SPR-SMN: scientific paper recommendation employing SPECTER with memory network
SCIENTOMETRICS(2022)
摘要
During the last decades, recommender systems are becoming quite popular since they provide great assistance to users on social networks and library websites. Unfortunately, the large volume of data combined with sparsity makes personalization a difficult task. In this regard, several models were introduced in the literature that suffers from the cold-start problem and the lack of personalization. In particular, the majority of these models ignore the relationship between the important factors and the semantic relations among the nodes (the authors, and the field of study) on the heterogeneous papers networks. Moreover, they fail to effectively capture researchers’ preferences, which leads to inadequate recommendations. To overcome these problems, with this study we propose a scientific paper recommendation model called SPR-SMN, which employs the SPECTER document embedding model to learn context-preserving paper content representations. The model captures the long-range dependencies and researchers’ preferences, by employing an end-to-end memory network and personalization module, respectively. We experimentally evaluate our method against baseline algorithms over two real-life datasets. The results indicate that the proposed method outperforms competing models.
更多查看译文
关键词
Recommender systems, Paper recommendation, Memory network, SPECTER, Cold-start problem, Personalization
AI 理解论文
溯源树
样例
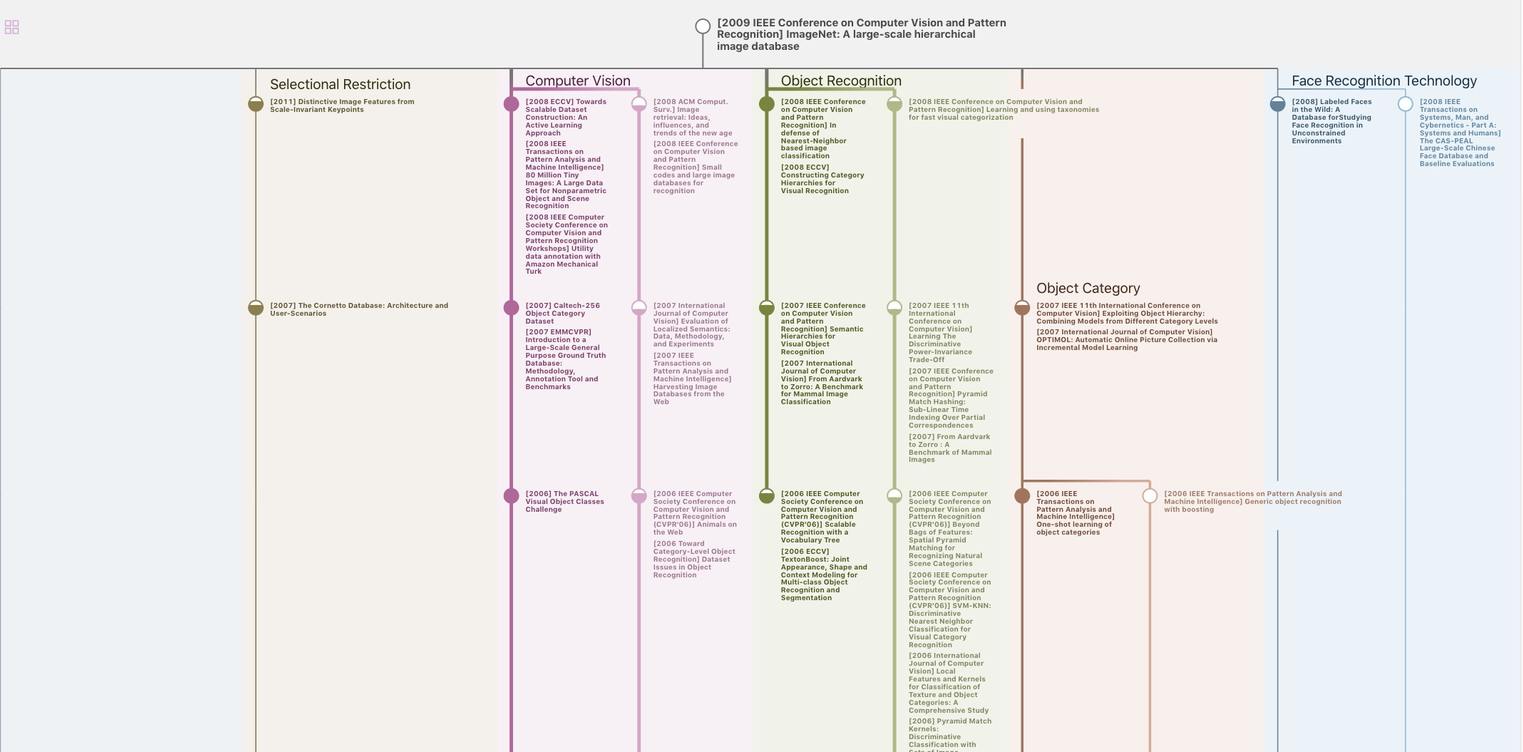
生成溯源树,研究论文发展脉络
Chat Paper
正在生成论文摘要