Learning Robust Shape-Indexed Features for Facial Landmark Detection
APPLIED SCIENCES-BASEL(2022)
摘要
In facial landmark detection, extracting shape-indexed features is widely applied in existing methods to impose shape constraint over landmarks. Commonly, these methods crop shape-indexed patches surrounding landmarks of a given initial shape. All landmarks are then detected jointly based on these patches, with shape constraint naturally embedded in the regressor. However, there are still two remaining challenges that cause the degradation of these methods. First, the initial shape may seriously deviate from the ground truth when presented with a large pose, resulting in considerable noise in the shape-indexed features. Second, extracting local patch features is vulnerable to occlusions due to missing facial context information under severe occlusion. To address the issues above, this paper proposes a facial landmark detection algorithm named Sparse-To-Dense Network (STDN). First, STDN employs a lightweight network to detect sparse facial landmarks and forms a reinitialized shape, which can efficiently improve the quality of cropped patches when presented with large poses. Then, a group-relational module is used to exploit the inherent geometric relations of the face, which further enhances the shape constraint against occlusion. Our method achieves 4.64% mean error with 1.97% failure rate on COFW68 dataset, 3.48% mean error with 0.43% failure rate on 300 W dataset and 7.12% mean error with 11.61% failure rate on Masked 300 W dataset. The results demonstrate that STDN achieves outstanding performance in comparison to state-of-the-art methods, especially on occlusion datasets.
更多查看译文
关键词
facial landmark detection, shape-indexed feature, face shape constraint, biometrics
AI 理解论文
溯源树
样例
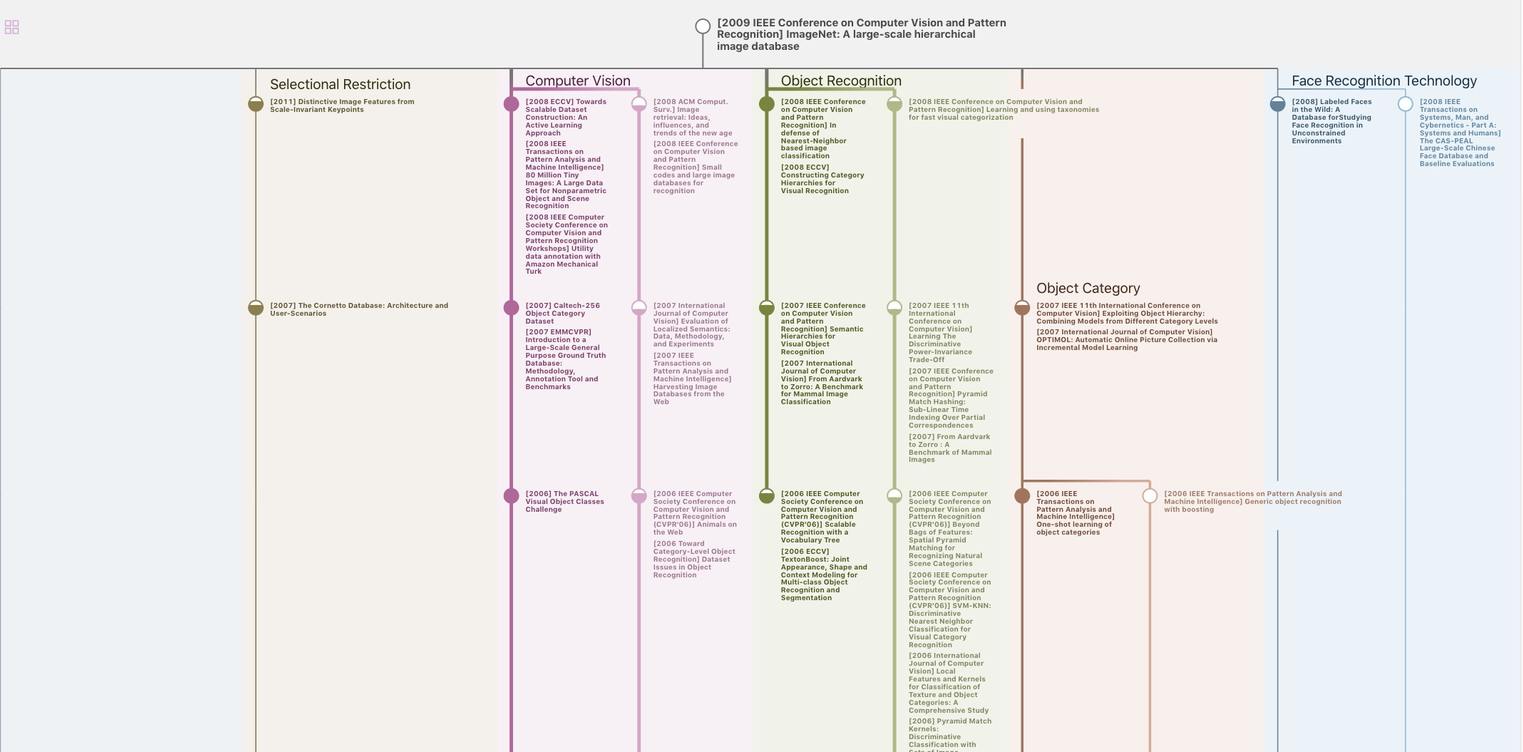
生成溯源树,研究论文发展脉络
Chat Paper
正在生成论文摘要