Training Socially Engaging Robots: Modeling Backchannel Behaviors with Batch Reinforcement Learning
IEEE Transactions on Affective Computing(2022)
摘要
A key aspect of social human-robot interaction is natural non-verbal communication. In this work, we train an agent with batch reinforcement learning to generate nods and smiles as backchannels in order to increase the naturalness of the interaction and to engage humans. We introduce the Sequential Random Deep Q-Network (SRDQN) method to learn a policy for backchannel generation, that explicitly maximizes user engagement. The proposed SRDQN method outperforms the existing vanilla Q-learning methods when evaluated using off-policy policy evaluation techniques. Furthermore, to verify the effectiveness of SRDQN, a human-robot experiment has been designed and conducted with an expressive 3d robot head. The experiment is based on a story-shaping game designed to create an interactive social activity with the robot. The engagement of the participants during the interaction is computed from user's social signals like backchannels, mutual gaze and adjacency pair. The subjective feedback from participants and the engagement values strongly indicate that our framework is a step forward towards the autonomous learning of a socially acceptable backchanneling behavior.
更多查看译文
关键词
Human-robot interaction,user engagement,backchannels,batch reinforcement learning
AI 理解论文
溯源树
样例
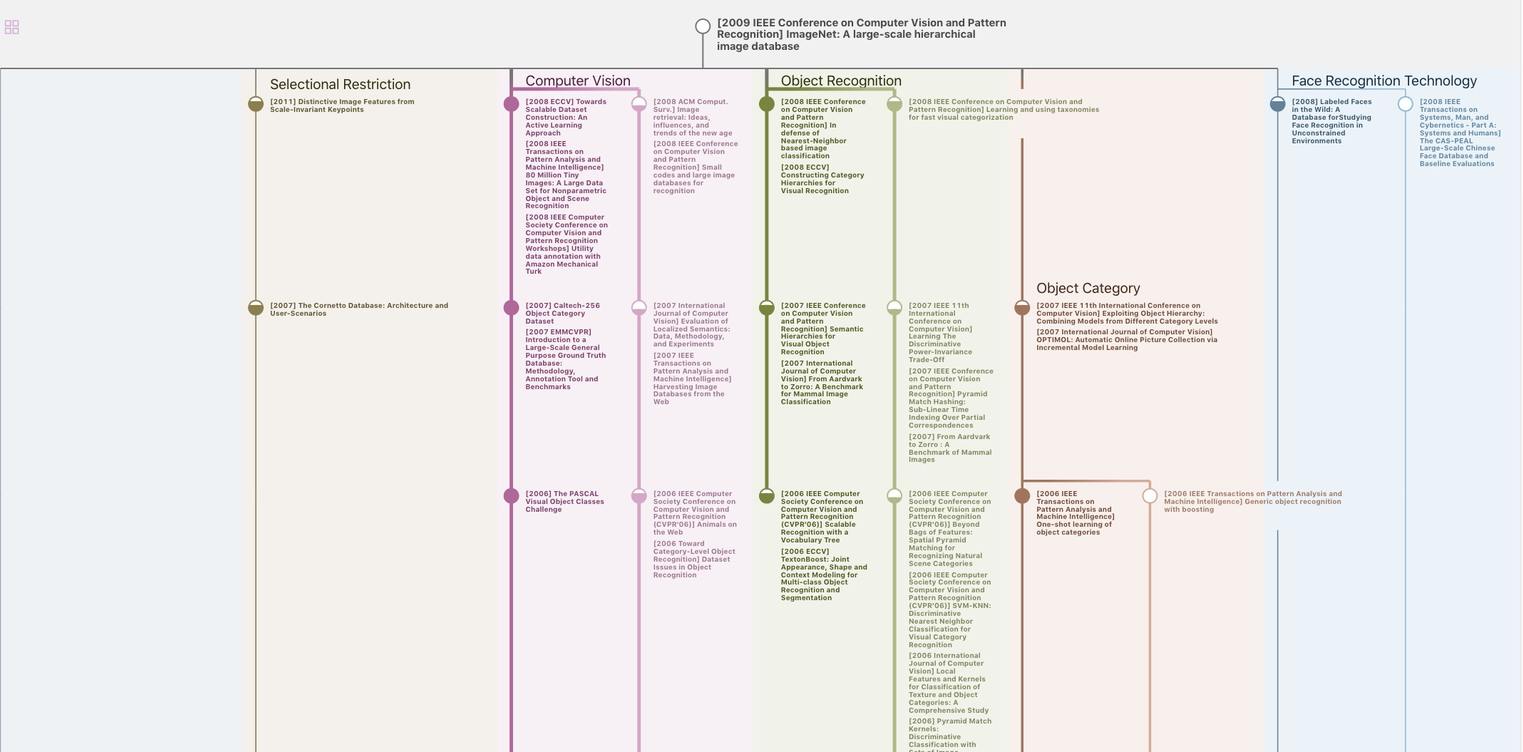
生成溯源树,研究论文发展脉络
Chat Paper
正在生成论文摘要