Online Hybrid Learning Methods for Real-Time Structural Health Monitoring Using Remote Sensing and Small Displacement Data
REMOTE SENSING(2022)
Abstract
Structural health monitoring (SHM) by using remote sensing and synthetic aperture radar (SAR) images is a promising approach to assessing the safety and the integrity of civil structures. Apart from this issue, artificial intelligence and machine learning have brought great opportunities to SHM by learning an automated computational model for damage detection. Accordingly, this article proposes online hybrid learning methods to firstly deal with some major challenges in data-driven SHM and secondly detect damage via small displacement data from SAR images in a real-time manner. The proposed methods contain three main parts: (i) data augmentation by Hamiltonian Monte Carlo and slice sampling for addressing the problem of small displacement data, (ii) data normalization by an online deep transfer learning algorithm for removing the effects of environmental and/or operational variability from augmented data, and (iii) feature classification via a scalar novelty score. The major contributions of this research include proposing two online hybrid unsupervised learning methods and providing effective frameworks for online damage detection. A small set of displacement samples extracted from SAR images of TerraSar-X regarding a long-term monitoring scheme of the Tadcaster Bridge in United Kingdom is applied to validate the proposed methods.
MoreTranslated text
Key words
structural health monitoring,pre-collapse prediction,online learning,remote sensing,small data,bridge
AI Read Science
Must-Reading Tree
Example
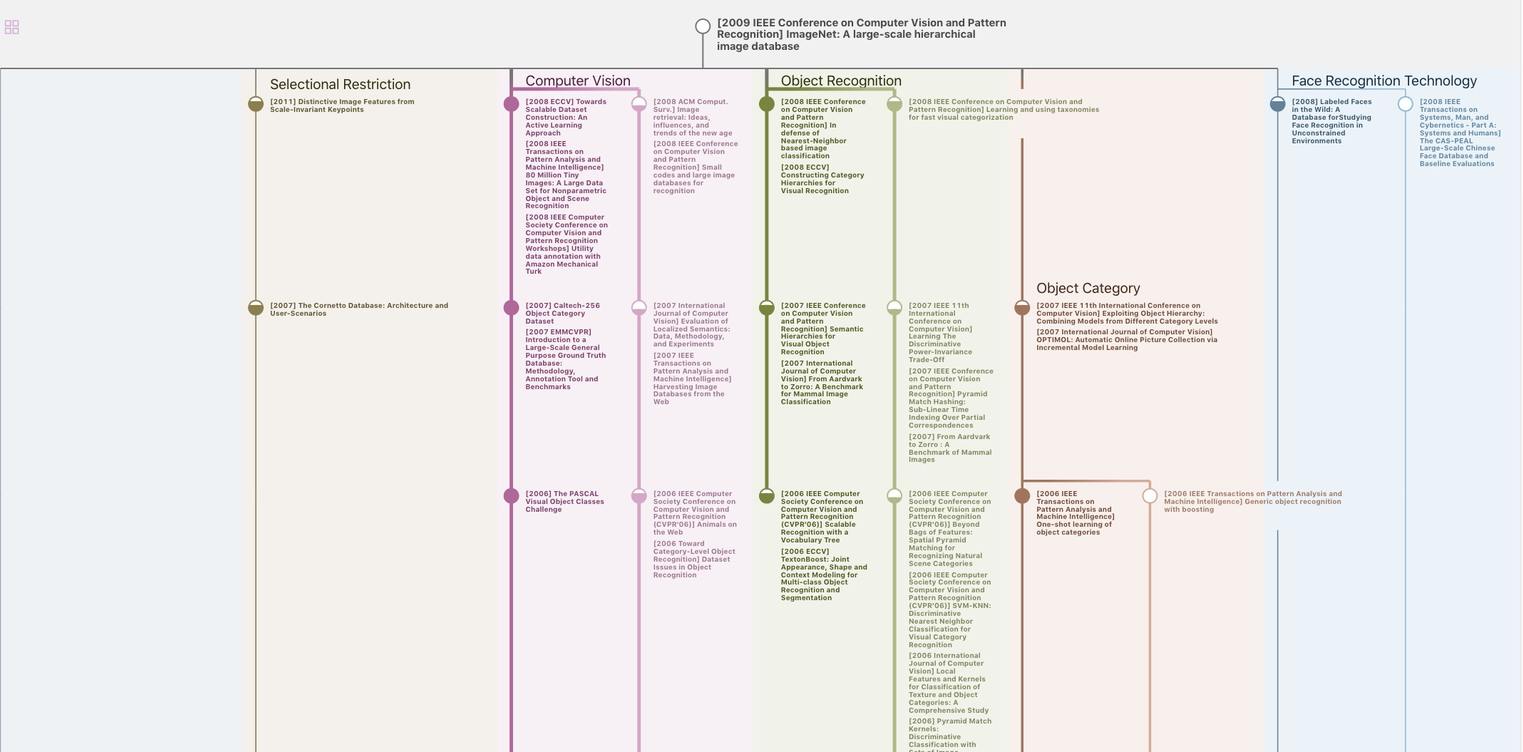
Generate MRT to find the research sequence of this paper
Chat Paper
Summary is being generated by the instructions you defined