Rapid prediction of protein natural frequencies using graph neural networks
Digital discovery(2022)
摘要
Natural vibrational frequencies of proteins help to correlate functional shifts with sequence or geometric variations that lead to negligible changes in protein structures, such as point mutations related to disease lethality or medication effectiveness. Normal mode analysis is a well-known approach to accurately obtain protein natural frequencies. However, it is not feasible when high-resolution protein structures are not available or time consuming to obtain. Here we provide a machine learning model to directly predict protein frequencies from primary amino acid sequences and low-resolution structural features such as contact or distance maps. We utilize a graph neural network called principal neighborhood aggregation, trained with the structural graphs and normal mode frequencies of more than 34 000 proteins from the protein data bank. combining with existing contact/distance map prediction tools, this approach enables an end-to-end prediction of the frequency spectrum of a protein given its primary sequence. We present a computational framework based on graph neural networks (GNNs) to predict the natural frequencies of proteins from primary amino acid sequences and contact/distance maps.
更多查看译文
关键词
natural frequencies,graph,protein,rapid prediction,neural networks
AI 理解论文
溯源树
样例
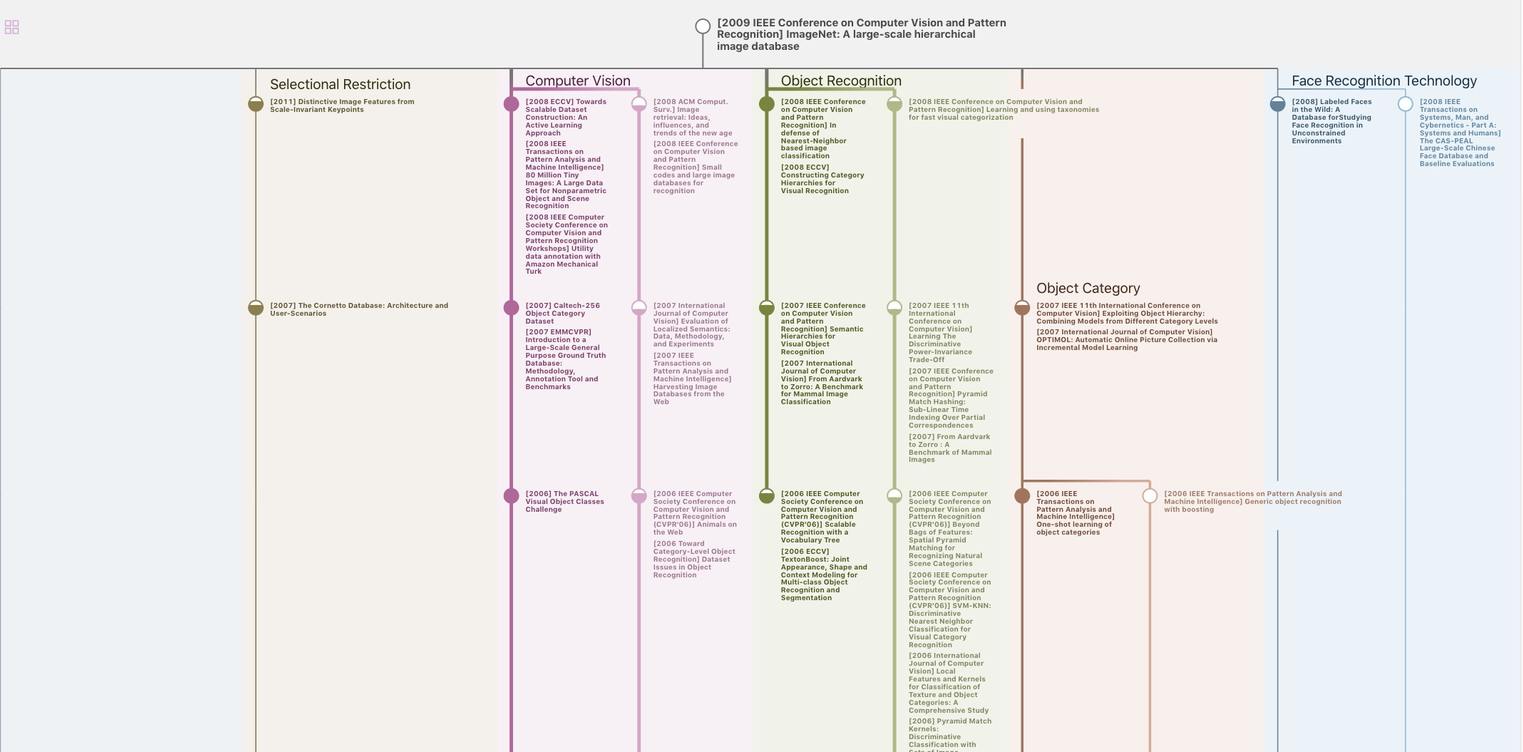
生成溯源树,研究论文发展脉络
Chat Paper
正在生成论文摘要