Adaptive similarity search for the retrieval of rare events from large time series databases
Advanced Engineering Informatics(2022)
摘要
Improving the recall of information retrieval systems for similarity search in time series databases is of great practical importance. In the manufacturing domain, these systems are used to query large databases of manufacturing process data that contain terabytes of time series data from millions of parts. This allows domain experts to identify parts that exhibit specific process faults. In practice, the search often amounts to an iterative query–response cycle in which users define new queries (time series patterns) based on results of previous queries. This is a well-documented phenomenon in information retrieval and not unique to the manufacturing domain. Indexing manufacturing databases to speed up the exploratory search is often not feasible as it may result in an unacceptable reduction in recall. In this paper, we present a novel adaptive search algorithm that refines the query based on relevance feedback provided by the user. Additionally, we propose a mechanism that allows the algorithm to self-adapt to new patterns without requiring any user input. As the search progresses, the algorithm constructs a library of time series patterns that are used to accurately find objects of the target class. Experimental validation of the algorithm on real-world manufacturing data shows, that the recall for the retrieval of fault patterns is considerably higher than that of other state-of-the-art adaptive search algorithms. Additionally, its application to publicly available benchmark data sets shows, that these results are transferable to other domains.
更多查看译文
关键词
Time series similarity search,Adaptive similarity search,Exploratory search,Relevance feedback,Pseudo relevance feedback
AI 理解论文
溯源树
样例
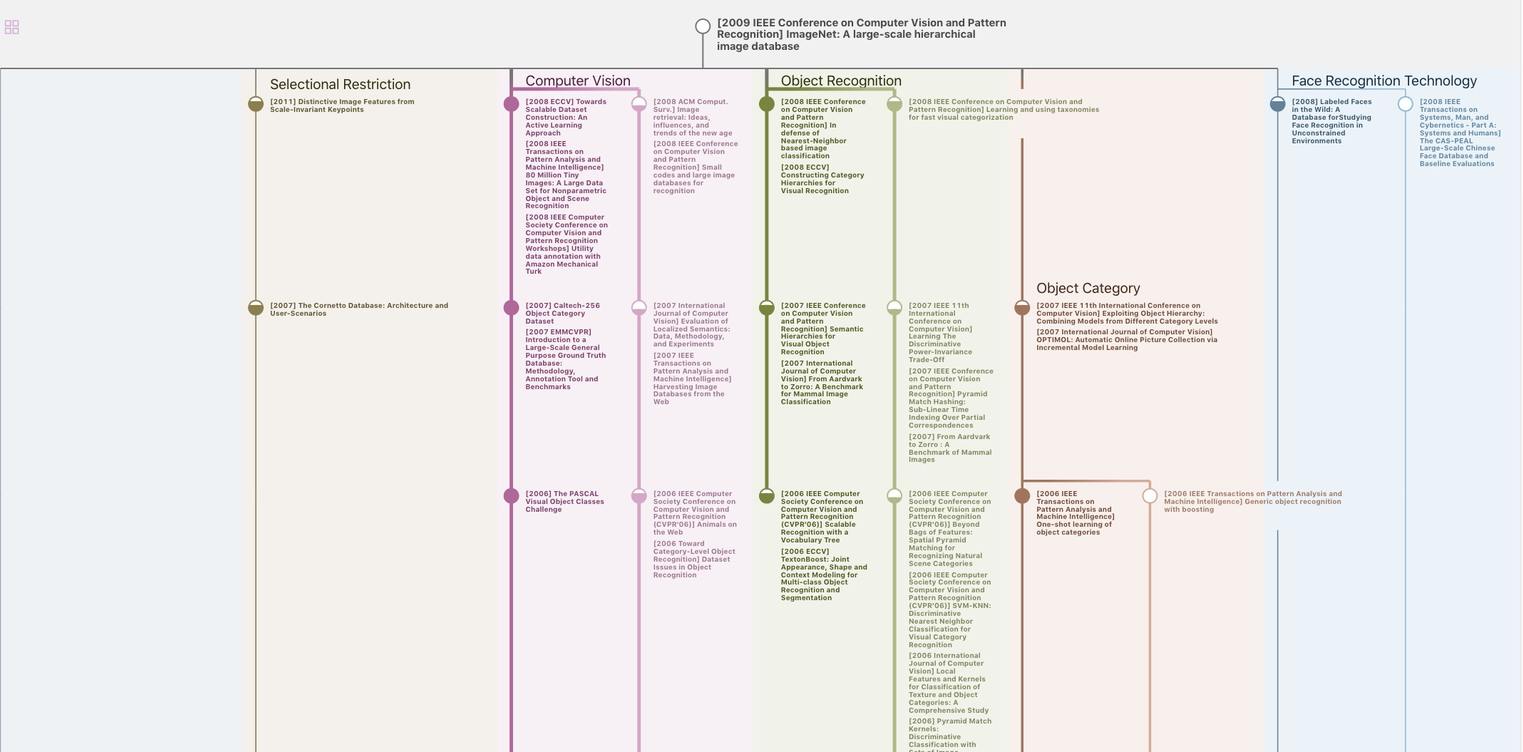
生成溯源树,研究论文发展脉络
Chat Paper
正在生成论文摘要