Deep learning for space-borne focal plane wavefront sensing
Space Telescopes and Instrumentation 2022: Optical, Infrared, and Millimeter Wave(2022)
摘要
For space-based Earth Observations and solar system observations, obtaining both high revisit rates (using a constellation of small platforms) and high angular resolution (using large optics and therefore a large platform) is an asset for many applications. Unfortunately, they prevent the occurrence of each other. A deployable satellite concept has been suggested that could grant both assets by producing jointly high revisit rates and high angular resolution of roughly 1 meter on the ground. This concept relies however on the capacity to maintain the phasing of the segments at a sufficient precision (a few tens of nanometers at visible wavelengths), while undergoing strong and dynamic thermal gradients. In the constrained volume environment of a CubeSat, the system must reuse the scientific images to measure the phasing errors. We address in this paper the key issue of focal-plane wave-front sensing for a segmented pupil using a single image with deep learning. We show a first demonstration of measurement on a point source. The neural network is able to identify properly the phase piston-tip-tilt coefficients below the limit of 15nm per petal.
更多查看译文
关键词
Wavefront sensing,Focal plane wavefront sensing,Deep learning,Neural Network,Artificial Intelligence,Astronomy,Nanosatellite,CubeSat
AI 理解论文
溯源树
样例
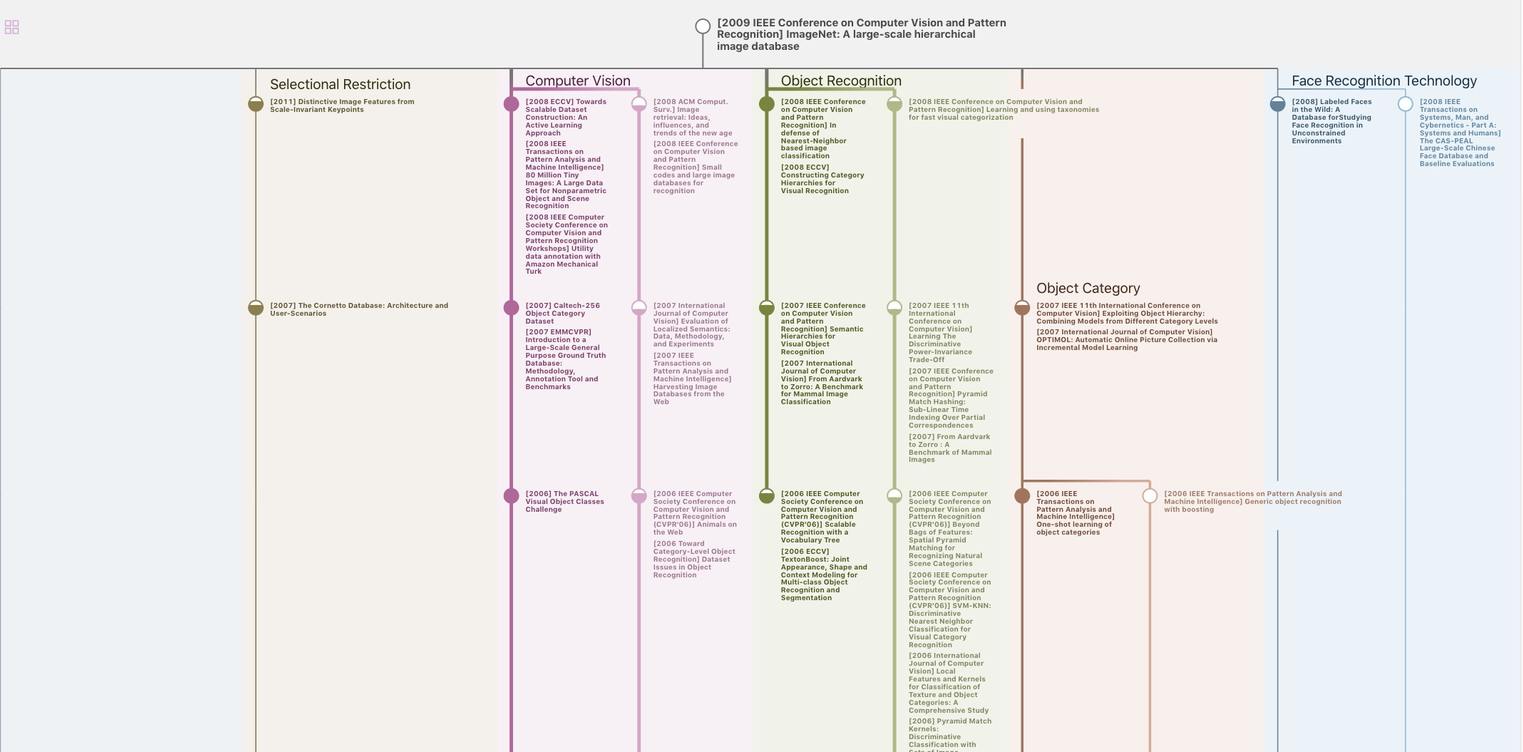
生成溯源树,研究论文发展脉络
Chat Paper
正在生成论文摘要