Hardware-Assisted Machine Learning in Resource-Constrained IoT Environments for Security: Review and Future Prospective
IEEE ACCESS(2022)
摘要
As the Internet of Things (IoT) technology advances, billions of multidisciplinary smart devices act in concert, rarely requiring human intervention, posing significant challenges in supporting trusted computing and user privacy, as well as protecting against attacks such as spoofing, denial of service (DoS), jamming, and eavesdropping. To tackle attacks on the IoT and cyber-physical ecosystem, many intrusion detection and security approaches have been presented in the literature. Machine learning (ML) based intrusion and anomaly detection has lately gained traction due to its capacity to cope with encrypted and rapidly developing threat techniques. This work investigates into machine learning (ML) and deep learning (DL) methodologies for IoT device security and examine the benefits, drawbacks, and potential. To protect an IoT infrastructure, various solutions look into hardware-based methods for ML-based IoT authentication, access control, secure offloading, and malware detection schemes. This review aims to illuminate the value of various approaches for addressing IoT security in a truly effective, flexible, and seamless manner, as well as to provide answers to questions about tradeoffs in integrating accelerators and customizing embedded device architectures for effective use of ML-based methods.
更多查看译文
关键词
Internet of Things, Security, Machine learning, Hardware, Malware, Microprogramming, Cryptography, AI-based IoT security, hardware-based machine learning, IoT intrusion detection, trusted embedded devices
AI 理解论文
溯源树
样例
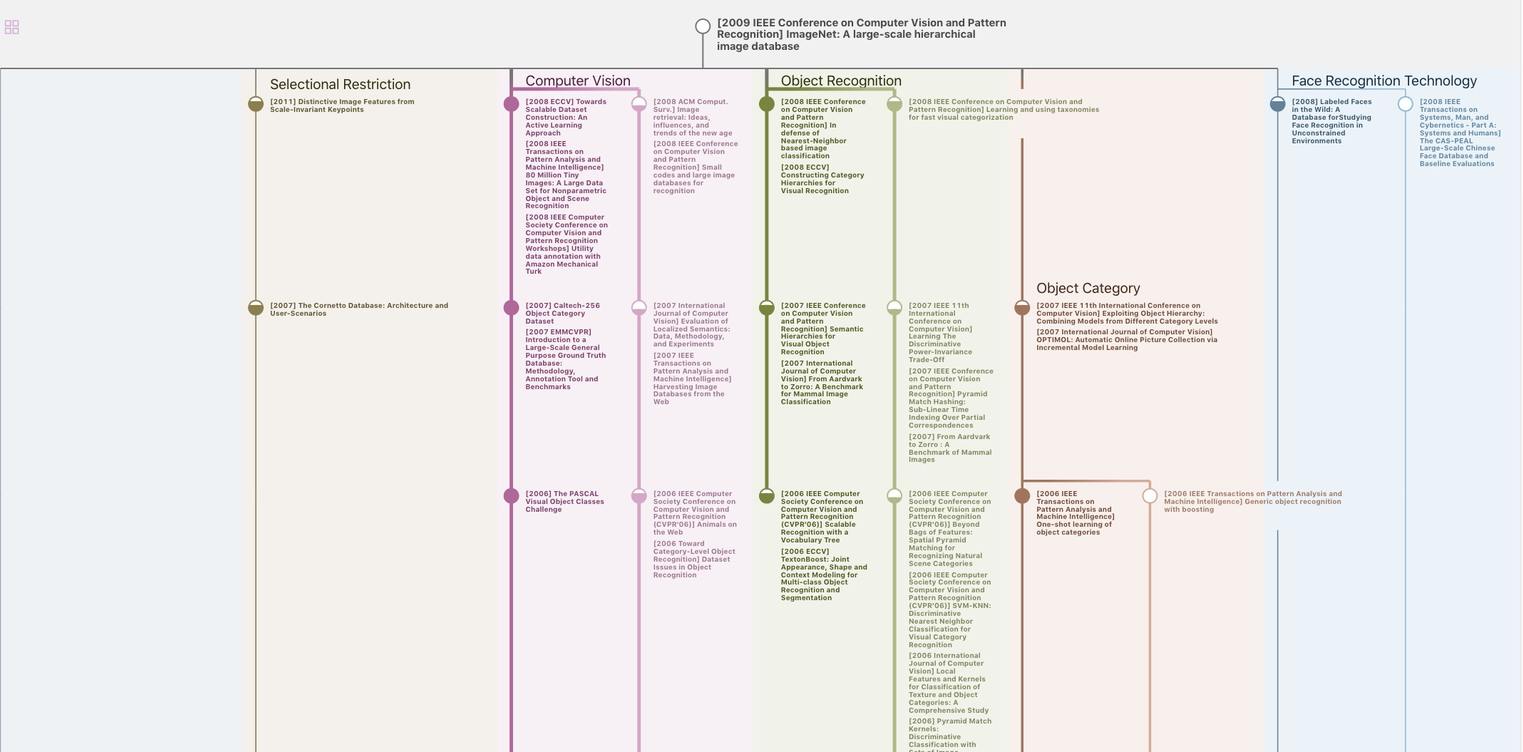
生成溯源树,研究论文发展脉络
Chat Paper
正在生成论文摘要