Machine learning algorithms to predict flow boiling pressure drop in mini/micro-channels based on universal consolidated data
International Journal of Heat and Mass Transfer(2021)
摘要
Two-phase flow in mini/micro-channels can meet the high heat dissipation requirements of many state-of-the-art cooling solutions. However, there is lack of accurate universal methods for predicting parameters like pressure drop in these configurations. Conventional ways of predicting pressure drop employ either Homogeneous Equilibrium Model (HEM) or semi-empirical correlations. This current study leverages the availability of data collected over the past few decades to build several machine learning models to demonstrate the efficacy and ease of building and deploying such models. A consolidated database of 2787 data points for flow boiling pressure drop in mini/micro-channels is amassed from 21 sources that includes 10 working fluid, reduced pressures of 0.0006 –0.7766, hydraulic diameters of 0.15–5.35 mm, mass velocities of 33.1 < G < 2738 kg/m 2 s, liquid-only Reynolds numbers of 14–27,658, superficial vapor Reynolds number of 75.58–199,453 and flow qualities of 0 and 1. This consolidated database is utilized to develop four machine learning based regression models viz., Artificial Neural Networks (ANN), KNN regression, Extreme Gradient Boosting (XGBoost) and Light GBM. Both input parameters and hyperparameters are optimized for the individual models. The models with dimensionless input parameters: Bd, Bo, Frf, Frfo, Frg, Frgo, Frtp, Prf, Prg, Peg, Pef, Ref, Refo, Reg, Rego, Reeq, Suf, Sug, Wef, Wefo, Weg, Wego,Wetp predict the test data for ANN model, XGBoost model, KNN model, and LightGBM model with MAEs of 9.58%, 10.38%, 13.52%, and 14.49%, respectively. The optimized machine-learning models performed better than highly reliable generalized pressure drop correlations plus showed good performance across individual datasets, flow regimes, and channel configurations.
更多查看译文
关键词
Machine learning,Neural networks,Ann,XGBoost,KNN,Light GBM,Flow boiling,Pressure drop
AI 理解论文
溯源树
样例
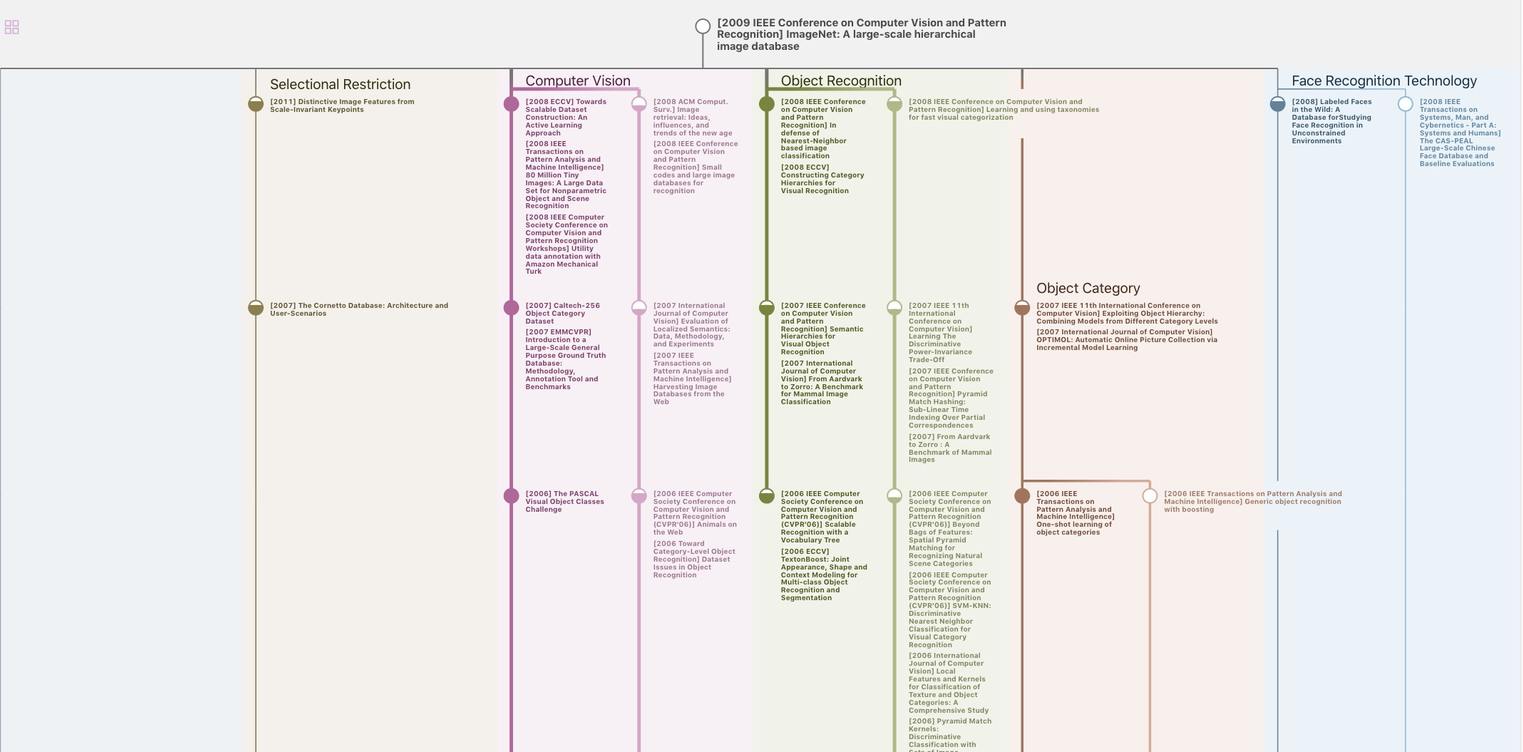
生成溯源树,研究论文发展脉络
Chat Paper
正在生成论文摘要