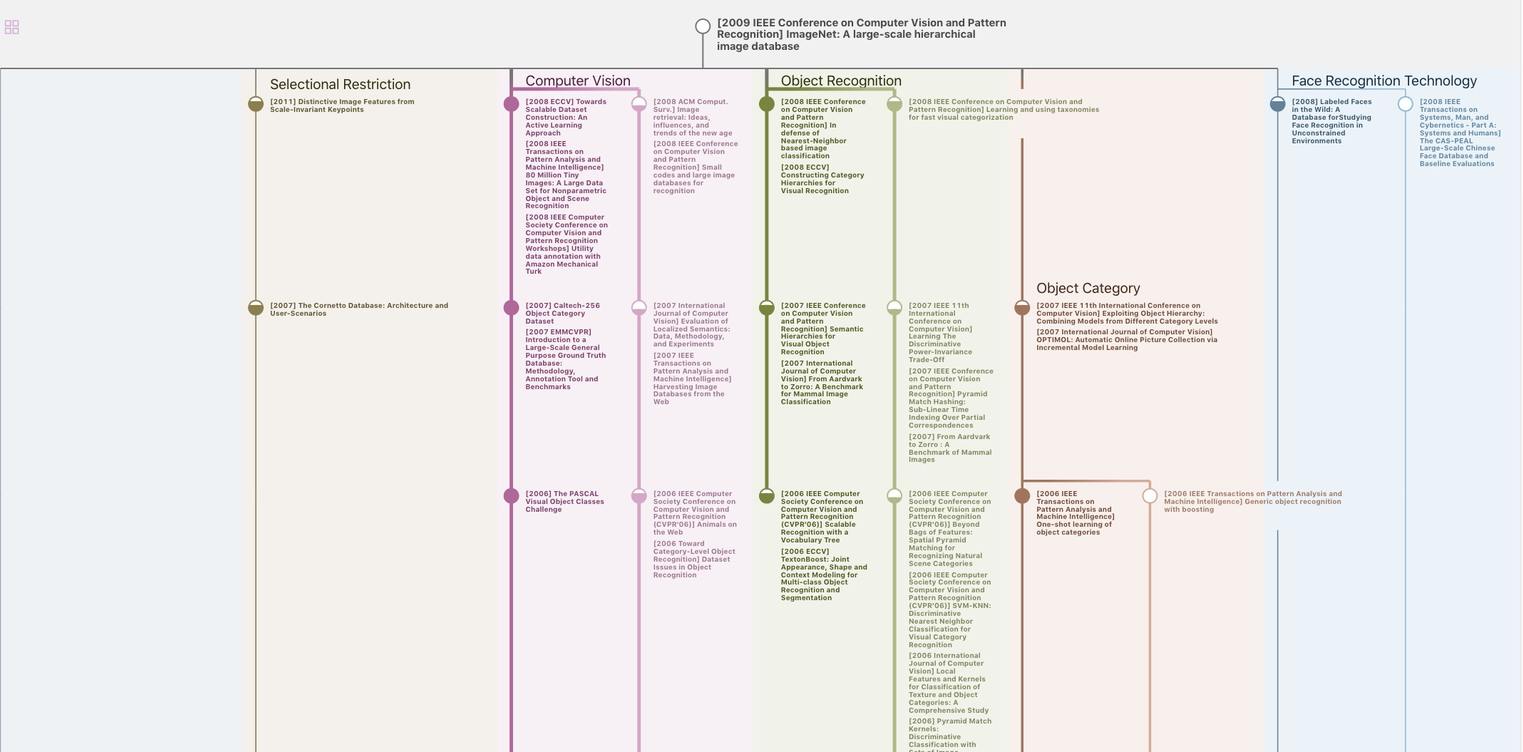
Generate MRT to find the research sequence of this paper
Chat Paper
Summary is being generated by the instructions you defined