Missing Values for Classification of Machine Learning in Medical data
2022 5th International Conference on Artificial Intelligence and Big Data (ICAIBD)(2022)
摘要
Missing values are an unavoidable problem for classification tasks of machine learning in medical data. With the rapid development of the medical system, large scale medical data is increasing. Missing values increase the difficulty of mining hidden but useful information in these medical datasets. Deletion and imputation methods are the most popular methods for dealing with missing values. Existing studies ignored to compare and discuss the deletion and imputation methods of missing values under the row missing rate and the total missing rate. Meanwhile, they rarely used experiment data sets that are mixed-type and large scale. In this work, medical data sets of various sizes and mixed-type are used. At the same time, performance differences of deletion and imputation methods are compared under the MCAR (Missing Completely At Random) mechanism in the baseline task using LR (Linear Regression) and SVM (Support Vector Machine) classifiers for classification with the same row and total missing rates. Experimental results show that under the MCAR missing mechanism, the performance of two types of processing methods is related to the size of datasets and missing rates. As the increasing of missing rate, the performance of two types for processing missing values decreases, but the deletion method decreases faster, and the imputation methods based on machine learning have more stable and better classification performance on average. In addition, small data sets are easily affected by processing methods of missing values.
更多查看译文
关键词
missing values,medical data,imputation,deletion,mixed-type
AI 理解论文
溯源树
样例
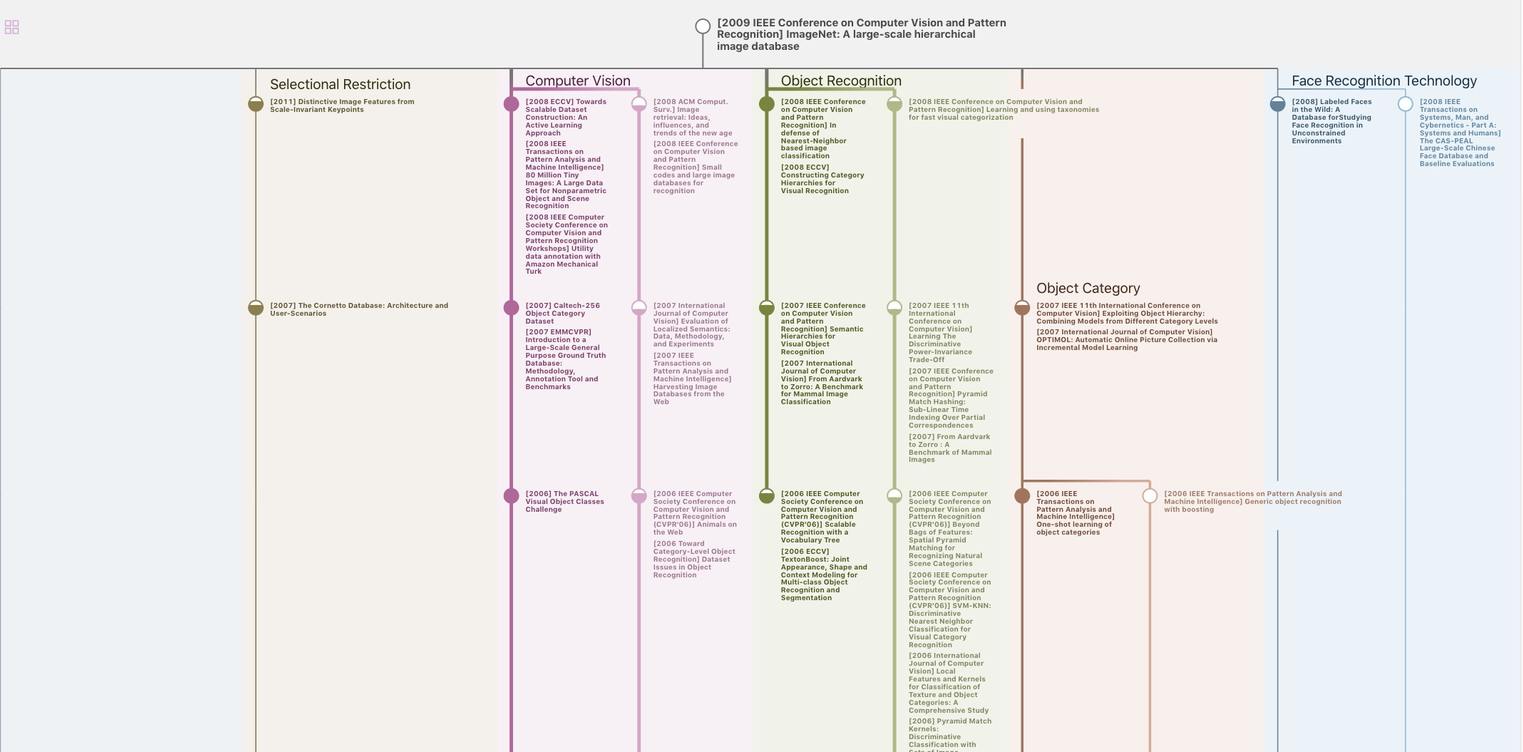
生成溯源树,研究论文发展脉络
Chat Paper
正在生成论文摘要