Group Sparse-based Discriminative Feature Learning for Face Recognition
2022 4th International Conference on Image Processing and Machine Vision (IPMV)(2022)
摘要
The rapid development of facial recognition technology has brought great convenience to daily life, but also serious security risks, especially in the case of occlusion and loud noise. Faced with this limitation, this letter proposes a fast face recognition framework called a Group Sparse-based Discriminative Feature Learning (GSDFL-Net). Specifically, GSDFL-Net uses a novel unified objective function to simultaneously learn the discriminant features, sparse code and classification errors. In the proposed framework, the feature projection is incorporated into GSDFL-Net model, which reduces the classification errors. Then, we integrate denoising FFDNet into the proposed GS FL-Net model to penalize the noisy pixels, which is simultaneously learned by our unified objective function. Besides, we derive an optimization mechanism to encourage obtained learning parameters and decrease the information loss. Extensive experiments demonstrate the effectiveness of the proposed scheme under different including occlusion random noise conditions on the famous Aleix Martinez and ExYale B database.
更多查看译文
AI 理解论文
溯源树
样例
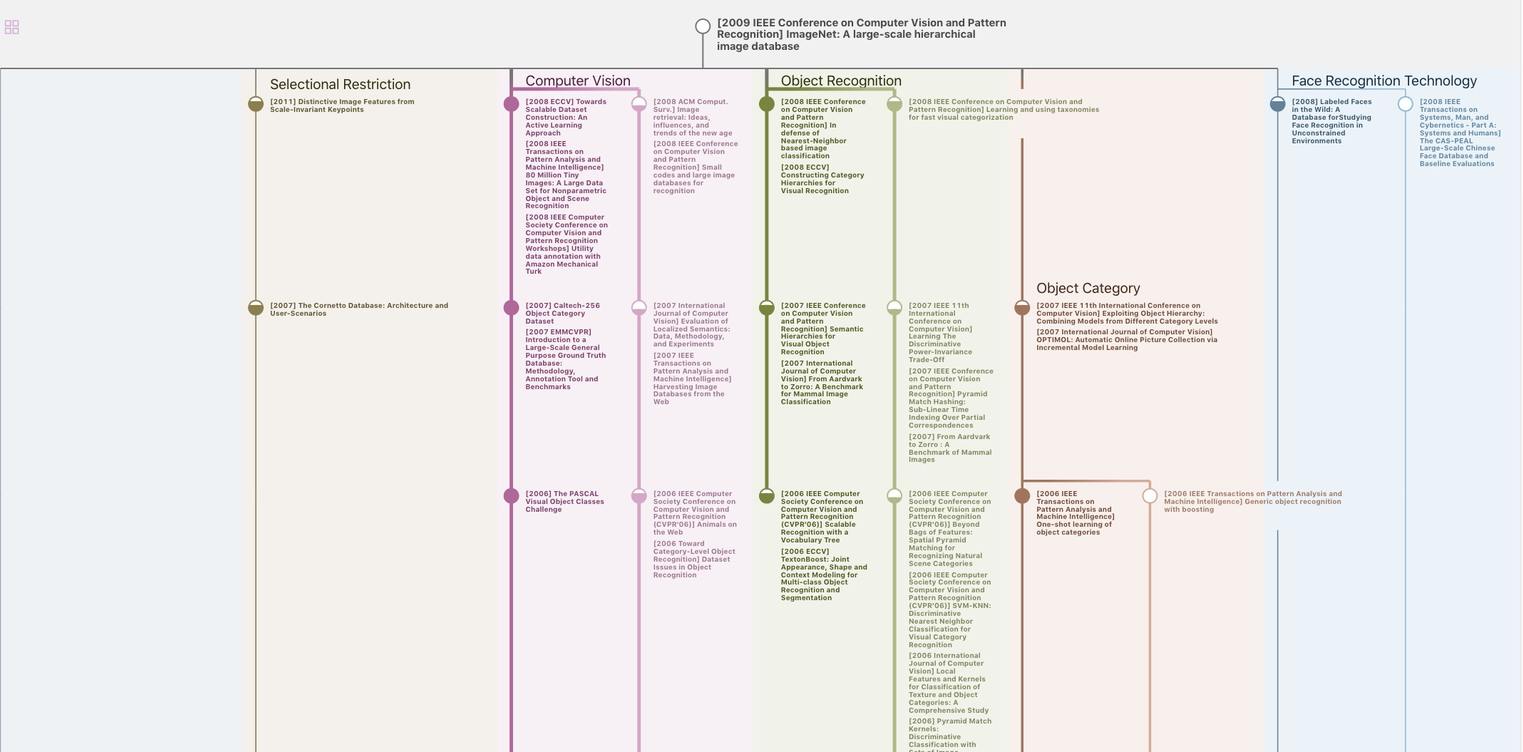
生成溯源树,研究论文发展脉络
Chat Paper
正在生成论文摘要