Untrained, physics-informed neural networks for structured illumination microscopy
Optics express(2023)
摘要
Structured illumination microscopy (SIM) is a popular super-resolution imaging technique that can achieve resolution improvements of 2x and greater depending on the illumination patterns used. Traditionally, images are reconstructed using the linear SIM reconstruction algorithm. However, this algorithm has hand-tuned parameters which can often lead to artifacts, and it cannot be used with more complex illumination patterns. Recently, deep neural networks have been used for SIM reconstruction, yet they require training sets that are difficult to capture experimentally. We demonstrate that we can combine a deep neural network with the forward model of the structured illumination process to reconstruct sub-diffraction images without training data. The resulting physics-informed neural network (PINN) can be optimized on a single set of diffraction-limited sub-images and thus does not require any training set. We show, with simulated and experimental data, that this PINN can be applied to a wide variety of SIM illumination methods by simply changing the known illumination patterns used in the loss function and can achieve resolution improvements that match theoretical expectations.
更多查看译文
关键词
structured illumination microscopy,neural networks,physics-informed
AI 理解论文
溯源树
样例
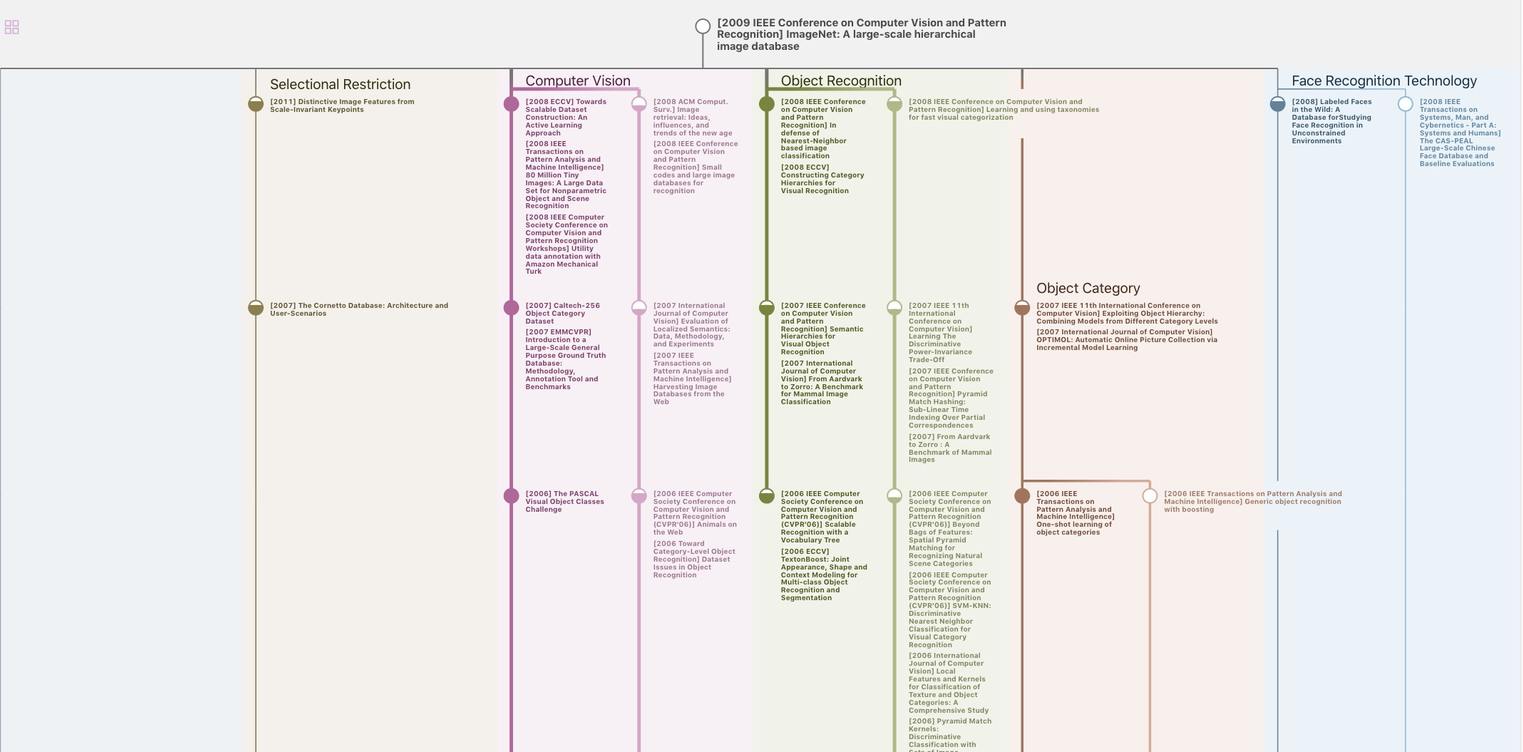
生成溯源树,研究论文发展脉络
Chat Paper
正在生成论文摘要