A Semiparametric Multiple Imputation Approach to Fully Synthetic Data for Complex Surveys
JOURNAL OF SURVEY STATISTICS AND METHODOLOGY(2022)
摘要
Data synthesis is an effective statistical approach for reducing data disclosure risk. Generating fully synthetic data might minimize such risk, but its modeling and application can be difficult for data from large, complex surveys. This article extended the two-stage imputation to simultaneously impute item missing values and generate fully synthetic data. A new combining rule for making inferences using data generated in this manner was developed. Two semiparametric missing data imputation models were adapted to generate fully synthetic data for skewed continuous variable and sparse binary variable, respectively. The proposed approach was evaluated using simulated data and real longitudinal data from the Health and Retirement Study. The proposed approach was also compared with two existing synthesis approaches: (1) parametric regressions models as implemented in IVEware; and (2) nonparametric Classification and Regression Trees as implemented in synthpop package for R using real data. The results show that high data utility is maintained for a wide variety of descriptive and model-based statistics using the proposed strategy. The proposed strategy also performs better than existing methods for sophisticated analyses such as factor analysis.
更多查看译文
关键词
Alternating conditional expectation,Combining rule,Complex survey,Ridge-penalized logistic regression,Statistical confidentiality limitation
AI 理解论文
溯源树
样例
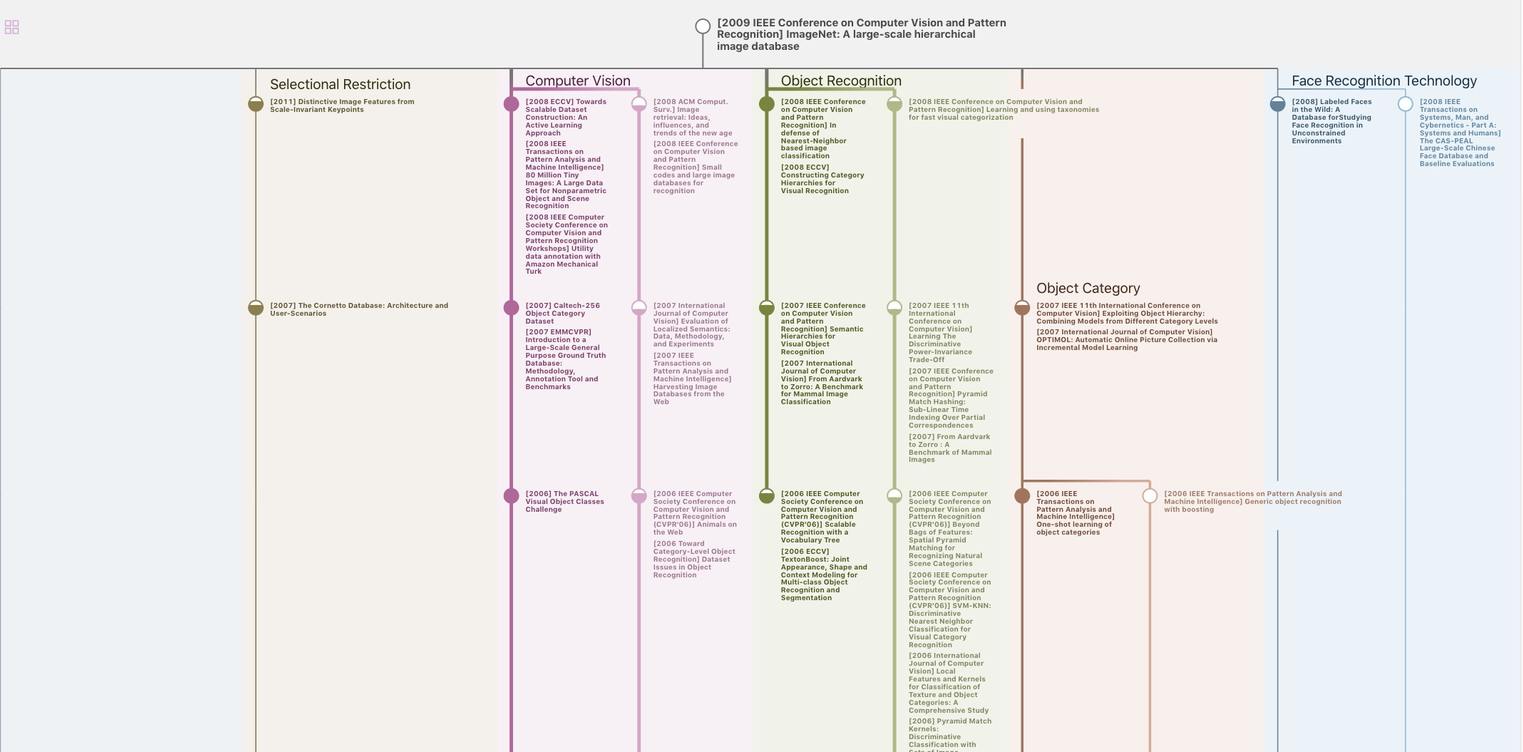
生成溯源树,研究论文发展脉络
Chat Paper
正在生成论文摘要