Polarized self-attention: Towards high-quality pixel-wise mapping
Neurocomputing(2022)
摘要
We address the pixel-wise mapping problem that commonly exists in the fine-grained computer vision tasks, such as estimating keypoint heatmaps and segmentation masks. These tasks require, at low computation overheads, modeling the long-range dependencies among high-resolution inputs and estimating the highly nonlinear pixel-wise outputs. While the attention mechanism added to Deep Convolutional Neural Networks (DCNNs) can boost long-range dependencies, the element-specific attention, such as the Nonlocal block, is highly complex and noise-sensitive to learn, and most of the simplified attention blocks are designed for image-wise classification purposes and simply applied to pixel-wise tasks. In this paper, we present the Polarized Self-Attention (PSA) block targeting the high-quality pixel-wise mapping with: (1) Polarized filtering: keeping high internal resolution in both channel and spatial attention computation while completely collapsing input tensors along their counterpart dimensions. (2) Enhancement: composing non-linearity that directly fits the output distribution of typical pixel-wise mappings, such as the 2D Gaussian distribution (keypoint heatmaps), or the 2D Binormial distribution (binary segmentation masks). Experimental results show that PSA boosts standard baselines by 2–4 points, and boosts state-of-the-arts by 1–2 points on 2D pose estimation and semantic segmentation benchmarks. Codes are available at ( https://github.com/DeLightCMU/PSA).
更多查看译文
关键词
Pixel-wise mapping,Self-attention,Polarization,Convolution
AI 理解论文
溯源树
样例
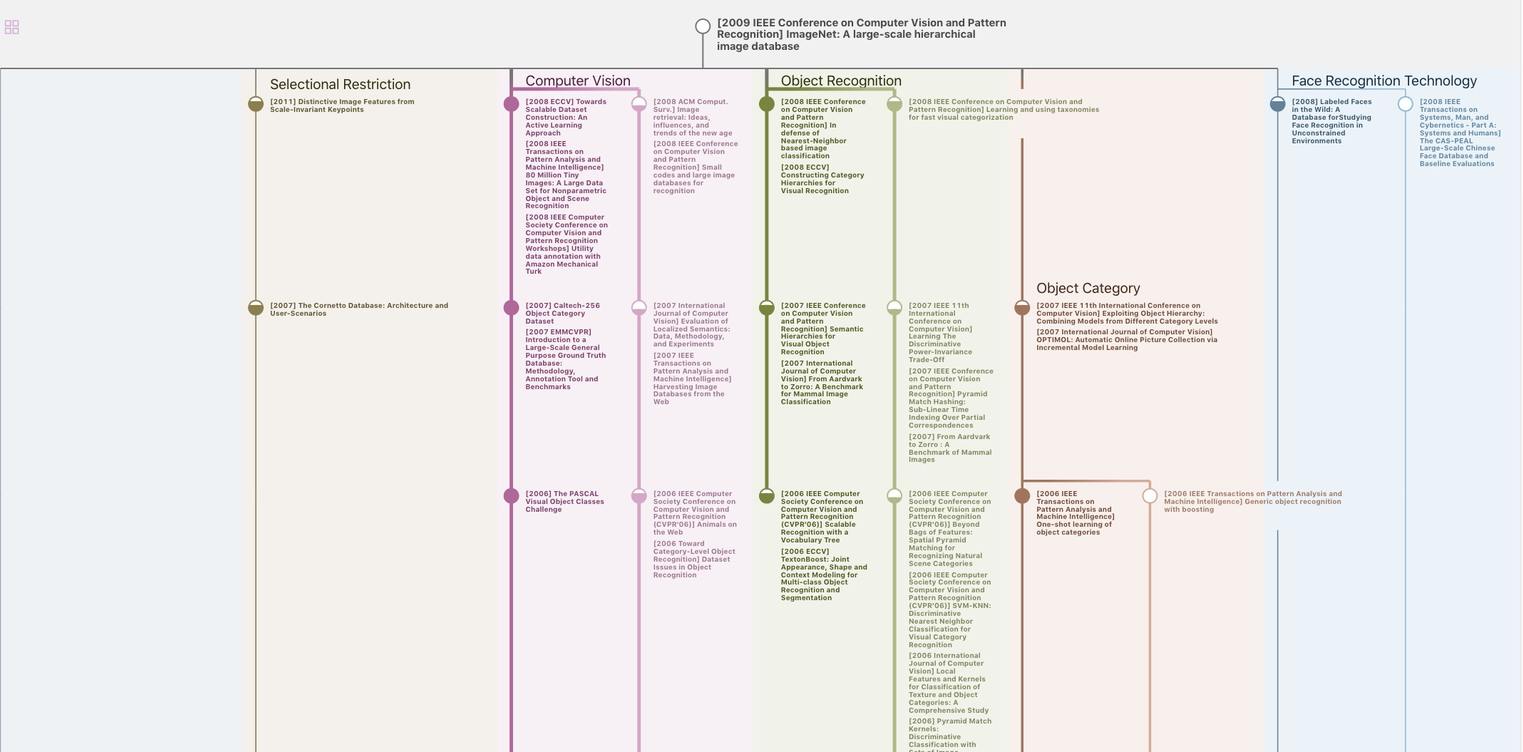
生成溯源树,研究论文发展脉络
Chat Paper
正在生成论文摘要