Spatial-temporal correlated graph neural networks based on neighborhood feature selection for traffic data prediction
Applied Intelligence(2022)
摘要
Prediction as a new frontier has begun to come into focus which has been widely used in traffic management and statistics. Because of traffic time series data possesses both spatial and temporal characteristics, existing models have their limitations in modeling time-space. Most methods utilize two different parts to capture the characteristics of time and space respectively and ignore the potential relationship between them, which does not meet actual traffic conditions in real world. Even if a few models take this correlation into account, they capture a tiny part of temporal characteristics to describe this correlation. These models just extract local features based on time dimensions and cannot capture full-scale temporal characteristics and spatial-temporal heterogeneity. Besides, most methods overlook the over-fitting of feature in aggregation operation by using graph neural networks to model time-space and predict. To settle these problems, this paper proposes a novel spatial-temporal corelated graph neural network (STCGNN) of data forecasting. This model can extract the full-scale characteristics of intricate spatial-temporal graph and can generate spatial-temporal adjacent correlated matrix based on whole characteristics of time series. Furthermore, this model can determine the aggregation degree of feature nodes by Neighborhood Feature Selection Network (NFSN) to avoid the over-fitting of feature. Various experiments are conducted on three real-world datasets and evaluation metrics are 15.81 of MAE, 14.59% of MAPE and 27.23 of RMSE on PEMS03 dataset. These results have demonstrated that our model STCGNN obtains the lowest errors in predicting than previous models.
更多查看译文
关键词
Spatial-temporal prediction, Graph neural network, Time series data
AI 理解论文
溯源树
样例
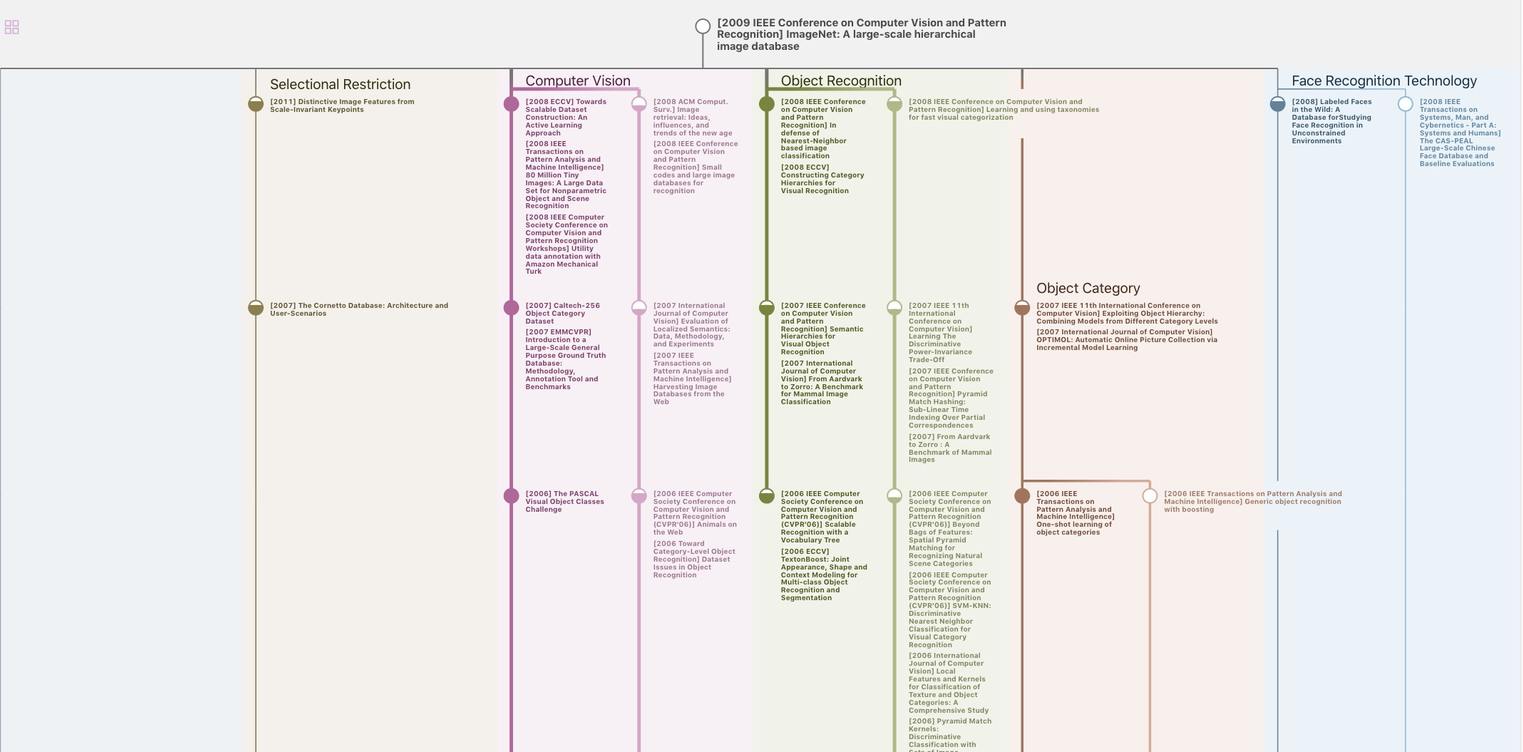
生成溯源树,研究论文发展脉络
Chat Paper
正在生成论文摘要