A transfer learning SHM strategy for bridges enriched by the use of speaker recognition x-vectors
JOURNAL OF CIVIL STRUCTURAL HEALTH MONITORING(2022)
摘要
The aim of vibration-based Structural Health Monitoring (SHM) is to strategize on how to monitor the health condition of a structure accurately and be able to rapidly and robustly detect the occurrence of structural damage by observing changes in the structural behavior. The dynamic traits of a structural system are represented by features extracted from its vibration response. Modal characteristics and indexes related to structural stiffness are popular in SHM; however, they sometimes happen to be misleading in pointing out the integrity status of the structure since the fluctuations in environmental and operational conditions highly influence them in addition to occurring damage and deterioration. Moreover, they are often characterized by complex and time-consuming extraction processes. Therefore, recent studies looked for alternative parameters to be used as damage sensitive features. In this context, the cepstral features initially introduced for speaker and speech recognition purposes showed to be highly promising and optimal candidates for damage detection in civil applications. Nowadays, SHM strategies relying on Artificial Neural Network tools to delineate models that mimic the dynamic behavior of structural systems are viral. However, a fundamental issue in developing these strategies for civil applications is caused by the imbalanced nature of the available databases for civil applications, which commonly do not contain sufficient information from all the different classes that need to be identified. Unfortunately, when the aim is to classify between the healthy and damaged conditions in a structure, it is extremely rare to have sufficient data for the unhealthy state since the system has already failed. Concurrently, it is common to have plenty of data coming from the structure under operational conditions. Consequently, the learning task, carried on with deep learning approaches, becomes case-dependent and tends to be specialized for a particular structure and a very limited number of damage scenarios. This work develops a vibration-based SHM framework for damage classification in structural systems to overcome such a limitation. The model is trained to gain richness and knowledge in the learning task from a source domain with extensive and exhaustive datasets and transfer that knowledge to a target domain with much less information. In particular, the proposed procedure relies on creating a model that will learn the lower-level features characterizing vibration records from the rich audio dataset and then specialize its knowledge on the chosen structural dataset. The goal is to enrich the model’s ability to discriminate between classes on the audio records, presenting multiple different categories with more information to learn. Furthermore, using Mel-Frequency Cepstral Coefficients as damage sensitive features in structural damage assessment facilitates the transition and dialogue between the audio and structural domains. The framework is tested using the test field data of the established and popular experimental dataset of the Z24 bridge, training the initial part of the network architecture over a different source data, the VoxCeleb dataset.
更多查看译文
关键词
Transfer learning,Structural health monitoring,Mel-frequency cepstral coefficients,X-vector features,Bridges
AI 理解论文
溯源树
样例
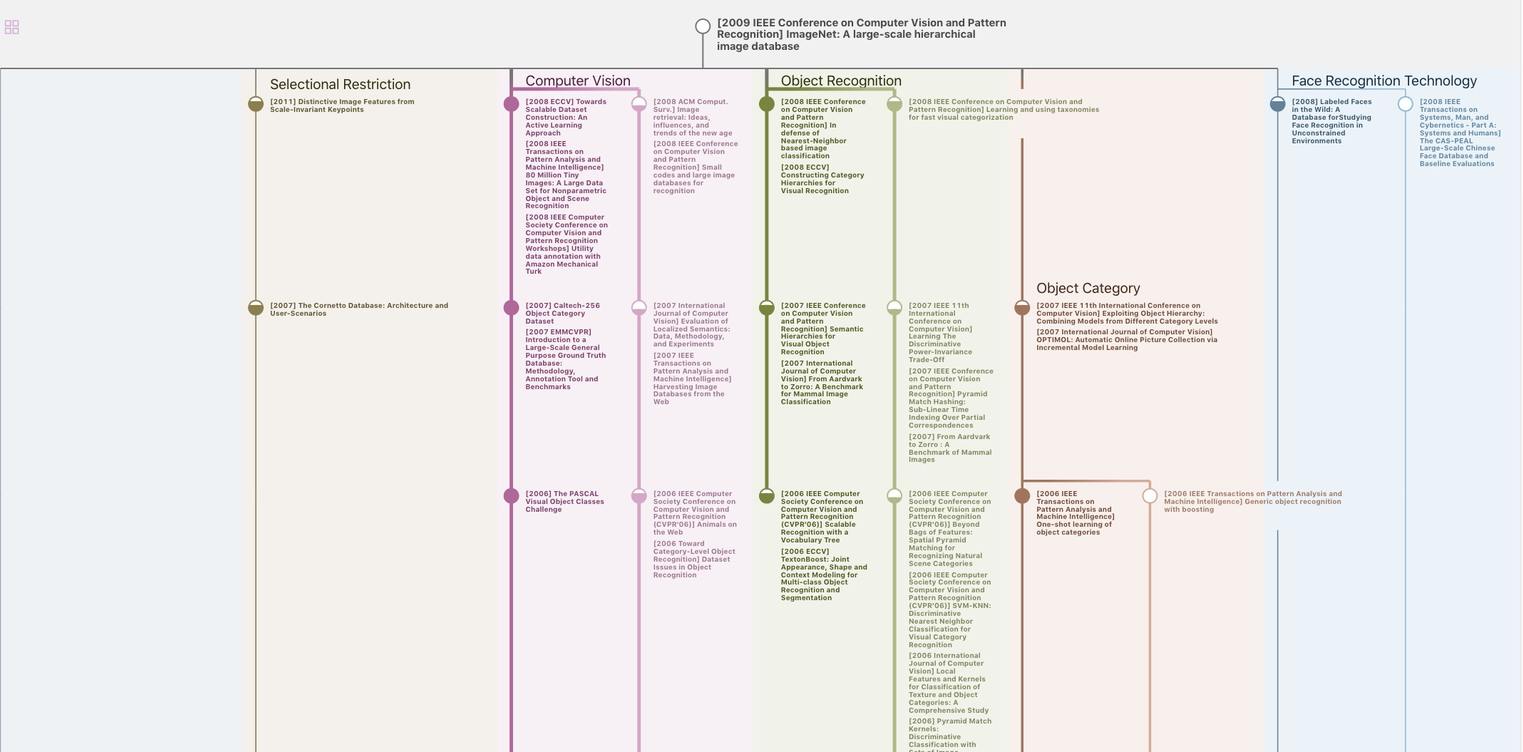
生成溯源树,研究论文发展脉络
Chat Paper
正在生成论文摘要