Reliability-based design in spatially variable soils using deep learning: an illustration using shallow foundation
GEORISK-ASSESSMENT AND MANAGEMENT OF RISK FOR ENGINEERED SYSTEMS AND GEOHAZARDS(2022)
Abstract
This paper presents the reliability-based design of a strip footing against bearing capacity failure in spatially variable soils using a deep learning approach. In this method, Convolutional Neural Networks (CNNs) is modified to construct, in the presence of soil spatial variabilities and uncertain loads, a single global response surface to emulate the behaviour of the geotechnical system over the entire design space. To improve the efficiency and accuracy of the deep learning approach, an adaptive training strategy is adopted. With sufficient training samples, the CNN-based global response surface can be used in lieu of the time-demanding finite-element model for efficient reliability-based design of the geotechnical system. The integration of the deep learning approach into the conventional double-loop framework is also demonstrated. Some key limitations of the double-loop framework are addressed using the deep learning approach. The results highlight that (i) the CNN-based global response surface successfully captures the behaviour of the strip footing over the entire design space, (ii) it can be seamlessly integrated into the conventional double-loop framework, (iii) it can effectively accommodate changes in target failure probability without excessive computational costs, and (iv) it successfully achieves a significant reduction in computational costs compared to other techniques.
MoreTranslated text
Key words
Spatial variability,reliability-based design,convolutional neural networks,geotechnical engineering,finite element analysis
AI Read Science
Must-Reading Tree
Example
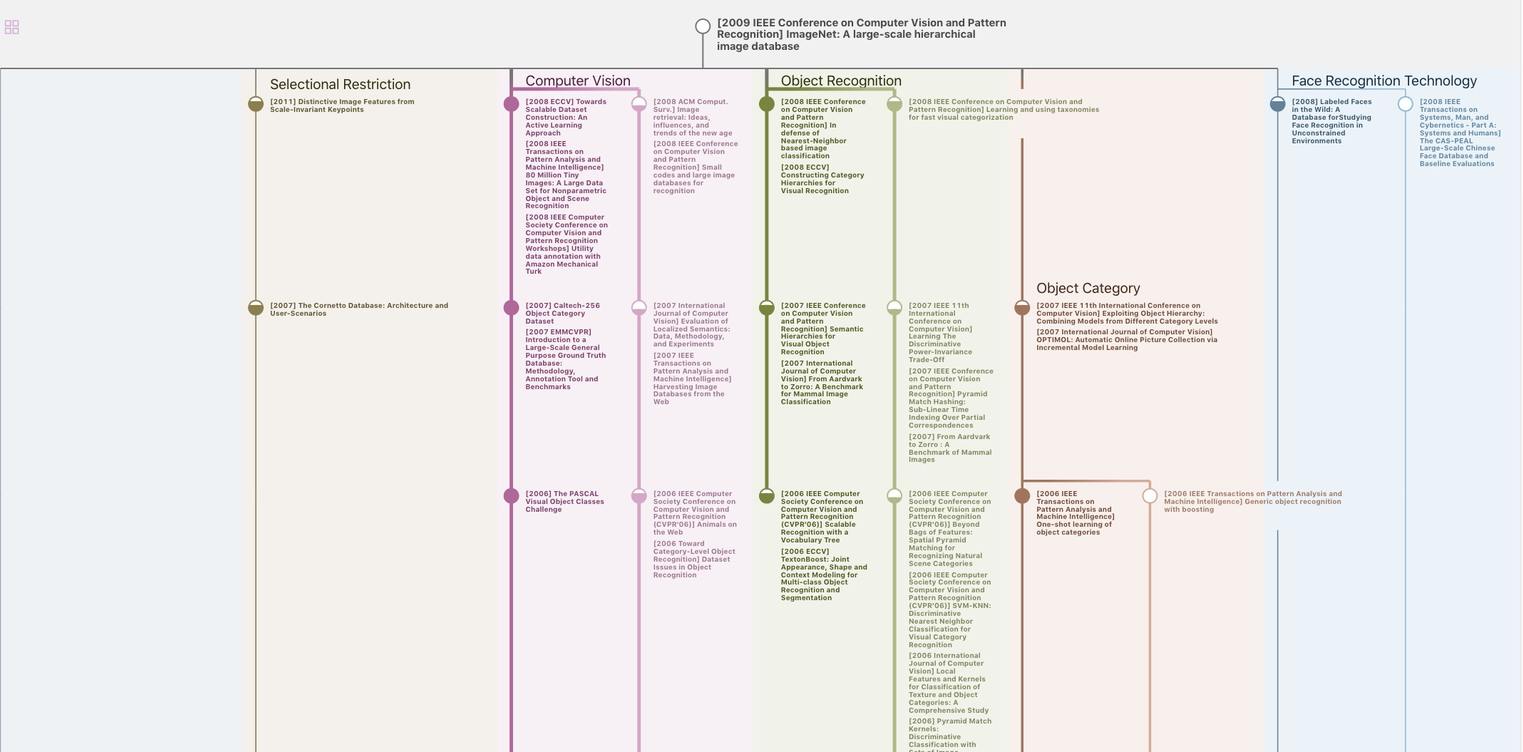
Generate MRT to find the research sequence of this paper
Chat Paper
Summary is being generated by the instructions you defined