Short-Term Load Forecasting Using Channel and Temporal Attention Based Temporal Convolutional Network
ELECTRIC POWER SYSTEMS RESEARCH(2022)
摘要
Load forecasting is the foundation of power system operation and planning. Accurate load forecasting can secure the safe and reliable operation of the power system, cut power generation costs, and increase economic benefits. However, it is challenging to effectively forecast load in view of the complicated effects due to a variety of factors involved. In order to enhance the forecast performance, this paper proposes a short-term load forecasting model based on Temporal Convolutional Network (TCN) with channel and temporal attention mechanism (AM), which fully exploits the non-linear relationship between meteorological factors and load. In addition, the Maximum Information Coefficient (MIC) is adopted to select high-quality input variables and eliminate irrelevant variables to reduce the parameters that the model needs to train. Meanwhile, Fuzzy c-means (FCM) combined with Dynamic Time Warping (DTW) is developed to conduct cluster analysis on load data to classify loads with high similar characteristics into the same cluster. Experiments were performed on two different datasets come from public and power grid company to verify the capabilities of the proposed forecasting approach. The experimental results show that the put forward method can effectively enhance the accuracy and generalization capability of load forecasting compared with other forecasting models.
更多查看译文
关键词
temporal convolutional network, attention mechanism, maximum information coefficient, fuzzy c-means, short-term load forecasting
AI 理解论文
溯源树
样例
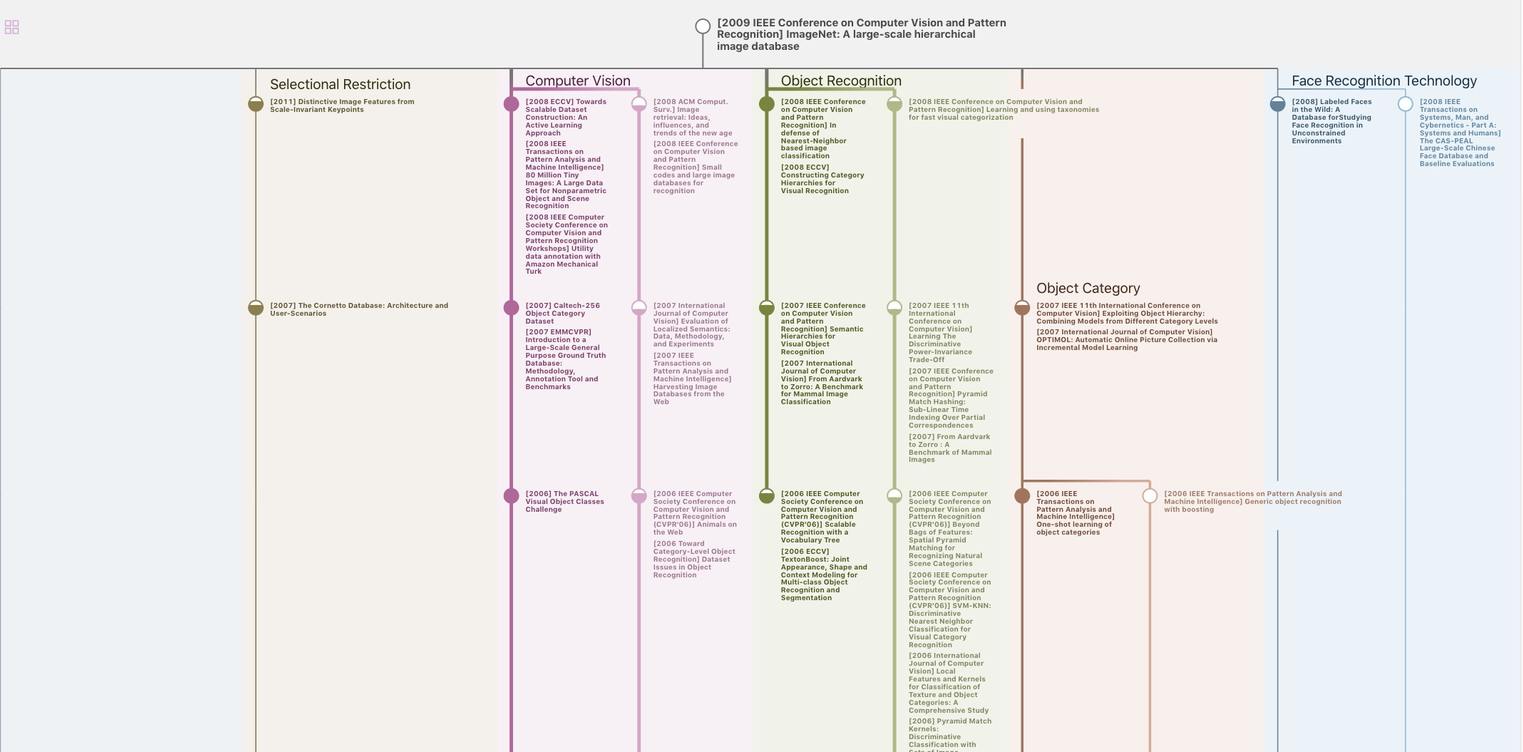
生成溯源树,研究论文发展脉络
Chat Paper
正在生成论文摘要