Evaluation of rapeseed flowering dynamics for different genotypes with UAV platform and machine learning algorithm
Precision Agriculture(2022)
Abstract
Rapeseed ( Brassica napus L.) is an important oil-bearing cash crop. Effective identification of the rapeseed flowering date is important for yield estimation and disease control. Traditional field measurements of rapeseed flowering are time-consuming, labour-intensive and strongly subjective. In this study, red, green and blue (RGB) images of rapeseed flowering derived from unmanned aerial vehicles (UAVs) were acquired with a total of seventeen available orthomosaic images, covering the whole flowering period for 299 rapeseed varieties. Five different machine learning methods were employed to identify and to extract the flowering areas in each plot. The results suggested that the accuracy of flowering area extraction by the decision tree-based segmentation model (DTSM) was higher than that of naive Bayes, K-nearest neighbours (KNN), random forest (RF) and support vector machine (SVM) in all varieties and flowering dates, with R 2 = 0.97 and root mean square error (RMSE) = 0.051 pixels/pixels. Data on the proportion of flowering area and its dynamics showed differences in the time and duration of each flowering date among varieties. All varieties were classified into four clusters based on k-means clustering analysis. There were significant differences in eight phenotypic parameters among the four clusters, especially in the time of maximum flowering ratio and the time entering the early and medium flowering dates. The results from this study could provide a basis for rapeseed breeding based on flowering dynamics.
MoreTranslated text
Key words
Brassica napus L., Flowering date, Machine learning algorithm, Clustering analysis, Variety classification
AI Read Science
Must-Reading Tree
Example
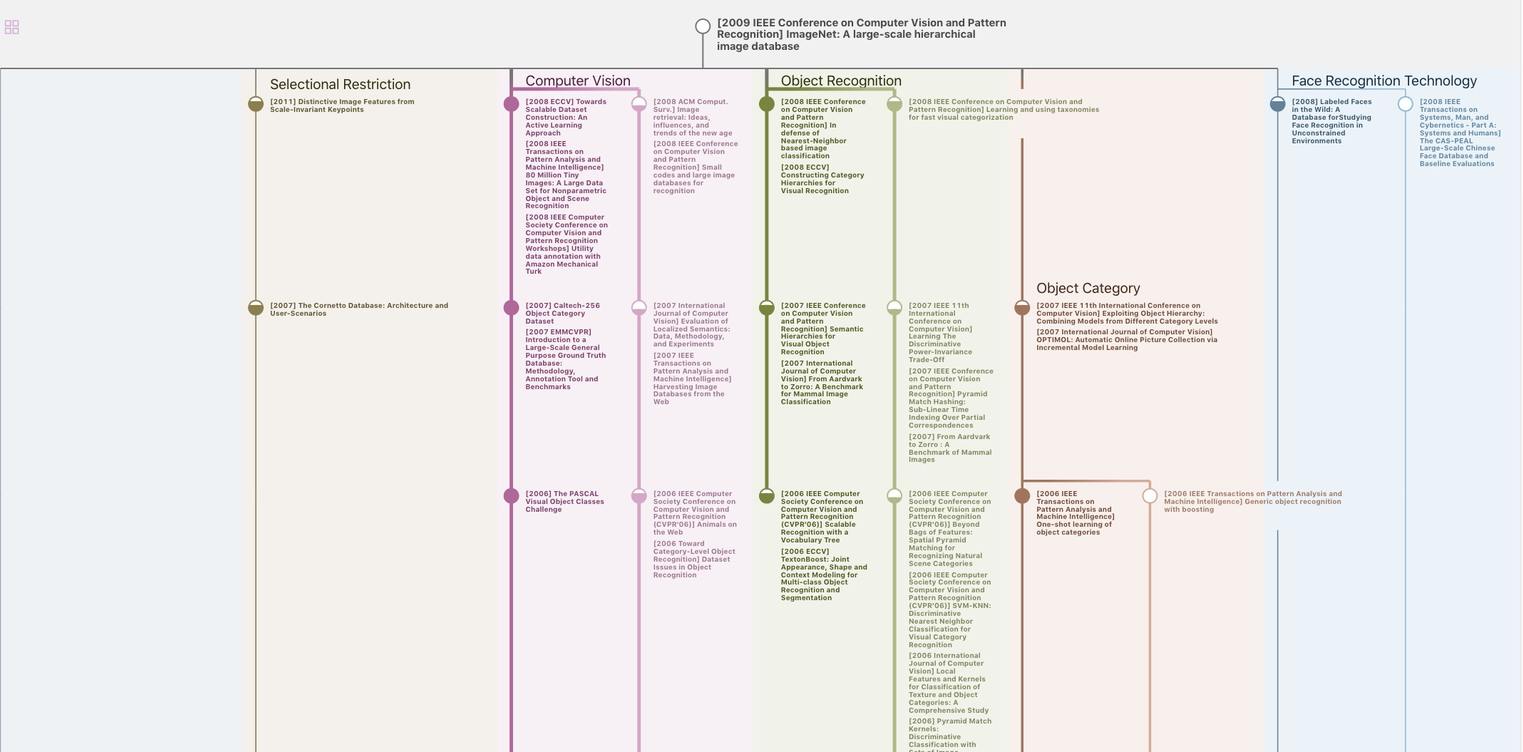
Generate MRT to find the research sequence of this paper
Chat Paper
Summary is being generated by the instructions you defined