A Fast Method for Whole Liver- and Colorectal Liver Metastasis Segmentations from MRI Using 3D FCNN Networks
APPLIED SCIENCES-BASEL(2022)
摘要
The liver is the most frequent organ for metastasis from colorectal cancer, one of the most common tumor types with a poor prognosis. Despite reducing surgical planning time and providing better spatial representation, current methods of 3D modeling of patient-specific liver anatomy are extremely time-consuming. The purpose of this study was to develop a deep learning model trained on an in-house dataset of 84 MRI volumes to rapidly provide fully automated whole liver and liver lesions segmentation from volumetric MRI series. A cascade approach was utilized to address the problem of class imbalance. The trained model achieved an average Dice score for whole liver segmentation of 0.944 +/- 0.009 and 0.780 +/- 0.119 for liver lesion segmentation. Furthermore, applying this method to a not-annotated dataset creates a complete 3D segmentation in less than 6 s per MRI volume, with a mean segmentation Dice score of 0.994 +/- 0.003 for the liver and 0.709 +/- 0.171 for tumors compared to manual corrections applied after the inference was achieved. Availability and integration of our method in clinical practice may improve diagnosis and treatment planning in patients with colorectal liver metastasis and open new possibilities for research into liver tumors.
更多查看译文
关键词
deep learning, HighResNet, cascade DL, 3D model, magnetic resonance, hepatic tumor
AI 理解论文
溯源树
样例
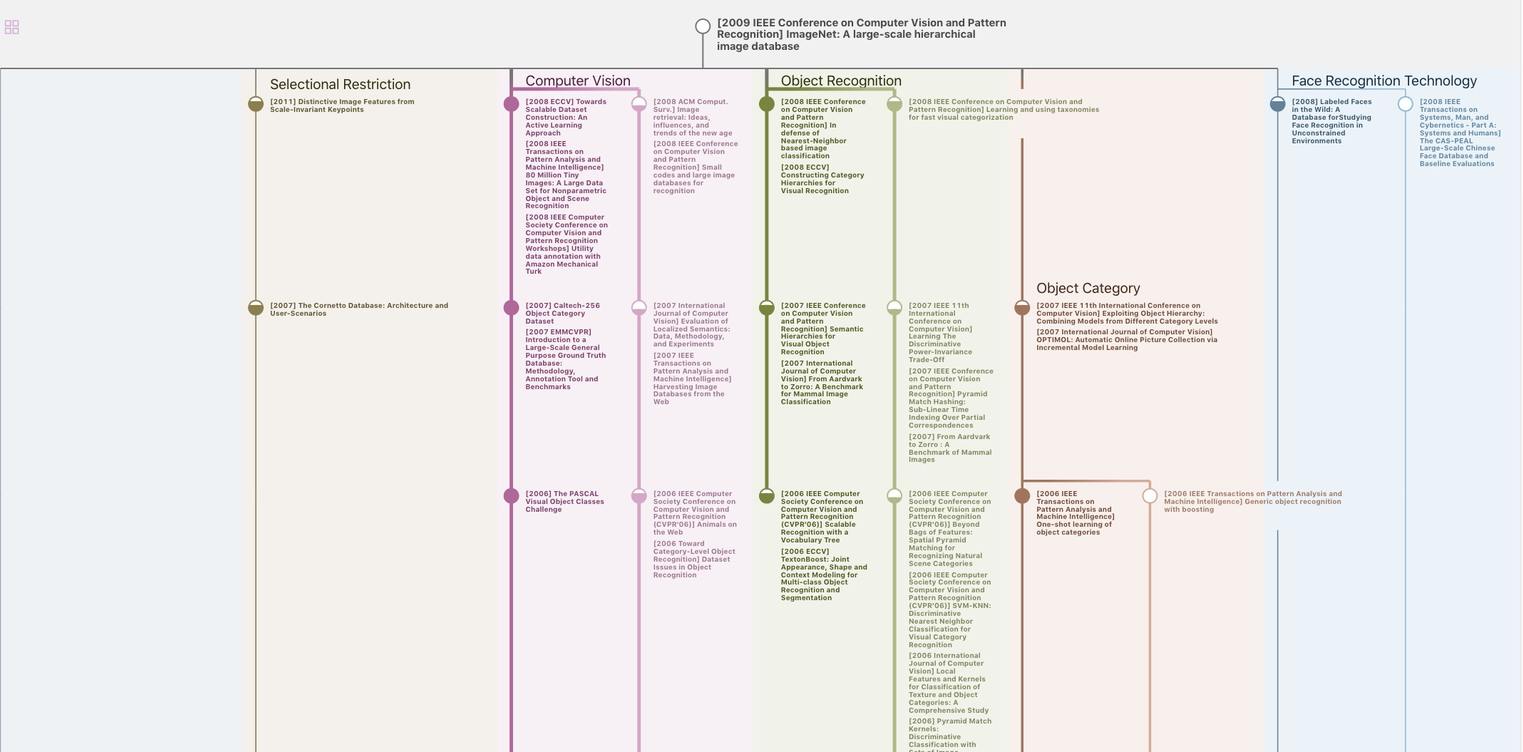
生成溯源树,研究论文发展脉络
Chat Paper
正在生成论文摘要