Stochastic Gradient Hamiltonian Monte Carlo for non-convex learning
Stochastic Processes and their Applications(2022)
摘要
Stochastic Gradient Hamiltonian Monte Carlo (SGHMC) is a momentum version of stochastic gradient descent with properly injected Gaussian noise to find a global minimum. In this paper, non-asymptotic convergence analysis of SGHMC is given in the context of non-convex optimization, where subsampling techniques are used over an i.i.d. dataset for gradient updates. In contrast to Raginsky et al. (2017) and Gao et al. (2021), our results are sharper in terms of step size, variance, and independent from the number of iterations.
更多查看译文
关键词
learning,gradient,non-convex
AI 理解论文
溯源树
样例
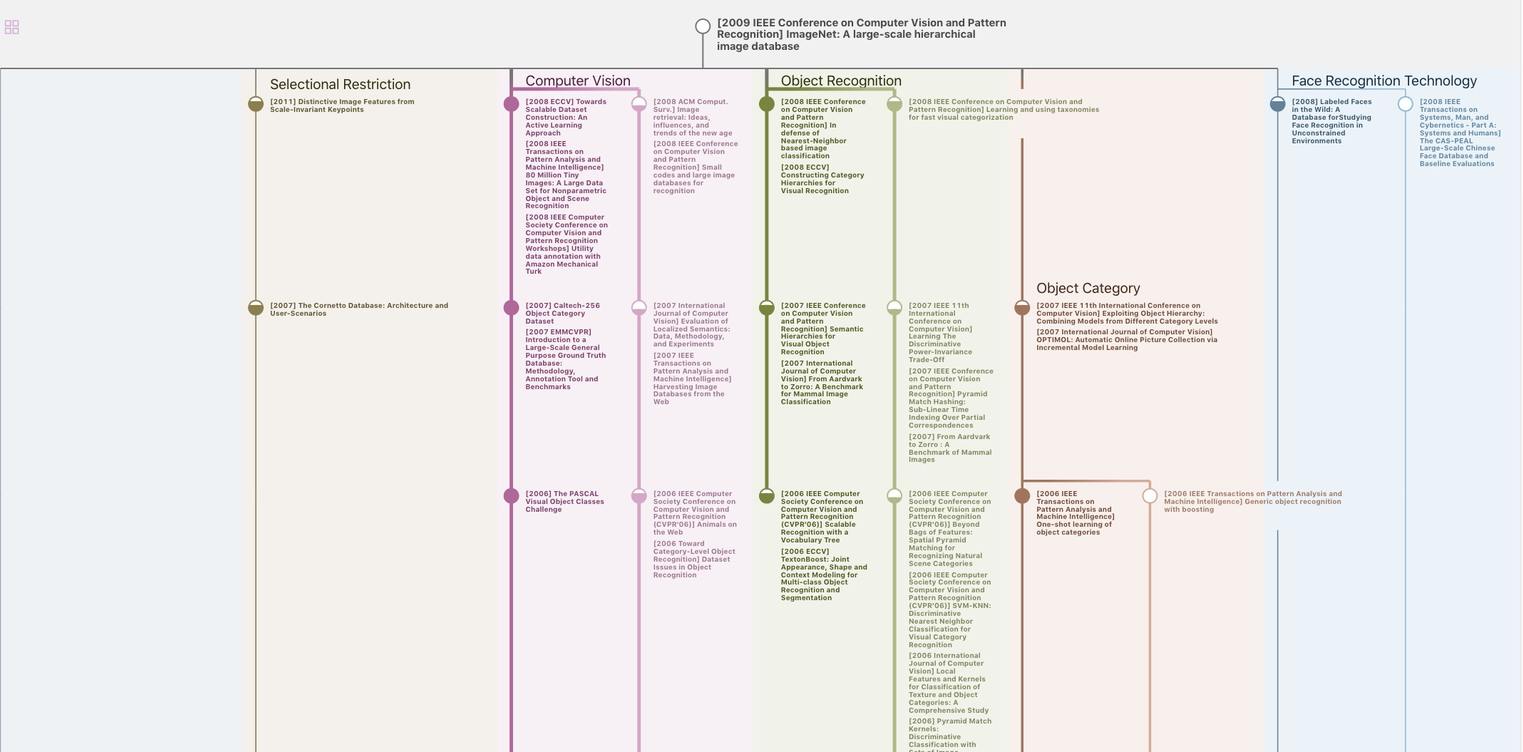
生成溯源树,研究论文发展脉络
Chat Paper
正在生成论文摘要