Example Forgetting: A Novel Approach to Explain and Interpret Deep Neural Networks in Seismic Interpretation
IEEE TRANSACTIONS ON GEOSCIENCE AND REMOTE SENSING(2022)
摘要
In recent years, deep neural networks have significantly impacted the seismic interpretation process. Due to the simple implementation and low interpretation costs, deep neural networks are an attractive component for the common interpretation pipeline. However, neural networks are frequently met with distrust due to their property of producing semantically incorrect outputs when exposed to sections the model was not trained on. We address this issue by explaining model behavior and improving generalization properties through example forgetting. First, we introduce a method that effectively relates semantically malfunctioned predictions to their respectful positions within the neural network representation manifold. More concrete, our method tracks how models "forget" seismic reflections during training and establishes a connection to the decision boundary proximity of the target class. Second, we use our analysis technique to identify frequently forgotten regions within the training volume and augment the training set with state-of-the-art style transfer techniques from computer vision. We show that our method improves the segmentation performance on underrepresented classes while significantly reducing the forgotten regions in the F3 volume in The Netherlands.
更多查看译文
关键词
Training, Computational modeling, Heating systems, Neural networks, Deep learning, Predictive models, Feature extraction, Deep learning, example forgetting, seismic interpretation, semantic segmentation
AI 理解论文
溯源树
样例
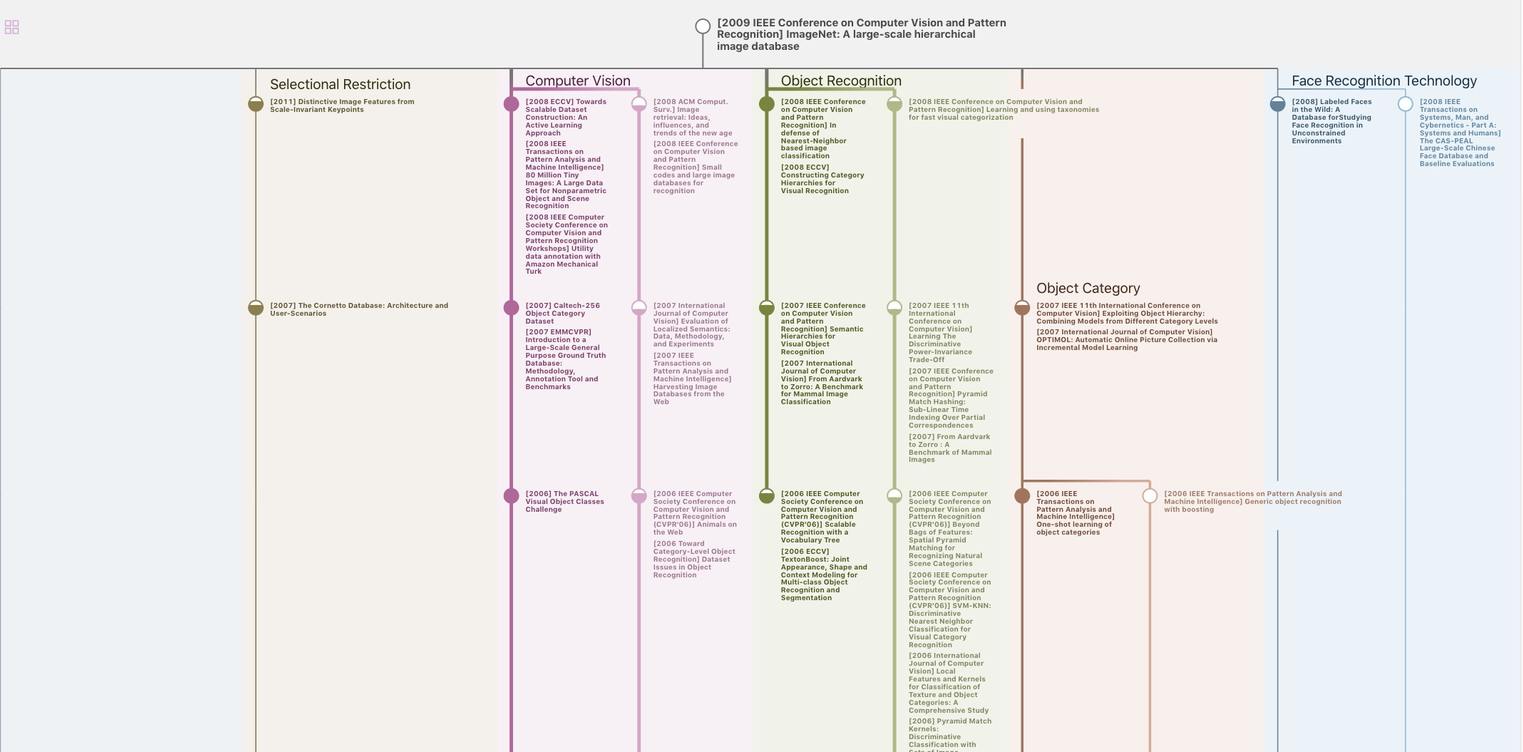
生成溯源树,研究论文发展脉络
Chat Paper
正在生成论文摘要